Owning uncertainty to get the most from optical networks or road trips
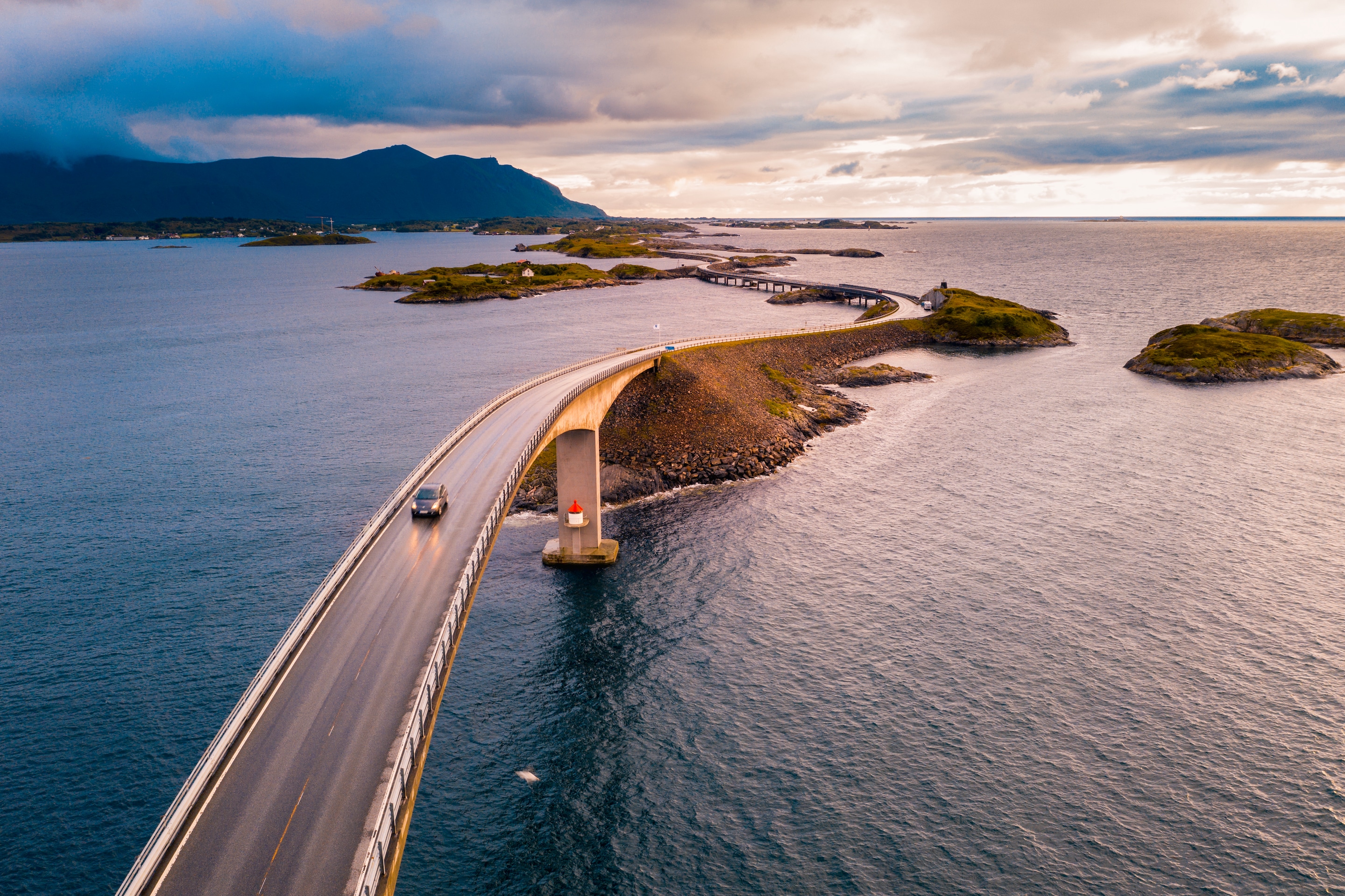
The ambitious tourist
Some years ago, I was excited about planning a road trip around Crete with my wife. Beautiful beaches, exquisite cuisine, ruins with ancient scripts and so much more. However, there was a little problem. The places we wanted to visit were scattered over 8,000 km2 and we had just eight days.
Fig. 1: Touristic sites in Crete
It seemed we would have to make sacrifices. Could we afford to miss the pink sands of Elafonisi Beach in the southwest? Should we skip Vai Beach and its palm trees in the northeast? Should we walk the gorge of Samaria or instead visit the prehistoric city of Knossos? Take a day trip to the tiny golden island of Chrysi or swim at Matala, where hippies flocked in the 60s? I did not want to miss any of these legendary places! If only we could build a perfect plan to fit everything in.
Fortunately, I had the internet at my fingertips. Within a few minutes I found a detailed map with travel distances, information about typical traffic conditions and user data on average wait times in museums and restaurants. However, unknowns are everywhere when it comes to traveling. How could I be sure that the average times would apply in our case? I thought about adding some time margin on top of the calculated itineraries and visiting durations, but things were still not very clear. Should I consider Murphy’s law or throw caution to the wind?
