Analytics as a Service: Transforming the telecom industry in the XaaS era
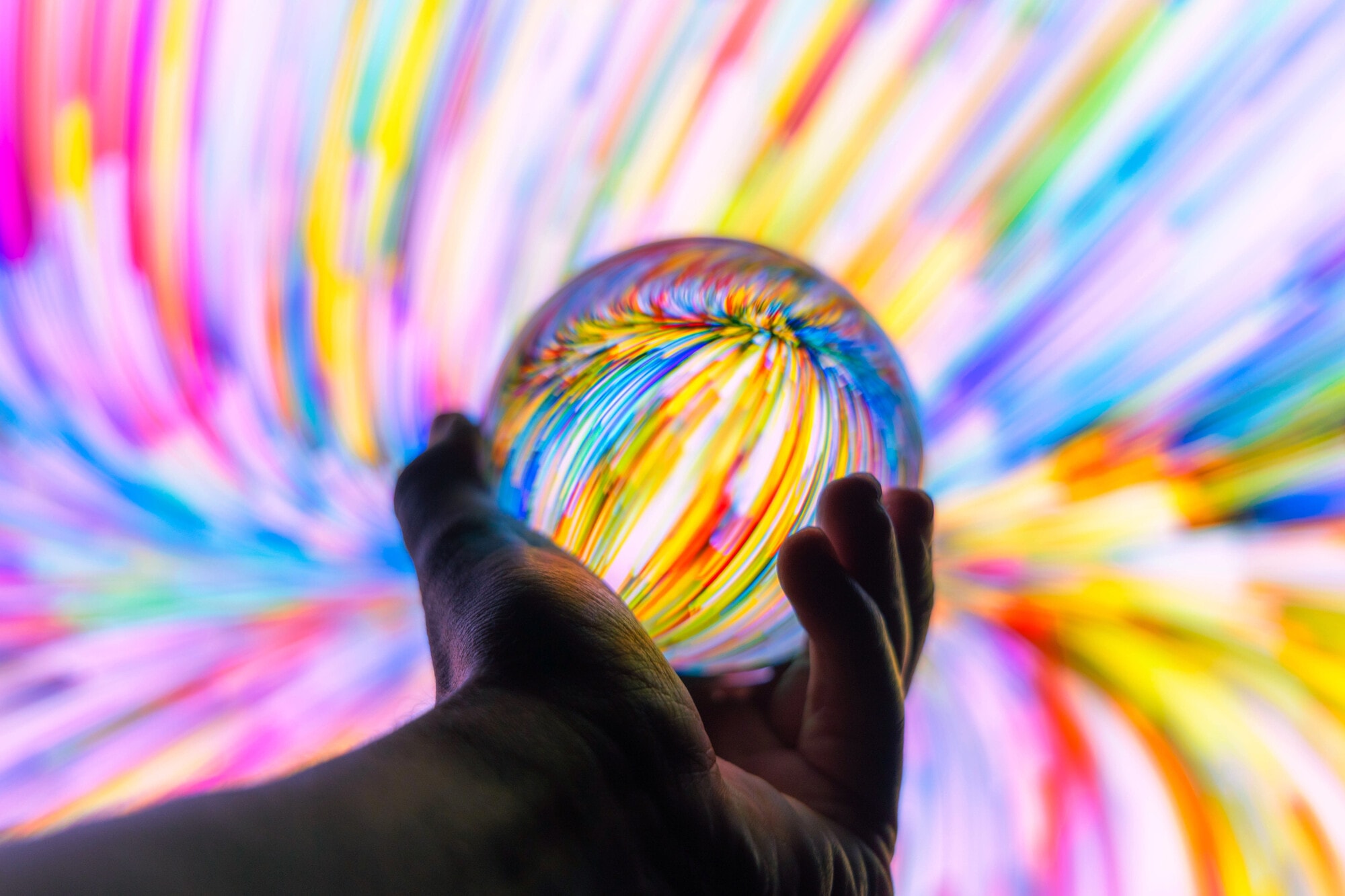
The telecommunications industry is undergoing a significant shift toward “everything as a service” (XaaS). Software as a service (SaaS) is a key driver of this transformation, enabling communications service providers (CSPs) to differentiate the types of applications and use cases consumed as SaaS, including infrastructure as a service (IaaS), platform as a service (PaaS) and other nested SaaS services.
