Stopping DDoS is easy - stopping only DDoS is hard
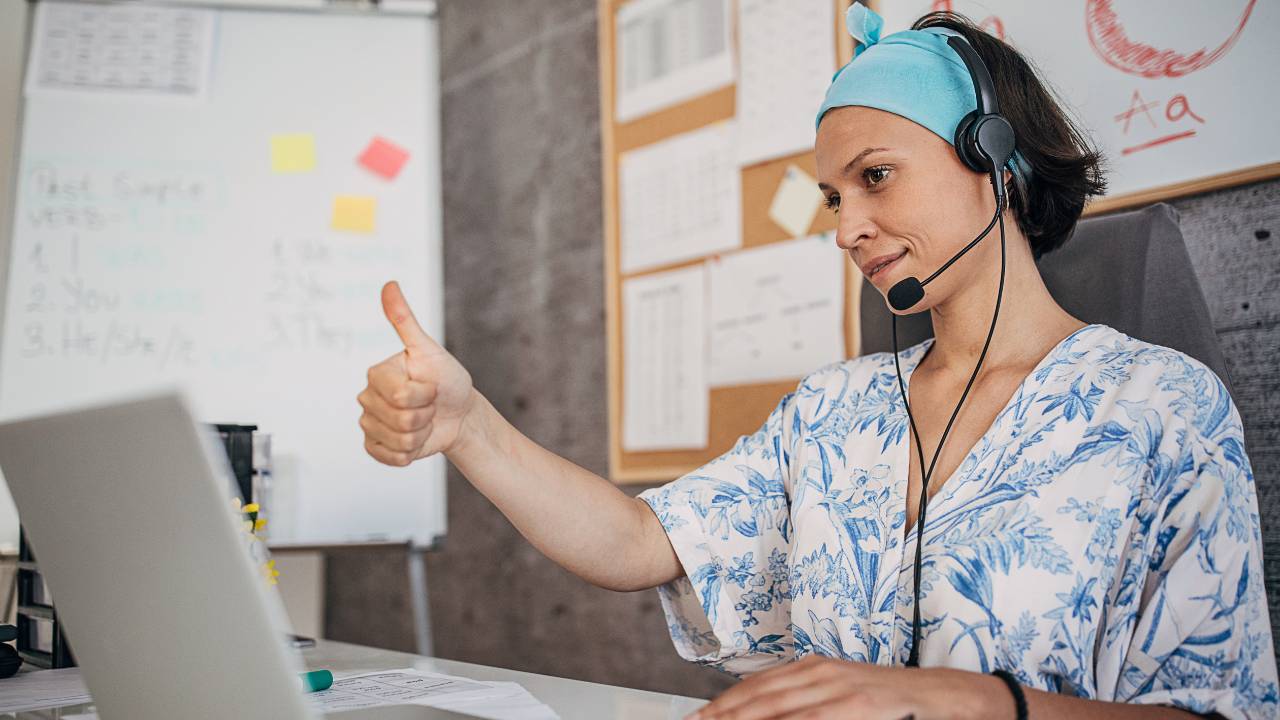
DDoS attacks can affect organizations of any size—even very large ones with very large security operations teams on the payroll, as revealed by the Microsoft 365 service outage a few weeks ago.
In this case, however, the problem was not so much the DDoS attack traffic itself—reportedly a TCP SYN flood, the fourth most common attack vector in our customers’ networks in 2024, according to the Nokia Deepfield Emergency Response Team (ERT). Instead, it was the response to the attack that created more issues than the attack itself: The DDoS mitigation did stop the malicious traffic, but it also stopped a significant share of the legitimate customer traffic.
As a result, the mitigation process removed a lot of “good” traffic along with the offending DDoS traffic, affecting services and users in the process.
Let me make it clear that the goal of this post is not to pile on this outage––it can happen! #hugops––but instead to see what we all can learn about it.
I briefly mentioned this in an earlier post, but here it is again. In assessing the effectiveness of a specific DDoS protection solution, it is essential to look at how much of the DDoS attack traffic we are (correctly) blocking and, at the same time, measure the impact of the mitigation on the legitimate (or “good”) traffic.
The real effectiveness of mitigation is about getting as close as possible to the desired goal of 0 percent false negatives (i.e., not passing any DDoS traffic as good) and 0 percent false positives (i.e., not blocking good traffic that was identified as DDoS, thereby avoiding the “computer says no” problem at scale).
These are really two edges of the same blade: It is easy to get 0 percent for either one of these metrics but much harder to get them both right (or as close to zero as possible).
For the past twenty years, acceptable rates for false positives and false negatives for organizations using traditional approaches and legacy anti-DDoS solutions have been anywhere from 5–10 percent. With growing DDoS traffic volumes and the increasing frequency and sophistication of DDoS attacks, this would translate to removing terabytes of legitimate customer traffic any time a large-scale DDoS attack is mitigated.
So, how do we bring both these metrics (false negatives and false positives) down with our Deepfield Defender-based anti-DDoS solution? It’s AI to the rescue! Or, to be more specific, it’s machine learning. For the past several years, we have been leveraging supervised learning techniques to continuously measure the effectiveness of our Deepfield Secure Genome® models (used by our Deepfield Defender customers) against one of the largest collections of real-world DDoS attack samples anywhere, contributed by collaborating customers through the Global DDoS Threat Alliance (GDTA).
Let’s consider an example where the Nokia Deepfield ERT creates a new mitigation rule to address a novel attack vector. This new rule is added to our beta ruleset, which is then run against a large subset of the GDTA attack samples and, even more importantly, against realistic “peacetime” traffic samples.
The new rule will only be added to live customer deployments if it improves mitigation coverage and doesn’t increase the false positive rate for the legitimate traffic of these peacetime samples.
We confirm this is the case by testing a simulated attack and measuring the percentages of false negatives and positives across these diverse samples. We can then deploy the new models in customers’ networks (Deepfield instances) so that the inference is run locally, with their own traffic, and with the knowledge that it will help to stop DDoS only.
There’s no question that stopping DDoS traffic is essential. But the true measure of the efficiency of DDoS protection lies in mitigating the bad traffic without impacting legitimate users—a challenge we’re committed to mastering.