In my engineering parlance, I told my wife that there appeared to be an interesting trade-off between unnecessary “dullness” and unnecessary “stressfulness” in our vacation planning. On the one hand, being conservative and choosing to visit very few places would give us a safety net if things went wrong. But it would also make the trip really boring! On the other hand, taking a lot of unnecessary risks could ruin our vacation by leaving us tired, stressed and feeling lost. But what was the best possible choice? My wife thought I was overcomplicating things but she let me explain.
Planning network connections is like planning holidays
The problem of setting up lightpaths (i.e., wavelengths) in optical networks has striking similarities to vacation planning. When we plan a holiday, we want to make the best of our time and visit as many places as possible. When operators plan their networks, they want to squeeze the most out of their hardware and offer the highest possible capacity or transmission distance to their customers. However, just as a holiday plan can fail because of random events, a network connection can fail at some point during its lifetime.
Fig. 2: Qualitative graphs of (a) system capacity versus system GSNR for planning with different risk levels and (b) the probability density function of the GSNR.
Conservative network planning with over-dimensioned margins (the reality for most installed systems today) prioritizes safe operation over resource optimization. This choice is undoubtedly safe, but at the same time rather boring. Accepting more risks to visit more places is a choice for adventurous travelers, just as accepting more risks to increase network performance and lower cost is a choice for adventurous network operators. Excessive risks don’t make much sense for vacation or network planners: No one wants to make family members, network operators or video streaming subscribers unhappy. Therefore, the second choice seems most promising, even if it does involve some risk.
Part (a) of Figure 2 shows a qualitative graph of ultimate system capacity versus the system’s generalized signal-to-noise ratio (GSNR) for planning with different risk levels. Ultimate system capacity and system GSNR are analagous to vacation fun and available vacation time, respectively. The solid black line shows a conservative plan. The dashed and dotted lines show more daring plans. The vertical and horizontal arrows show the potential capacity and distance benefits of risky planning compared to conservative planning.
Part (b) of Figure 2 shows a qualitative graph of the probability density function (PDF) of the GSNR. If GSNR is below the forward error correction (FEC) threshold SNRFEC, the system stops operating, leading to unhappy customers and network operators. The probability of such an event (called outage probability) is equal to the red shaded area under the PDF curve. The distance between the nominal system GSNR, GSNRn, and the limit SNRFEC is called margin. Claiming more capacity pushes SNRFEC towards higher values and therefore increases the outage risk.
So how does a network operator make the right compromise? It would help a lot if we could somehow quantify the risk.
The uncertainty behind margins
The GSNR margin in network planning actually depicts the knowledge of the system at a certain moment during its lifetime, and perhaps also the short- or long-term expectancy for its evolution. For instance, if we believe that we know our system perfectly well at a certain moment and we are absolutely sure it is not going to evolve at all, then we theoretically need to take zero margin. On the other hand, uncertainty or imperfect knowledge of our system (which is usually the case) means that we need to consider an additional margin to ensure that the system always operates with a GSNR above the FEC threshold.
When it comes to network planning, monitors provide information that’s somewhat similar to what the internet offers for vacation planning. Good search engines and web-based services provide real-time details on travel times, road conditions, traffic congestion, estimated wait times and other useful information. Monitors provide continuous measurements of some physical parameters of the system and actual GSNR statistics for previously established connections over the same infrastructure. This data can feed an application, such as Nokia WaveSuite Health and Analytics, which processes it and triggers alarms or actions.
However, quantifying the risk in network planning requires one more step beyond providing expected values. Planners also need a measure of uncertainty (or accuracy).
Owning the uncertainty
To get a measure of uncertainty in vacation planning, a traveler would need a website that could provide the expected duration of a given trip and describe how much one could trust this information. Indeed, slow drivers like me might take more than the average time. But how much more? This could be described by the standard deviation from the average trip time of all previous travelers who did the exact same trip.
Information that can serve as a measure of the uncertainty of monitored quantities can help improve network planning. This information is often captured by the standard deviations of previously collected data or offline characterizations with the help of accurate laboratory measurements. It can be arbitrarily high or low depending on the accuracy of the employed monitoring method and other parameters. In all cases, owning this uncertainty a priori (i.e., knowing it and accepting it) helps us quantify the risks and make better decisions.
So will everything go wrong in the end or should we be more pragmatic about our planning? We don’t really know, so the final planning decisions in the worlds of holidays and networks are a matter of personal taste! Some planners are willing to take more risks and others aren’t.
Really bad luck, or simply just another day?
At Nokia Bell Labs, we developed a mathematical model that can analytically assess the performance of monitoring-enabled optical transmission systems when the uncertainty of the input parameters is controlled and owned. This is a first step toward quantifying the trade-off between potential benefits and associated risks in optical network planning. The model uses the notion of correlation of input random variables and a confidence parameter to quantify the different choices based on the level of risk we are willing to embrace.