XaaS represents a fundamental shift in how technology and business services are delivered and consumed. It is characterized by its flexibility, scalability and focus on customer outcomes. While presenting challenges for CSPs, XaaS offers significant opportunities for innovation, growth and competitive advantage.
XaaS encompasses a vast and growing array of services delivered over the internet, extending beyond the initial core offerings of SaaS, IaaS and PaaS. Other XaaS services include, but are not limited to, desktop as a service (DaaS), authentication as a service (AUaaS), unified communications as a service (UCaaS), functions as a service (FaaS), storage as a service (STaaS), containers as a service (CaaS), network as a service (NaaS), disaster recovery as a service (DRaas) and security as a service (SECaaS).
Several technological advancements enable and drive the growth of XaaS, including cloud computing, microservices architecture, application programming interfaces (APIs), automation and artificial Intelligence (AI).
Analytics as a Service (Analytics aaS), in this context, is emerging as a prime example and a natural fit within the broader XaaS trend. Analytics aaS provides analytics software and capabilities through cloud-based technologies, enabling users to track key metrics and gain insights from their data.
This research note examines how CSPs are leveraging Analytics aaS from an infrastructure, placing it within the XaaS landscape and exploring the benefits, obstacles and key decision-makers involved in its adoption.
Understanding analytics aaS within the XaaS landscape
Analytics aaS aligns with the core principles of XaaS, making it a compelling solution for CSPs navigating the complexities of digital transformation. Analytics aaS exemplifies the core principles of XaaS as follows:
-
Meeting the Demand for Specialized Services: XaaS is characterized by a growing need for specialized services delivered on-demand.
-
Analytics aaS addresses this by providing access to advanced analytics tools and expertise without significant upfront investments or the need for specialized in-house teams. This accessibility is particularly crucial for CSPs seeking to leverage data-driven insights for network optimization, customer experience enhancement and new revenue generation.
-
-
Enabling Agility and Scalability: Analytics aaS embodies the XaaS principles of agility and scalability.
-
CSPs can rapidly deploy analytics solutions, scale their usage based on demand and adapt to evolving business requirements. This flexibility is essential in the dynamic telecom landscape, where providers need to respond quickly to changing market conditions and technological advancements.
-
-
Lowering the Barrier to Entry: XaaS aims to democratize access to technologies and services previously available only to large enterprises and their customers.
-
Analytics aaS contributes to this democratization by making advanced analytics accessible to CSPs of all sizes. This accessibility levels the playing field, allowing smaller providers to compete more effectively by leveraging data-driven insights.
-
As a result, Analytics aaS contributes to closing the gap between those who have access to and can effectively use digital technologies and those who cannot by lowering the cost of technology, reducing complexity, minimizing exclusivity and accelerating innovation.
-
-
Catalyzing the Rollout of Autonomous Networks: XaaS facilitates the development and deployment of AI/ML models in several ways.
-
Analytics aaS offers pre-integrated, domain-specific data models and AI/ML algorithms, making it easier for CSPs to incorporate these capabilities into their networks. This reduces the need for extensive in-house development and speeds up the adoption of AI-driven automation for autonomous networks.
-
Enhanced data management: Analytics aaS providers can handle the complexities of data engineering, including data integration, automation and quality assurance, which are crucial for training effective AI/ML models. This ensures that AI/ML algorithms have access to reliable and accurate data, improving their effectiveness in managing autonomous networks.
-
Pooling of data for improved accuracy: Analytics aaS enables the aggregation of anonymized data from multiple customers, creating larger and more diverse training datasets for AI/ML models. This results in more robust and accurate models, leading to better decision-making and automation in autonomous networks.
-
-
Leveraging Cloud Infrastructure: XaaS heavily relies on cloud computing for on-demand access and scalability.
-
As Analytics aaS solutions are typically built on cloud platforms, they further contribute to cloud technology adoption in the telecom industry. This reliance on cloud infrastructure provides the flexibility and scalability needed to manage the increasing volume of data generated by modern telecom networks, particularly with the rise of 5G and IoT.
