Optimize data center networking for AI workloads
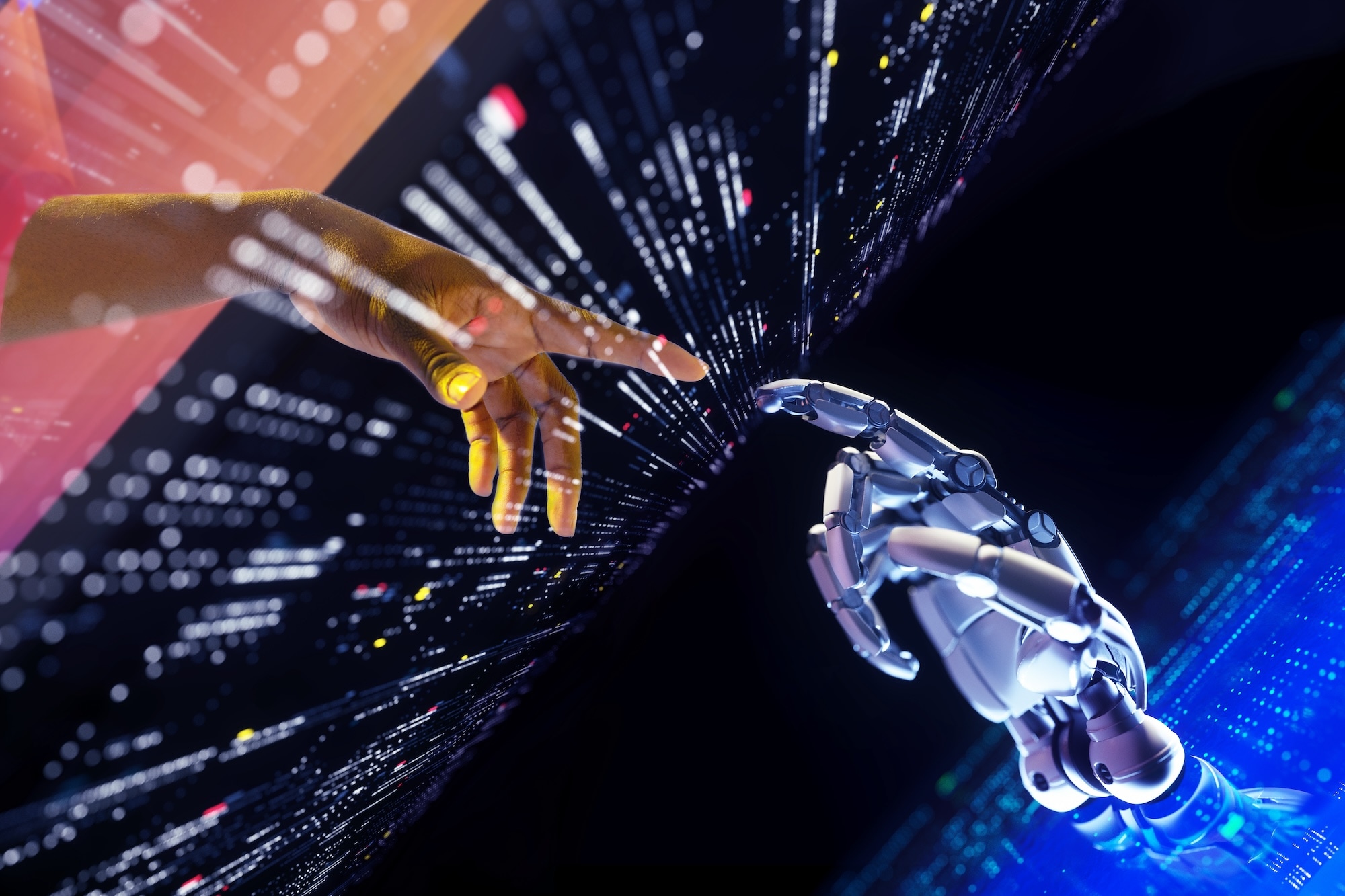
Artificial intelligence (AI) isn’t just dominating technology headlines. It’s changing the way organizations work. Across every industry, companies are turning to AI to streamline their operations, unlock new revenue and deliver more compelling customer experiences.
But organizations have to overcome some challenges to realize the benefits of AI. One of the biggest is to ensure that their data centers can handle AI’s massive data flows and compute requirements. They also need to adapt to its unique traffic patterns and use of Remote Direct Memory Access (RDMA). In other words, organizations need to optimize data center networking for AI workloads.
