Why telcos must strategically execute the ‘verticalization’ of Gen AI at scale
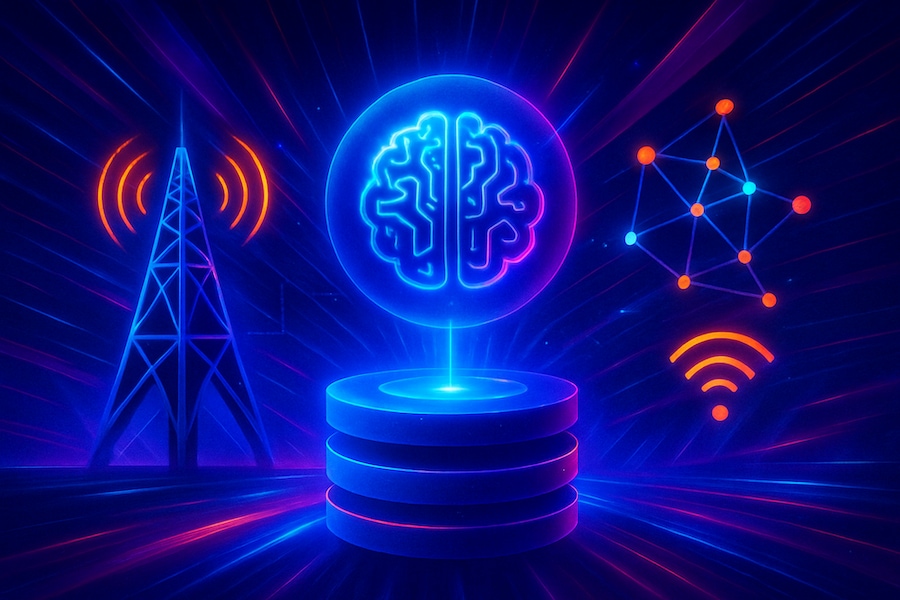
The telecom industry stands at an inflection point. Over the past two decades, every wave of technological evolution — from 3G /4G to 5G/fixed mobile convergence with early cloud-native adoption — has added complexity across all layers of the network.
While these advancements have unlocked new capabilities, they've also introduced complexity and operational overhead that many communication service providers (CSPs) are struggling to manage.
Now, as the world rushes forward in the age of Generative AI, telcos are presented with a once-in-a-generation opportunity to transform how networks are built, managed and operated — making them highly autonomous, agentic and “conversational” at each layer.
To accomplish this, we turn to the concept of Gen AI “verticalization.” In other words, driving impactful business outcomes through a GenAI platform (LLM ops, agentic AI ops) that’s top-to-bottom holistic and infused with domain-specific knowledge.
We’re already witnessing accelerated adoption of Gen AI verticalization tailored to sectors like retail, fintech, biotech and large enterprises.
For telcos, the network vertical is an environment characterized by high velocity, real-time/non-real time data and deep domain knowledge, as well as the need for both on-prem and sovereign cloud support, stringent regulatory governance and cost constraints. These unique attributes make a highly verticalized Gen AI solution a no-brainer.
The need to act is urgent, and the timing couldn't be better.
The automation imperative: simplifying the complex
Today’s telcos face a dual mandate: cut costs while building future-ready, cloud and AI native networks for new-age services. The traditional methods of achieving operational efficiency — process re-engineering, outsourcing and standard automation — are reaching diminishing returns. What telcos need now is a paradigm shift, and Gen AI-based automation offers just that.
With each generational upgrade — across multiple domains of radios, transport and routers, rising 5G standalone core networks and associated slicing operations, and new monetization platforms — we’ve added abstraction layers such as control planes, orchestration layers, virtualized/containerized network functions, hybrid clouds … and the list continues to grow. This has made networks not only difficult to manage but also increasingly expensive to maintain.
Gen AI offers a three-layered platform that transforms structured/unstructured data streams to persona-based agentic AI business outcomes. These layers are 1) accelerated compute infra with model serving, 2) AI ops / LLM ops stack and 3) agentic AI apps. When built specifically for the telco domain at each layer, this platform has strong potential to serve as a highly effective foundational tool for simplification.
With its ability to understand and generate human-like responses, analyze massive volumes of data and even automate decision-making processes, Gen AI is uniquely positioned to drive intelligent automation across all network layers. It can streamline service delivery, optimize troubleshooting, simplify network planning and enhance customer interactions — paving the way for leaner, smarter and more agile networks.
