Our blog
Discover networking innovations transforming the world
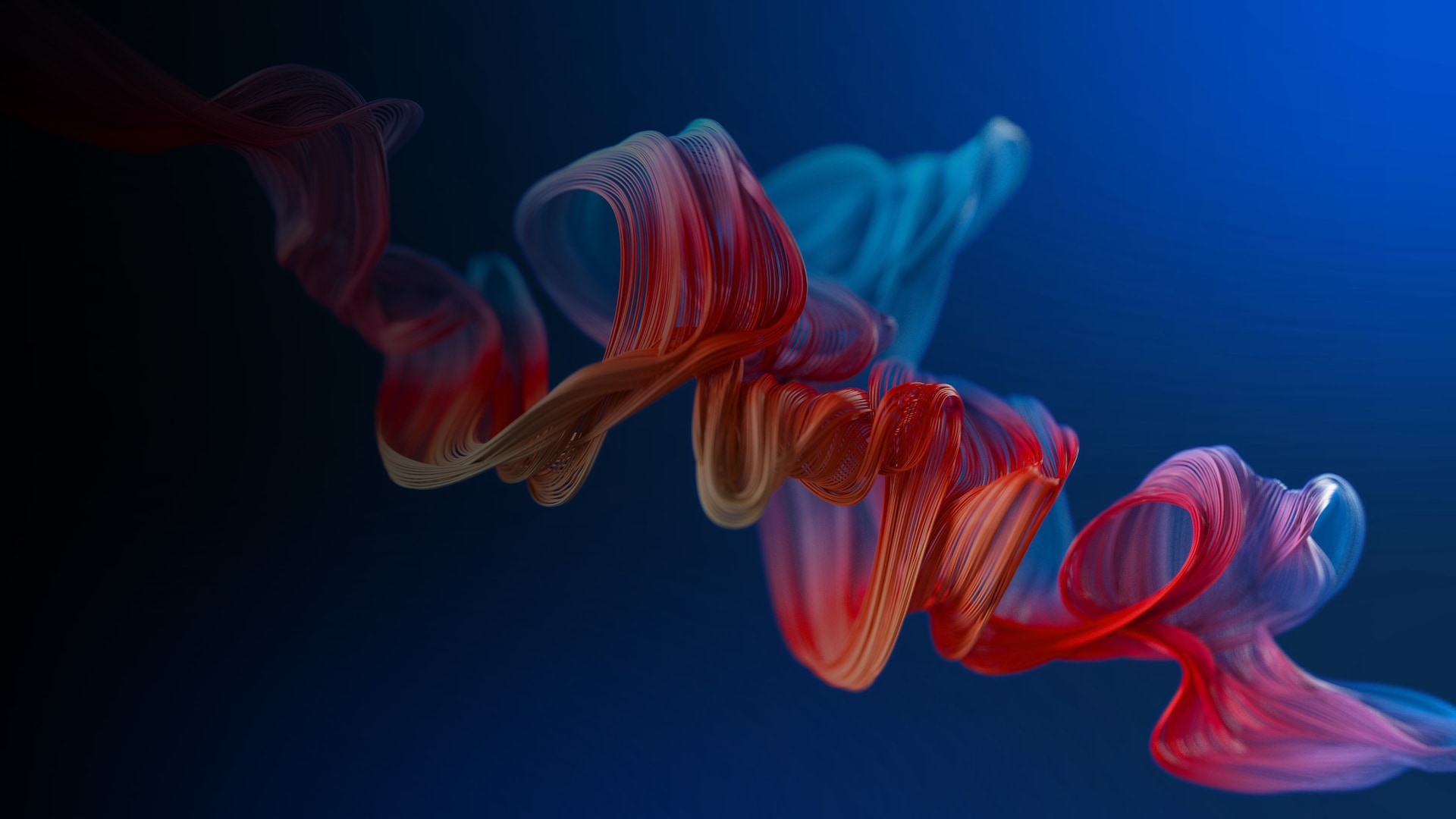
Featured blogs
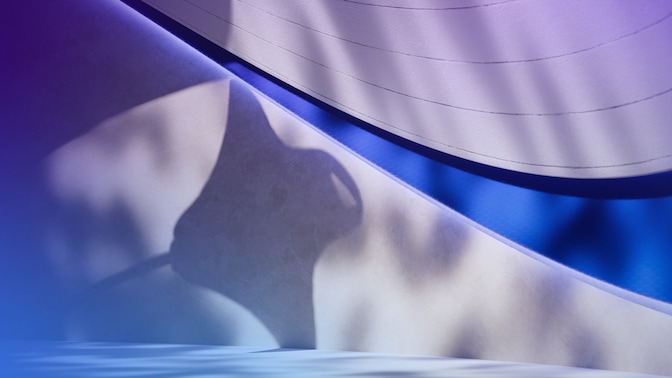
The future of AI and automation is here
AI and automation are reshaping the telecom industry. Nokia is leading this development with advanced technology solutions.
