How AI will enhance optical network automation
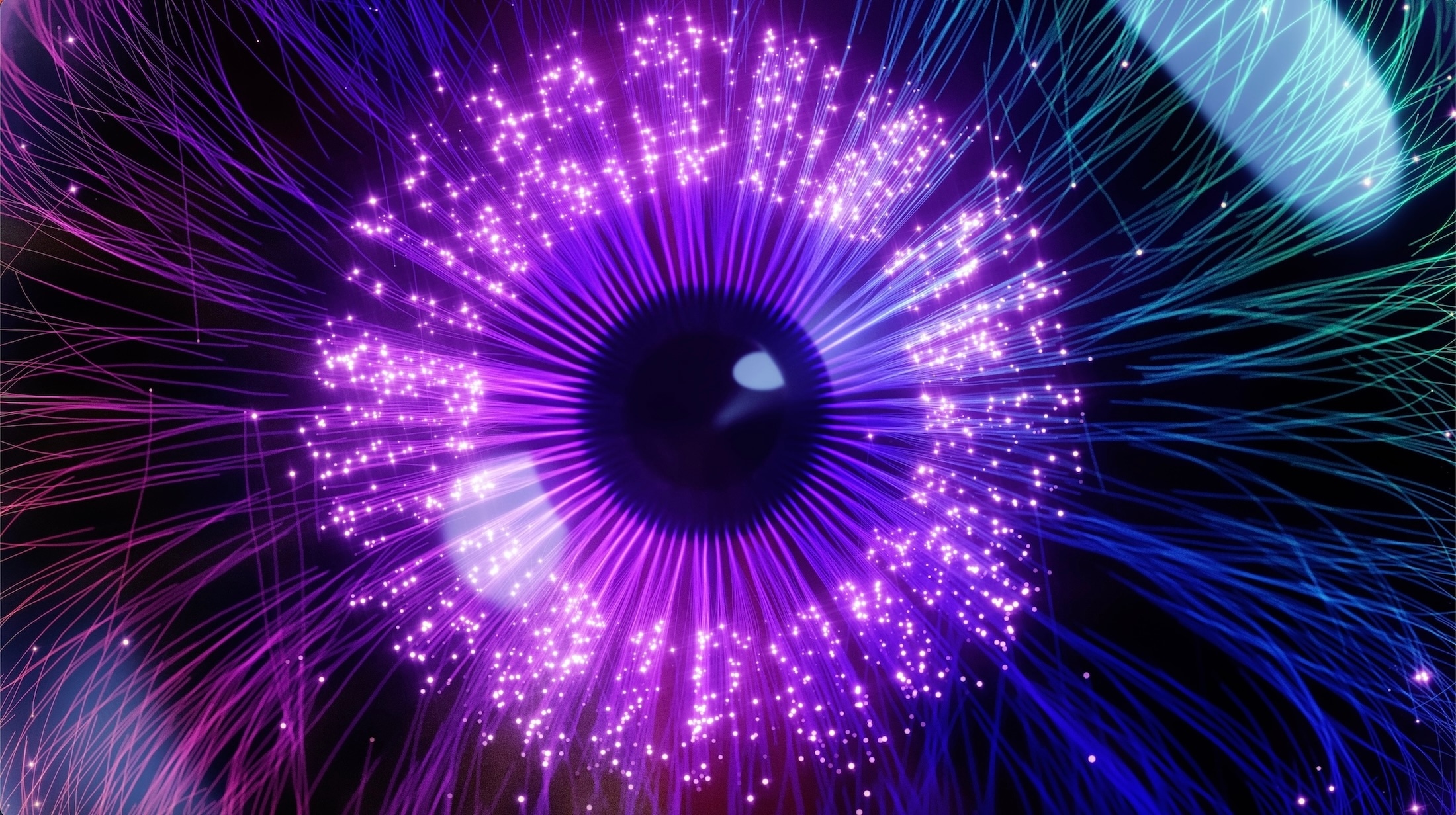
The idea of building artificial intelligence (AI) into optical network automation is gaining traction as network operators take on growing bandwidth demands, network complexity and customer expectations for fast response times.
AI promises to enhance efficiency, predict failures, open new revenue opportunities and streamline operations in optical networks. But despite the excitement around AI, it's essential to assess its practical readiness for real-world applications. Early implementations show good potential, but comprehensive, scalable solutions are still under development.
Let’s take a closer look some of the benefits of using AI in optical network automation—as well as some limitations—to see how it might best be implemented.
Benefits of using AI for optical network automation
AI will simplify network lifecycle management by reducing the time it takes to react, respond to and resolve issues. This can be done by using AI algorithms to:
-
Forecast traffic patterns and recommend configurations to proactively upscale capacity and optimize spectral efficiency throughout the network
-
Identify and diagnose faults in the network, which will shorten repair windows
-
Predict potential failures by analyzing patterns in network key performance indicators (KPIs), which will enable preemptive maintenance and reduce downtime
-
Help network operators resolve network faults or failures by using AI large language models (LLMs) trained on user manuals and troubleshooting guides to propose step-by-step recommendations
-
Ease tasks such as employee onboarding and network element deployment by enabling network operators to use natural language interactions with the automation system to get relevant information from a knowledge base.
These AI applications will undoubtedly reduce the operational effort involved in managing networks. We can use these same algorithms within closed-loop operations where the system monitors network parameters and automatically adjusts the infrastructure to keep the network optimized, initially with human supervision (guided automation) and then without human intervention (full automation). You can read more about closed-loop optical network operations in this blog.
AI will also make it easier to extract information from the network, so network operators can get the insights they need to make decisions about the network or business. We can do this by using generative AI to pull data from various locations throughout the network and assemble reports on specific metrics in a single pane of glass. This eliminates “swivel-chair management” and significantly reduces the time required to generate reports.
By simplifying network lifecycle management and making it easier to obtain network performance metrics, AI will free up network professionals to focus on important business challenges rather than on how to interact with hardware elements and software systems. This will cut the amount of time required for training on networks as technology evolves over time.
Barriers to using AI in optical network automation
Incorporating AI into optical network automation promises to bring numerous benefits to network operators. But operators will have to overcome several concerns and technical challenges to implement it successfully, including:
-
Trustworthiness: The decision-making process of AI systems may not always be transparent or predictable. This can make it difficult for operators to understand how and why certain decisions are made, or cause them to worry the system is “hallucinating” and presenting false or misleading information as fact.
-
Integration with legacy networks: Optical networks frequently contain multiple generations of hardware and software deployed over time. Integrating AI-based automation with these technologies can be difficult and require significant investment and technical expertise.
-
Available expertise: Planning, deploying and managing AI-driven network automation requires specialized skills and knowledge that may not be present within the network operator’s organization.
-
Overdependence: If an issue occurs in a critical network function managed by AI, human expertise must still be available to ensure network continuity and reliability.
-
Security: Data security is very important to network operators, which will want to ensure their AI systems are air gapped.
Addressing these concerns and others involves careful planning, ongoing monitoring and the development of best practices and standards for AI deployment in optical networks.
We’re confident that thoughtfully introducing AI capabilities into Nokia WaveSuite will provide positive outcomes for network operators. Some specific use cases where we think AI will add real benefit include generating reports, quickly finding relevant information in user manuals, enabling conversational intent-based interactions with the automation system, assisting with troubleshooting, performing capacity planning, and gaining a deep understanding of network KPIs.
The possibilities are endless. Network operators can depend on Nokia to make the best use of AI in WaveSuite, so they can get more out of their networks and focus on creating value for their customers.
Nokia WaveSuite and AI