As telcos increasingly recognize this transformative potential, the focus has shifted from pilot projects to widespread adoption and scaling, reflecting a significant change in strategy and investment priorities.
For example, in 2023, 34% of CSPs surveyed by McKinsey & Company were focused on pilots and quick wins. In 2025, however, 64% are focused on scaling their AI/Gen AI adoption in the form of multi-agent systems across the organization.
From a financial standpoint, Appledore predicts AIOps will make up 26% of the $14.8 billion that CSPs will invest by 2028 in automation. Furthermore, the McKinsey Global Institute estimates that Gen AI has the potential to unlock 35 to 70% more value beyond that previously offered by advanced analytics and “traditional” AI.
CSP data and cost sensitivities: Sovereignty and control matter
Of course, data control, sovereignty and cost remain top concerns for telcos, and we have experienced this firsthand in the past three years with the accelerated adoption of hybrid cloud. Because CSPs handle sensitive user data, regulated by strict data protection and national security laws, many large-scale productivity use-cases must remain on-premises.
This makes sovereign cloud and sovereign AI strategies essential, especially to networks and operations layers. Telcos need infrastructure that allows them to own and control their data pipelines end to end, whether hosted in private clouds, edge, regional or central data centers. They must embrace hybrid models where Gen AI is deployed on-prem for mission-critical use cases, and in the cloud for more scalable tasks.
Equally important is avoiding vendor/model lock-in. Whereas closed Gen AI stacks inflate costs and restrict innovation, an open-source-first approach, grounded in flexible architecture and supported by a modular tech stack, enables telcos to build capabilities incrementally and sustainably, on their own terms.
The rise of the industrial, open-source Gen AI Tech stack
The verticalization of Gen AI across enterprise, fintech, biotech and other industries is pushing the need for industrial-grade, open-source Gen AI stacks.
Unlike the early days of Gen AI, dominated by a few “closed” commercial platforms, the current Gen AI landscape is thriving with open innovation. Models like Llama, Mistral and Falcon are maturing rapidly, often outperforming their proprietary counterparts in specific tasks, all while being cost-effective and highly customizable. Open models, sharing training-set data and open weights, are fast becoming the norm; not to mention the emergence of reasoning models for “well thought out” and high-relevancy responses, ushered in by the DeepSeek 3 model in early 2025.
Aiding these efforts is a rich ecosystem of open-source Gen AI tools supporting verticalization:
- Retrieval-augmented generation (RAG) frameworks infuse domain-specific knowledge to enhance engagements that drive meaningful business outcomes. RAG integrates both models’ comprehension abilities and relevant telco knowledge to enable domain-specific, conversational experiences. Employing advanced RAG optimization data science can further enhance the quality of responses.
- Parameter-efficient fine-tuning and LoRA (low-rank adaptation) for customizing foundational models with deep telco-specific data are essential for realizing telco verticalization. Specifically, these models are highly tuned (“adapted”) and optimized to perform telco tasks, with the aim to minimize the human time involved.
- Knowledge vectorization and indexing tools to handle a wide range of telco knowledge corpus. This includes the ability to perform efficient chunking, high-throughput multimodal data processing, metadata forming, data indexing and flexible semantic retrieval. Mastering these vertical-specific knowledge management techniques to drive the velocity of automation is both an art and science.
- Agentic AI workflows and pipelines that seamlessly integrate Gen AI into existing business, operations and IT ecosystems. This includes smart usage of function calling and tool usage in a multi-turn, multi-agent environment. Such advanced design patterns must be accommodated by a disciplined practice of test-driving a development regime that utilizes prompt engineering, explainability, hallucination management and guardrails for robustness. A classic software development and test regiment is simply not sufficient in a Gen AI system.
Telcos will have to increasingly leverage these types of advancements to Gen AI stack implementation to enhance business-specific outcomes and elevate customer experience.
Given the high data velocity (both real-time and non-real time), variety and complexity in telecom networks, the potential impact of Gen AI verticalization in telcos could be even more transformative.
Domain knowledge: the missing link
But here lies a critical success factor: telco domain knowledge, something that’s very close to us at Nokia. General purpose Gen AI models, no matter how powerful, lack the contextual understanding needed to solve nuanced telco problems. Telcos operate in a landscape with unique terminologies, regulatory constraints, operational metrics and service models.