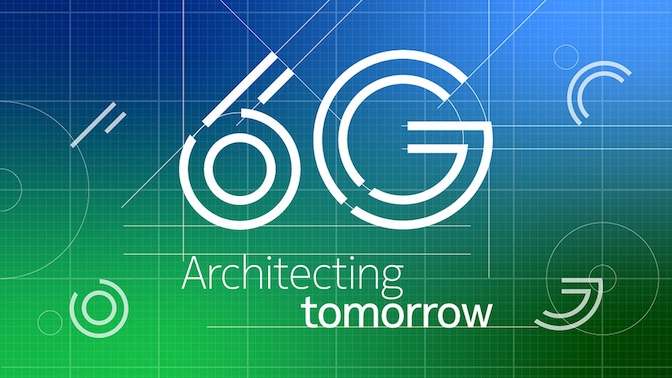
The 6G train has left the station
The 6G train has left the station
Latest blog posts
Networks
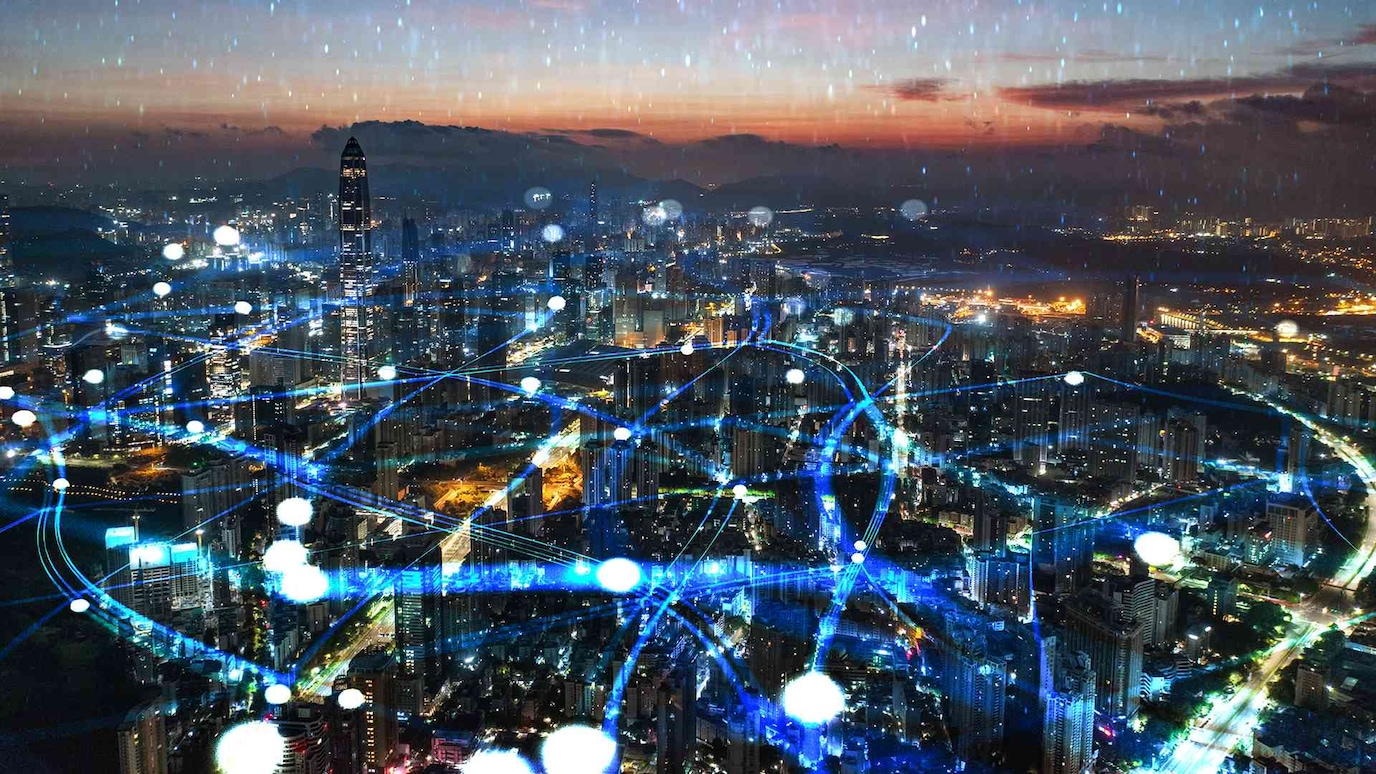