-
-
Advancing Environmental, Social and Governance (ESG) Goals: XaaS contributes to broader sustainability goals and responsible business practices. Analytics aaS enables CSPs to address multiple ESG goals.
-
Make more informed decisions to improve their ESG performance by analyzing data related to energy consumption, social impact and other relevant factors. For example, CSPs can achieve energy savings in the Radio Access Network (RAN).
-
Realize sustainable resource utilization by reducing the need for on-premise hardware and streamlining operations.
-
Experiment with new technologies and services quickly, which facilitates the development and deployment of solutions that address ESG concerns.
-
Improve transparency and accountability by implementing robust security measures and compliance with regulations like GDPR, which are crucial for good governance and ethical business practices.
-
Analytics aaS is a catalyst for AI/ML adoption
Analytics aaS can alleviate many of the challenges associated with AI/ML adoption, including:
-
Data Engineering Hurdles: Preparing and managing data for AI/ML can be complex.
-
Analytics aaS solutions include pre-built integrations and data pipelines based on industry best practices that streamline this process, saving time and ensuring data quality. This simplification allows CSPs to focus on extracting insights from their data rather than grappling with the complexities of data engineering.
-
-
Model Engineering Hurdles: Building accurate and effective AI/ML models requires specialized expertise.
-
Analytics aaS offerings include pre-built models tailored for specific telecom use cases, such as network optimization, customer churn prediction and security threat detection. The access to pre-built models accelerates the deployment of AI/ML solutions and reduces the need for extensive in-house or external consulting expertise.
-
-
Infrastructure Hurdles: AI/ML workloads can be computationally intensive and require significant infrastructure investment.
-
Analytics aaS leverages the scalability and cost-effectiveness of cloud infrastructure to address this challenge. By offloading infrastructure management to the Analytics aaS provider, CSPs can reduce their capital expenditures and avoid the complexities of managing their own AI/ML infrastructure.
-
Analytics aaS provides multiple operational, business and financial benefits for CSPs
CSPs can realize multiple benefits by adopting Analytics aaS, including faster time to value, reduced complexity, cost savings, improved operational efficiency, enhanced security and increased scalability and agility.
-
Faster Time to Value: Analytics aaS solutions, with their pre-built integrations and automation features, enable CSPs to deploy analytics capabilities faster compared to building them in-house or using commercial on-premises solutions. This rapid deployment translates into a faster realization of benefits and a quicker return on investment.
-
Analytics aaS allows large CSPs, who have highly customized applications, to reduce maintenance and operating expenses and replace these applications as required.
-
-
Reduced Complexity: Analytics aaS eliminates the need to rely on extensive expertise from internal experts or external consultants in data engineering, AI/ML and MLOps to manage the technology lifecycle, software updates or security patching. This reduced complexity allows CSPs to focus on their core competencies rather than investing heavily in non-mission critical solutions.
-
CSPs can leverage the specialized and domain-specific knowledge of the Analytics aaS provider and their pre-built models, simplifying the implementation and management of AI/ML solutions that ensure the infrastructure’s health, performance and AI/ML quality.
-
CSPs can integrate advanced AI/ML capabilities with Analytics aaS, simplifying integration complexities through standardized interfaces and APIs. This allows for cross-domain use case development for both internal innovation and external 5G monetization opportunities.
-
Analytics aaS providers reduce the workload of CSPs’ IT staff by addressing governance and ethical AI to ensure privacy, security and transparency.
-
-
Cost Savings: Analytics aaS offers flexible pricing models, including subscription-based, consumption-based and outcome/value-based options. This shift from capital expenditures (capex) to operational expenses (opex) can be particularly beneficial for CSPs looking to optimize their costs and improve their financial flexibility.
-
Opex-based models provide CSPs with predictability in operating expenses and remove the necessity for significant upfront investments in infrastructure and specialized personnel.
-
They also allow for on-demand access to test and sandbox environments for model retraining and new data sources, ensuring agility and cost-effectiveness.
