How AI is Revolutionizing Spatial Audio
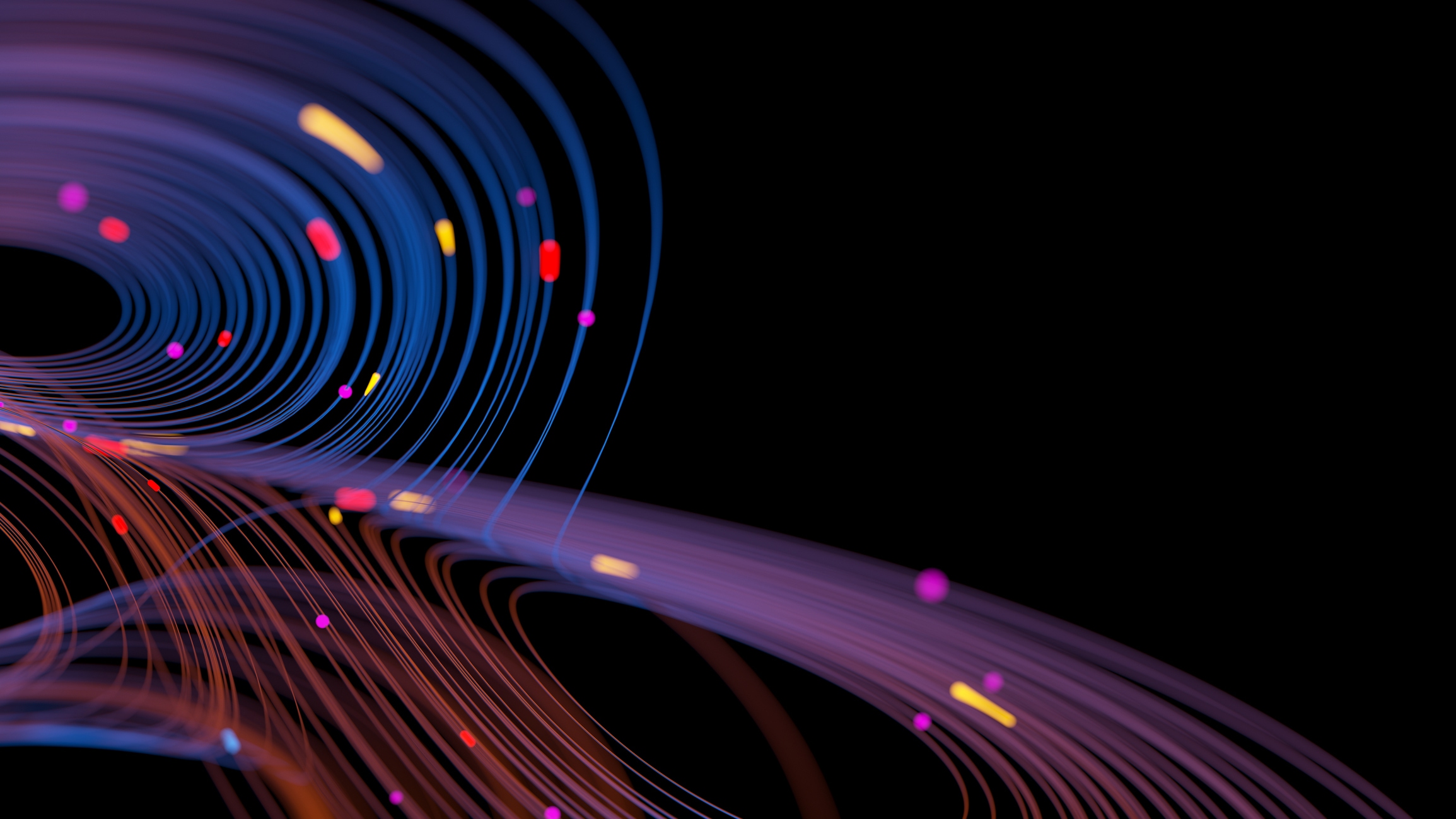
The world of spatial audio is constantly evolving with new technologies emerging that can deliver more immersive and realistic sound experiences. One of the key challenges in this field is Ambisonics encoding, a technique used to capture and reproduce sound from multiple directions.