8 Jul 2025
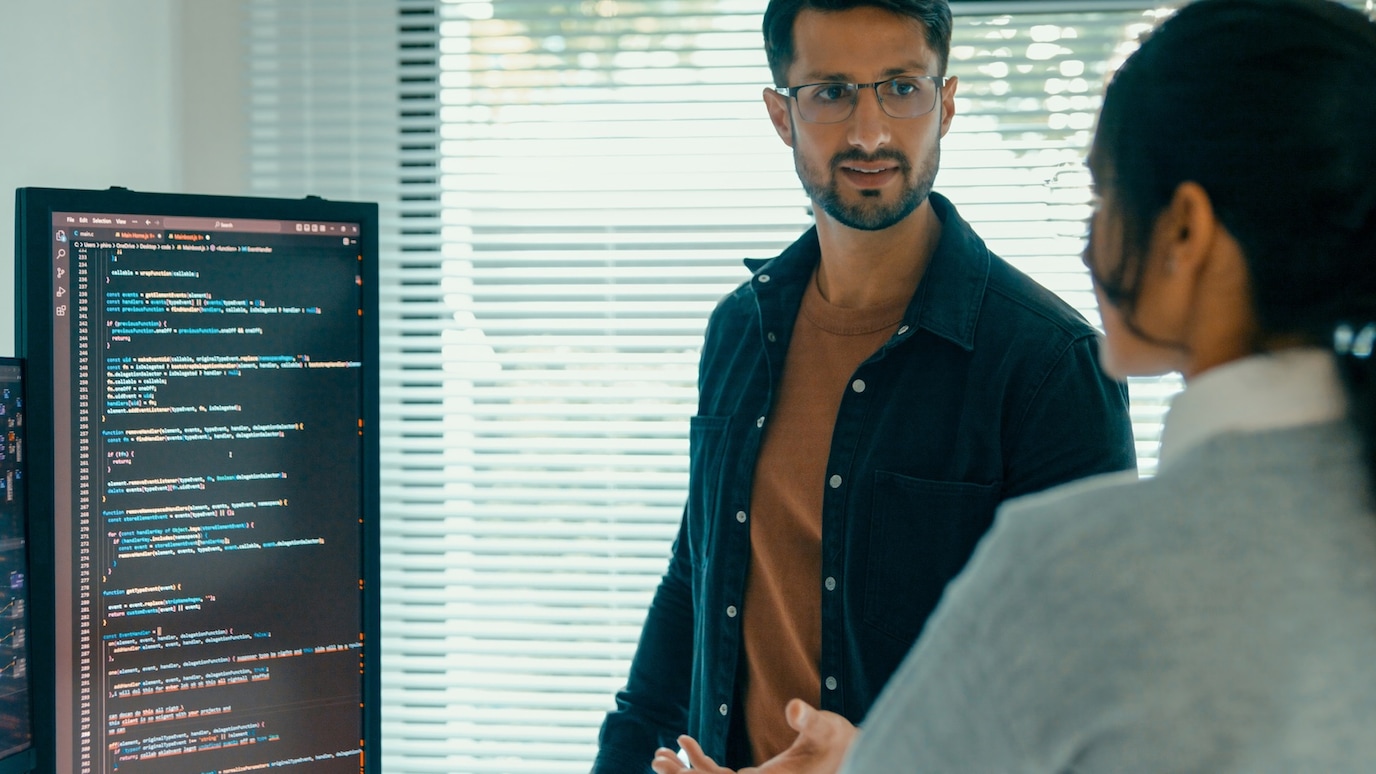
8 Jul 2025