-
-
Improved Accuracy: Analytics aaS providers give access to vast, pooled data from their entire customer base, leading to larger and more diverse training datasets than a single CSP could create. This data, along with continuous user feedback and corrections, improves the accuracy of AI/ML models. CSPs can use this data via decentralized, federated learning, conforming to privacy considerations and ethical AI stipulations of local regulatory bodies.
Key use cases for analytics aaS
CSPs and Analytics aaS providers have identified multiple use cases driving Analytics aaS adoption for network operations:
-
Augmenting Network Operations: Analytics aaS provides the agility for CSPs to optimize their networks and operations, supporting:
-
Technology trials, innovation labs as well as development and testing environments: Analytics aaS offers flexible and readily available environments for CSPs to experiment with new technologies and service offerings.
-
Disaster recovery: In case of outages or disasters, Analytics aaS can act as a reliable backup for critical analytics functions, ensuring business continuity.
-
-
Optimizing Existing Services: Analytics aaS enables CSPs to analyze network data and enhance existing services, leading to:
-
Network slicing optimization: Analytics aaS helps tailor network slices to specific use cases and optimize their performance for improved customer experience.
-
Resource optimization: By analyzing network data, Analytics aaS helps CSPs allocate resources more efficiently and optimize their utilization.
-
AIOps Assurance: Leveraging AI-driven analytics, Analytics aaS enhances operational assurance, enabling proactive issue identification and resolution.
-
Predictive Identification of Anomalies: By providing pre-built data engineering, access to extensive data sets, continuous improvement and simplified operations, Analytics aaS allows CSPs to efficiently leverage the power of AI/ML to identify anomalies and proactively address potential issues.
-
Business-driven prioritization: Analytics aaS supports business-driven prioritization by aligning analytics efforts with strategic goals, prioritizing projects based on their speed of delivering value, adapting to changing market dynamics and business needs, allocating resources efficiently based on cost-effectiveness and making data-driven decisions that support business objectives.
-
-
Enabling New Revenue Streams and Business Models: Analytics aaS plays a crucial role in creating new business opportunities and revenue streams:
-
Network as code at the edge: Analytics aaS facilitates the development and deployment of applications based on the “network as code” principle, particularly at the edge, where real-time data processing is vital.
-
MVNO/MVNE as a Service: Analytics aaS empowers mobile virtual network operators and empowers mobile virtual network enablers to offer analytics-driven services to their customers, expanding their service portfolio.
-
Pop-up networks on-demand: Analytics aaS supports the dynamic provisioning and management of temporary networks for events or specific use cases, opening up new markets and revenue opportunities.
-
-
Enhancing Security and Threat Management
-
Automated Security Assurance: With the increasing complexity of 5G and edge computing, Analytics aaS is essential for providing real-time security analytics, threat detection and automated security assurance.
-
Obstacles to analytics aaS adoption and potential solutions
Despite the numerous advantages, certain obstacles might hinder the widespread adoption of Analytics aaS in the telecom sector. These include financial concerns, customization concerns, security concerns and the challenges posed by complex integration ecosystems.
-
Financial Concerns: Transitioning from traditional capex models to the opex model inherent in Analytics aaS can be challenging CSPs that historically preferred on-premises software or third-party hosted solutions.
Analytics aaS providers must offer flexible payment options (subscription-, consumption- and outcome/value-based), potentially including capex-like models, to ease the transition for CSPs accustomed to conventional procurement processes. -
Customization Concerns: Large CSPs have heavily customized solutions tailored around specific use cases that meet their specific needs and requirements. These solutions are difficult to operate and expensive to maintain, further hindering the transition to a standardized SaaS model.
CSPs can overcome this obstacle by starting with SaaS applications that are less critical and require less customization, such as analytics, before moving to more complex systems. While Analytics aaS solutions are standardized, they offer configuration options that allow for some level of tailoring to specific needs. Analytics aaS vendors need to prioritize customer success, proactively engaging with customers to ensure they are deriving value from the standardized solution. -
Security Concerns: Entrusting sensitive customer data to an external Analytics aaS provider requires a high level of trust and confidence in the provider’s security measures and compliance with data privacy regulations.