Enter our latest innovation; In collaboration with Tampere University, we introduced a new technology that transforms immersive audio capture and processing, to deliver improved spatial audio performance while also significantly reducing development costs. Not only will this unlock new possibilities for spatial audio recording, but it will accelerate the adoption of advanced immersive experiences for today’s devices.
The challenge of modern spatial audio capture
Recording spatial audio has always been a rigid process. Existing machine learning-based encoding methods often struggle to accurately capture the full range of frequencies, leading to a distorted and unrealistic sound experience. Even the latest AI audio capture solutions require specific training for each microphone array configuration, making them inflexible and time-consuming.
These drawbacks have created a significant barrier to the widespread adoption of spatial audio technology, particularly in emerging applications like virtual reality and immersive telecommunications.
Creating a universal translator for spatial audio
To overcome these limitations, we've developed a new deep neural network (DNN) based method for Ambisonics encoding. Our solution, the first of its kind, automatically adapts to different microphone array arrangements, without requiring retraining. Think of it as a universal translator for spatial audio—one system that can work with virtually any microphone setup.
U-Net design
The key to our approach lies in a U-Net architecture, a unique dual-system that processes both the physical arrangement or microphone geometry and the audio signals they capture.
The system’s key components include:
- A geometry encoder that understands the physical layout of microphones
- A signal processor that handles the actual audio data
By learning the relationship between the microphone geometry and the audio signals, our neural network maintains high-quality audio processing across different microphone configurations and adapts to different arrangements without needing retraining. Something that has been quite challenging for DNN-based solutions.
Real-world performance
Putting our DNN solution to the test revealed impressive results in controlled environments and we were able to surpass traditional capture methods in accuracy and the handling of spatial audio information. Our technology excels in anechoic conditions, (echo free acoustic environments) consistently delivering high-quality results across a variety of microphone arrangements. And while there are challenges in reverberant environments, (think echo-heavy rooms) our system still outperforms conventional methods in maintaining consistent performance across frequencies.
Industry implications
This breakthrough has far-reaching implications for industries like virtual and augmented reality, where it can enable more immersive experiences with flexible audio capture. Telecommunications can also benefit from enhanced audio quality in video conferencing, while today’s mobile devices can also leverage existing hardware for improved spatial audio capture.
Looking ahead, we see further development focusing on enhancing performance in reverberant conditions and improving the handling of multiple sound sources, paving the way for even more impactful applications in the future.
A fundamental shift in spatial audio recording
This development isn’t just another technical improvement—it’s a fundamental shift in how we can approach spatial audio recording. The ability to use one system across different microphone configurations could significantly reduce development costs and complexity while improving audio quality.
For consumers, this could mean better immersive audio experiences across their devices. It could also open new possibilities for 5G Advanced Immersive Voice and Audio Services (IVAS) powered applications, allowing the spatial audio encoding process to be easily adapted to new devices. For developers and content creators, it offers more flexibility in hardware choices without compromising audio quality. For the industry as a whole, it represents a step toward more standardized and accessible spatial audio solutions.
Our work demonstrates that AI can solve real-world audio engineering challenges in novel ways, opening doors for innovation in spatial audio technology. As virtual and augmented reality continue to evolve, such advances in audio processing will become increasingly crucial for creating truly immersive experiences.
This research represents not only a technical achievement, but a practical solution to a long-standing challenge in spatial audio processing, paving the way for more flexible and accessible immersive audio technologies.
Read more in our latest paper, “Gen-A: Generalizing Ambisonics Neural Encoding to Unseen Microphone Arrays.”