AI workloads bring new challenges
Most organizations don’t have a clear view of what role AI will play in their current and future digital transformation initiatives. They’re forging ahead because they recognize that AI can help them assess and interpret data, make smarter decisions and solve tough problems. So it’s no surprise to see the rapid adoption of AI use cases such as natural language processing (NLP), outcome prediction, personalization and visual analysis.
These use cases have diverse applications, but they introduce special challenges and unique networking challenges. For example, they generate workloads that are more compute-intensive than those associated with traditional applications. They use massive volumes of data from many different sources. And they require fast, parallel processing.
From training to inference: Understanding AI workloads
The AI world revolves around models. All AI workloads are classified into one of two main categories—AI training or AI inference—based on the tasks they perform with a given model.
AI training focuses on preparing a model for a specific use case. It includes data collection, model selection, model training, model evaluation, model deployment and model monitoring. The workloads for AI training involve huge data flows and heavy compute across large clusters of graphics processing units (GPUs). They need high bandwidth and are highly sensitive to packet loss.
AI inference focuses on packaging the trained model and serving it to users. Inference analyzes and processes input from users and feeds it into the model, which then delivers relevant output. The data flows are much smaller than those for AI training, but the output may come from many different GPUs working in parallel, so low latency is a must.
Evolving back-end and front-end networks for AI workloads
Organizations need optimized data center networking to meet the special requirements of AI workloads. These networks must provide seamless, ultra-reliable connectivity to every part of the AI infrastructure. They may require specialized software and large-scale storage to ensure fast job completion times (JCTs).
For AI training workloads, the ideal solution is a lossless back-end network that combines high capacity and speed with low latency. For AI inference workloads, the best approach is a front-end network that can deliver fast response times to users from the network edge.
Organizations can deploy back-end and front-end networks separately or converge them to address specific customer, cost and power usage requirements. They can also distribute front-end and back-end infrastructure across multiple locations to support promising use cases such as GPU as a service (GPUaaS), offered by cloud providers, and real-time training and inference deployed by enterprises at the network edge. These distributed deployments require exceptionally reliable, high-performance data center interconnectivity solutions.
Why Ethernet is right for AI workloads
InfiniBand has been a popular technology choice for AI networking because it supports RDMA and enables reliable, high-capacity interconnects. Organizations are now turning to Ethernet technologies to build back-end networks for AI workloads. Ethernet already dominates front-end network designs.
The Ultra Ethernet Consortium (UEC) is making the switch easier with enhancements that cement Ethernet’s status as the ideal technology for AI network infrastructures.
For example, UEC members, including Nokia, are developing an open, interoperable, high-performance, full-communications-stack architecture that can meet the networking demands of AI and HPC workloads at scale. It aims to optimize these workloads by modernizing RDMA operation over Ethernet. The Ultra Ethernet Transport (UET) protocol will achieve this goal with innovations that enable higher network utilization and lower “tail latency” to reduce JCTs.
Essential building blocks for AI-ready data center fabrics
What does it take to embrace Ethernet fabrics and evolve back-end and front-end networks to handle the rigors of AI training and inference workloads? Read on to discover key building blocks for AI-ready data center networking.
Flexible hardware
Organizations need flexible hardware options in multiple form factors to implement high-performance leaf–spine fabrics. These Ethernet data center switching platforms should make it easy to build lossless back-end networks that can deliver high capacity and latency of AI training workloads. They should also support front-end designs that can interconnect AI inference and non-AI compute workloads and shared storage with low latency.
A modern, open network operating system
Data center switching platforms need a network operating system (NOS) that’s ready for current and future needs. An ideal NOS will ensure reliability and quality, support openness, automation at scale and facilitate customization with modern interfaces. It should provide capabilities that enable organizations to support lossless Ethernet networking and meet the scalability and performance demands of any AI workload.
Flexible automation tools
Organizations need to automate their data center fabric operations to handle bigger, more challenging AI workloads. The best solutions will make it simple to support intent-based automation and extend it to every phase of the fabric lifecycle—fabric design, deployment and day-to-day operations.
Data center interconnectivity
The deployment of distributed AI infrastructures will allow organizations to support AI training and inference workloads at the network edge, close to users. To get full value from these applications, they need solutions that can support reliable, high-performance interconnectivity across AI network domains.
Find out more
Read our application note and visit our website to learn more about how the Nokia Data Center Fabric solution provides all the building blocks you need to optimize networking for current and future AI workloads.