To effectively apply Gen AI, domain knowledge must be deeply integrated into every layer of the stack. Omdia reports that 69% of CSPs are already shifting toward incorporating Gen AI into their critical cybersecurity operations, an act that requires deep knowledge of 5G networks and its management and operational processes.
Fortunately, telcos have two major assets in this regard:
- Data (knowledge base): Massive volumes of network, operational, customer and performance structured and unstructured data span years. Unique to telcos are the three layers of networks, management & operations, and Product/IT; each one has its challenges when it comes to data governance.
- Telco domain experts: Seasoned professionals with decades of experience across network planning, operations, network and customer care, and operations.
These resources, when brought together with Gen AI capabilities, can create a virtuous cycle.
For instance, models trained on historical network faults can not only automate L1 to L4 troubleshooting but also predict and prevent future outages. Similarly, customer support systems enriched with domain-trained Gen AI can resolve issues faster and with greater personalization. All of this opens a strategic monetization pathway: telcos can productize their datasets and domain-trained models for use in adjacent sectors like smart cities, automotive and industrial IoT.
Building vertical Gen AI platform for telcos
Verticalization isn’t a plug-and-play affair. To succeed, telcos must build deep in-house software competencies around Gen AI practice. Finding a key strategic partner that infuses both domain expertise and brings unique Gen AI skillset is key.
This means going beyond just hiring AI scientists — it requires assembling multidisciplinary teams that blend:
- LLM ops experts, consisting of both ML system and data scientists familiar with lifecycle management, Gen AI best practices, and state-of-the-art toolkits and practices. There are multiple layers of open-source and third-party toolkits, from data management to model farm management to, eventually, the agentic AI layer. Each needs its own special focus.
- Telco domain experts who can bridge the gap between Gen AI tech stack outputs with effective business operational success. These are experts who do provisioning, planning, deployment, services & care operations and have a global view on challenges across telco operators.
- AI engineers and data scientists with experience in scalable, distributed platform systems and managing/maintaining Gen AI platform lifecycles. It’s key to have data science engineers, at all levels, who are skilled in fine-tuning, data preprocessing and performance optimization, especially when dealing with complex structured and unstructured data.
None of this is uncharted territory
At Nokia, we have already built centers of excellence around open source-based software platforms via central initiatives for developing highly resilient, cloud native telco workloads. The same disciplined and replicable processes must now be adapted for a “verticalized platform” for agentic AI application adoption.
Organizations will also need a clear blueprint that enables reusability, starting with a model farm framework to determine which LLMs and/or SLMs (open-source or commercial) to use and when. It’s imperative to optimally train the model using federated and clean datasets gleaned from across the organization.
With the right teams in place, telcos can take ownership of their AI journey, reduce dependency on third-party vendors and tailor solutions to their exact needs.
Mid-term payoffs: clear, quantifiable gains in 12 to 18 months
The best part? This is not a long-term moonshot. With targeted investments and strategic alignment, telcos can begin seeing real productivity gains within 12 to 18 months.
While we mined 50-plus telco persona-based use cases specifically for Gen AI, several agentic AI high-impact use cases are already within reach. Think of each role in your organization – from planning engineers, operations & care engineers, network strategists, security professionals and product owners – each having agentic AI applications aiding 5x to 10x efficiencies.
Some real-life, low-hanging use cases are:
- End-to-end troubleshooting automation (L1 to L4): Gen AI agents can reduce mean time to repair (MTTR) by automating root cause analysis, resolution guidance and ticket management.
- Design automation: High-level and low-level design processes, often manual and time-consuming, can be semi-automated with Gen AI, cutting planning cycles from weeks to days.
- Service orchestration, customer experience and care integration: Gen AI can unify fragmented systems across provisioning, billing and support, creating a seamless customer journey.
Moreover, many of these cases overlap with end-customer experiences, allowing them to scale across B2C, B2B and B2B2X models.
Conclusion: Telco Gen AI verticalization is not optional — it’s inevitable
The convergence of three forces — the need for cost-efficient simplification, the maturity of open-source Gen AI, and the availability of domain-specific data and expertise — has created the perfect storm for Gen AI verticalization in telecom.
This is not just a technology play; it’s a strategic imperative that drives business outcomes. The telcos that act now will lower costs and improve efficiencies, unlocking new growth vectors in an industry that’s ripe for reinvention. The tools, talent and technology are available — what’s needed is vision and velocity.
Gen AI verticalization is no longer a future consideration. For telcos, the time to act is now.