Choosing Analytics aaS providers with strong security certifications (like SOC2, GSMA SAS and ISO 27001) and demonstrably transparent data management practices is crucial to building trust and addressing these security concerns. CSPs must conduct thorough due diligence to ensure the Analytics aaS provider has robust security protocols, data encryption mechanisms and compliance with relevant data protection laws. -
Complex Integration Ecosystems: Integrating Analytics aaS with existing legacy systems and emerging ecosystems in the public and private cloud can pose significant technical challenges and necessitate a considerable amount of effort. The use of non-standard APIs remains common practice in the CSP ecosystem, increasing complexity and reducing automation opportunities.
Collaborating with experienced Analytics aaS providers and system integrators can help navigate these technical complexities and ensure a smoother integration process. Starting with less sensitive use cases for Analytics aaS, such as network optimization, before tackling more sensitive areas like customer data analysis, can be a practical approach to mitigate integration risks.
Key decision-makers in analytics aaS adoption
The responsibility for purchasing Analytics aaS solutions is spread across different CSP departments.
-
Data and AI Officers: These roles are key stakeholders and decision makers when it comes to selecting and promoting Analytics aaS within CSP organizations. They are responsible for ensuring a smooth transition to SaaS, safeguarding data security and compliance, maximizing the value derived from analytics and fostering AI innovation within the organization. Effective management of these areas enables the data and AI officer to help the organization leverage Analytics aaS as a strategic tool for achieving business goals and remaining competitive.
-
CxOs: As executive sponsors, CxOs (CEO, CFO, CIO and others) play a crucial role in driving the overall SaaS transformation, including Analytics aaS adoption. Their understanding of the strategic benefits of Analytics aaS, such as improved customer experience, cost optimization and new revenue opportunities, is vital for securing organizational buy-in and driving the implementation process.
-
Procurement and CFO: These roles focus on the financial viability of Analytics aaS, analyzing the total cost of ownership (TCO) and the potential for converting capex to opex. Their approval and support are essential for navigating the shift from traditional capex models to the subscription-based nature of Analytics aaS.
-
Legal: Legal teams are involved in creating or extending frame agreements to accommodate the SaaS model and address any new terms and conditions associated with Analytics aaS. They ensure compliance with data privacy regulations, intellectual property rights and service level agreements (SLAs).
-
Operations: Operations teams assess the SLAs of Analytics aaS providers to ensure they meet the CSP’s operational standards. They are responsible for ensuring the seamless integration of Analytics aaS solutions into existing workflows and minimizing any disruption to existing services.
-
IT/Network Teams: This team handles the technical integration of Analytics aaS solutions, ensuring seamless connectivity with existing systems and the chosen hyperscaler, such as AWS, Azure and GCP. Their expertise in cloud computing, data management and network infrastructure is critical for successful Analytics aaS deployment and ongoing management.
-
Security: Security teams are responsible for validating the Analytics aaS provider’s security model and data privacy practices, ensuring compliance with company policies and regulatory requirements. They conduct security audits, vulnerability assessments and penetration testing to ensure the confidentiality, integrity and availability of data. Increasingly, security teams are responsible for and involved in reviewing AI governance models, including the need for reassurance about the risks and impact of packaged Analytics aaS AI.
Analytics aaS will play a crucial role in shaping the future of the telecom industry
Analytics aaS is a pivotal component of the broader XaaS movement and is poised to transform the telecom industry. It embodies the key benefits of XaaS – accessibility, affordability, scalability and outcome-orientation – while simultaneously enabling the adoption of transformative technologies like AI and ML. By leveraging Analytics aaS, CSPs can:
-
Gain a competitive edge by unlocking valuable insights from their data.
-
Improve operational efficiency.
-
Enhance customer experiences.
While there are challenges to overcome, the potential rewards of Analytics aaS adoption are significant. As the telecom industry continues to evolve in the XaaS era, Analytics aaS will play an increasingly crucial role in shaping the future of the industry. By addressing the concerns of key decision-makers, fostering a culture of data-driven decision-making and partnering with experienced Analytics aaS providers, CSPs can fully harness the transformative power of Analytics aaS and thrive in the increasingly data-centric digital landscape.