A rough calculation for a holiday plan could, for instance, indicate that we would need approximately 30 minutes of margin, with a confidence of approximately 67 percent. This would involve setting the confidence parameter n=1. If we wanted to increase our confidence to 95 percent, we would need to set n=2 and therefore double our margin to 1 hour. We could even set n=3 and allow for 1.5 hours of margin to be 99.7 percent sure. In other words, the confidence parameter can quantify our desired conservativeness on the final result. I am pretty sure my wife would set a higher n than I would for our holiday planning! The same goes for optical operators. An operator willing to take more risks would set a lower n compared to a more conservative operator.
Correlation is slightly different. In holiday planning, fully correlated events would mean that our most pessimistic predictions about traffic, museum queues and restaurant wait times would come true – at the same time. This type of planning assumes that “everything that can go wrong will indeed go wrong and this will happen in a somewhat orchestrated, systematic way.” In the context of our previous example, our model would then predict 45 minutes (instead of 30) for 67 percent confidence to visit the exact same places as before.
Sometimes fully correlated inputs make perfect sense and are not just science fiction. For instance, heavy traffic and long queues in museums in a specific area could both be caused by the fact that there happen to be a lot of tourists around. However, it would be challenging to justify the idea that long queues in one museum would necessarily (or systematically) mean long queues in another museum on a different day or a different part of the island. Such an event would be bad luck, not the universe conspiring against us.
Fig. 3: Example of PDFs for the GSNR of a typical system. Different correlation scenarios are considered with a) uncorrelated, b) partially correlated or c) uncorrelated input random variables. Analytical models are developed to capture the PDFs for any considered correlation scenario (solid and dashed lines) and their accuracy is benchmarked against Monte Carlo simulations (markers).
The picture is qualitatively similar in network planning. The physical system parameters are initially given by component data sheets and a first set of engineering rules (e.g., power settings). During the system’s lifetime, physical parameters may change and engineering rules may also be willfully reconsidered.
In modern networks, physical parameters may be constantly monitored and their values reported in a data lake. The network tools (e.g., Nokia WaveSuite Network Insight applications) have access to this data and may eventually trigger an action, such as changing the settings to increase capacity or rerouting a connection.
Apart from the uncertainty associated with each monitoring method, it would be useful to know if there is correlation between different monitored system parameters. For instance, amplifier noise figures and fiber dispersion parameters both increase with temperature. If these parameters are monitored for a transmission fiber and an amplifier in close proximity, their values may appear to be correlated since they will both appear to change at the same time. Another example of correlated uncertainties (or errors) appears if a method with a systematic bias is used to monitor several different physical quantities at the same time. Once again, different measurements will appear to be correlated.
In all cases, including such forms of correlation in our planning helps us build better planning tools and use our network more efficiently. On the other hand, if different physical parameters are not influenced by the same subjacent phenomena and they are monitored by completely different methods, then estimations should be uncorrelated. Even if this latter case appears to be more logical, the majority of the systems installed today implicitly consider full correlation between input parameters, i.e., all worst-case scenarios happening at the same time.
OK to be more daring but what’s the reward?
More daring in vacation planning means taking more risks to potentially get to see more places. But is it really worth it to risk one day’s plan falling apart to visit two more amazing destinations? Once again, the answer is subjective and a matter of personal taste.
So what should operators expect if they take more risks in planning their networks? In our paper, we showed that the correlation between different input parameters can be set arbitrarily to fine-tune network planning. The differences between correlation scenarios roughly quantify the expected benefits of more daring network planning. An indicative analysis shows that fully correlated inputs (Murphy’s law) versus partially correlated (but still conservative) inputs could reduce GSNR uncertainty by up to 1 dB. This translates to increased wavelength propagation distance and network capacity. The amount of increase depends on the application. Some networks will see little to no benefit but the gains can be significant for other networks.
Operators can expect similar benefits if they switch from partially correlated inputs to the more daring scenario of uncorrelated inputs. A 50 percent potential increase on propagated distance for free is an attractive feature for network operators because it has a direct impact on the overall cost per transmitted bit. In another recent paper, we go one step further and use real network data to quantify the system’s availability if its capacity were to be updated.
Quantifying the unknown
Knowing more about a system and the monitoring methods it uses will improve an operator’s ability to optimize it and safely reduce margins to the absolute necessity. As more such data becomes available, the operator can capture and use more correlations (even strange ones) for future network planning. The ultimate aim of our tool is to transform knowledge into margin reduction and operator savings.
The only question we cannot answer is whether an operator should take more or fewer risks. However, operators can further reduce risk by using multiple algorithms to monitor performance. One such algorithm is described by Matteo Lonardi in the preceding post in this blog series. This approach is analogous to going online during a vacation to see whether our planning correlation assumptions still hold true. Operators can also reduce risk by using lightpath protection and restoration techniques such as Nokia’s GMPLS-based wavelength routing implementation. In the end, risk is a personal choice or is dictated by the operator’s strategy. With the right tools, operators can work to minimize risk so that they can get most from their networks, and that’s the way it should be.
Learn more from a product perspective below.
Application notes:
Nokia Insight-driven optical networks
Nokia WaveSuite Network Insight