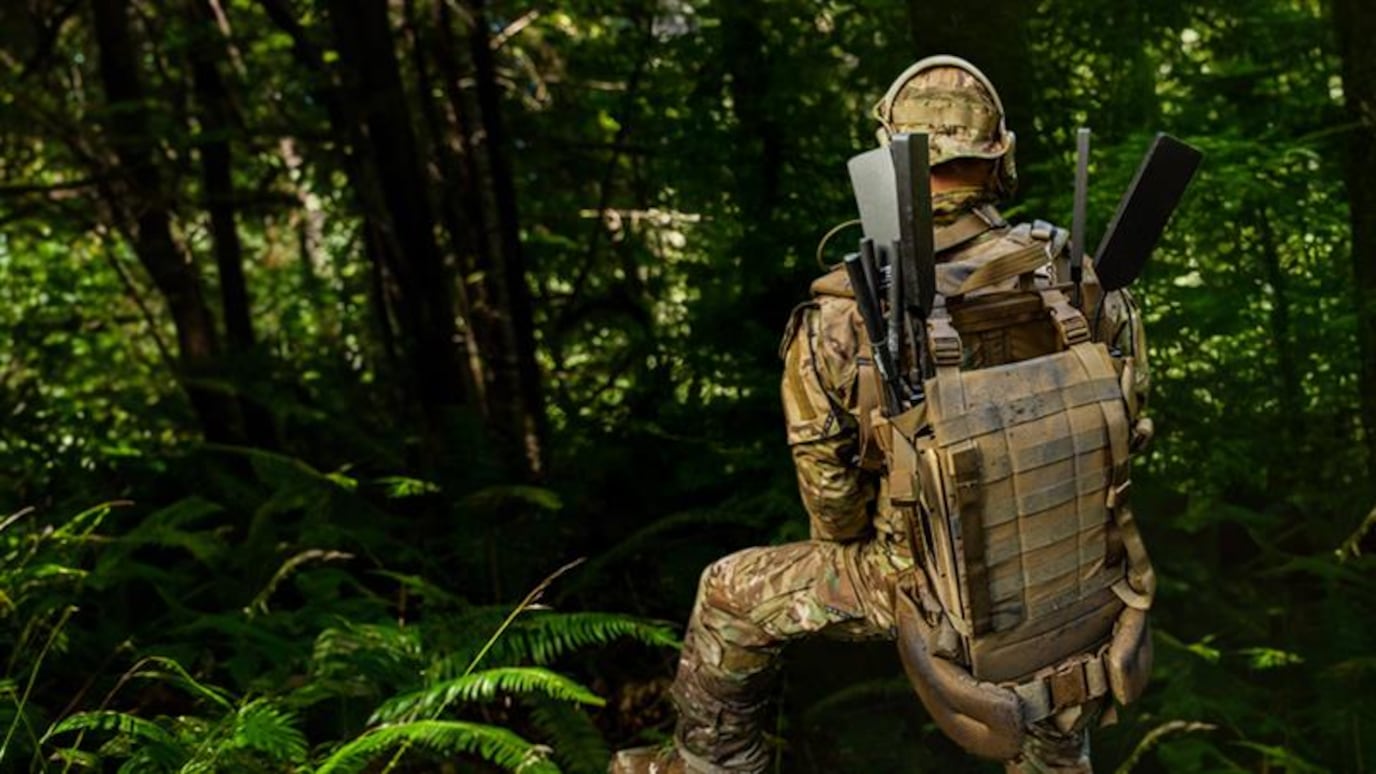
3 Jul 2025
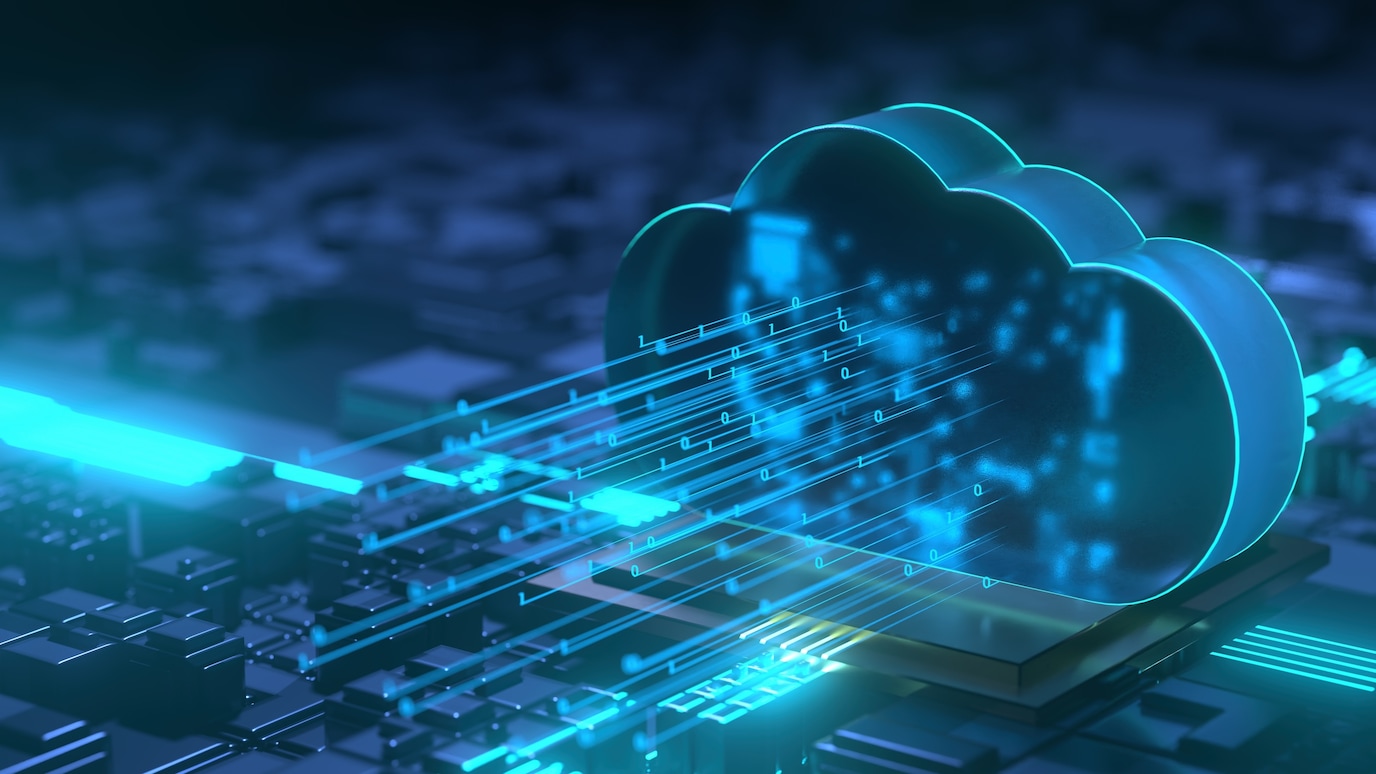
30 Jun 2025
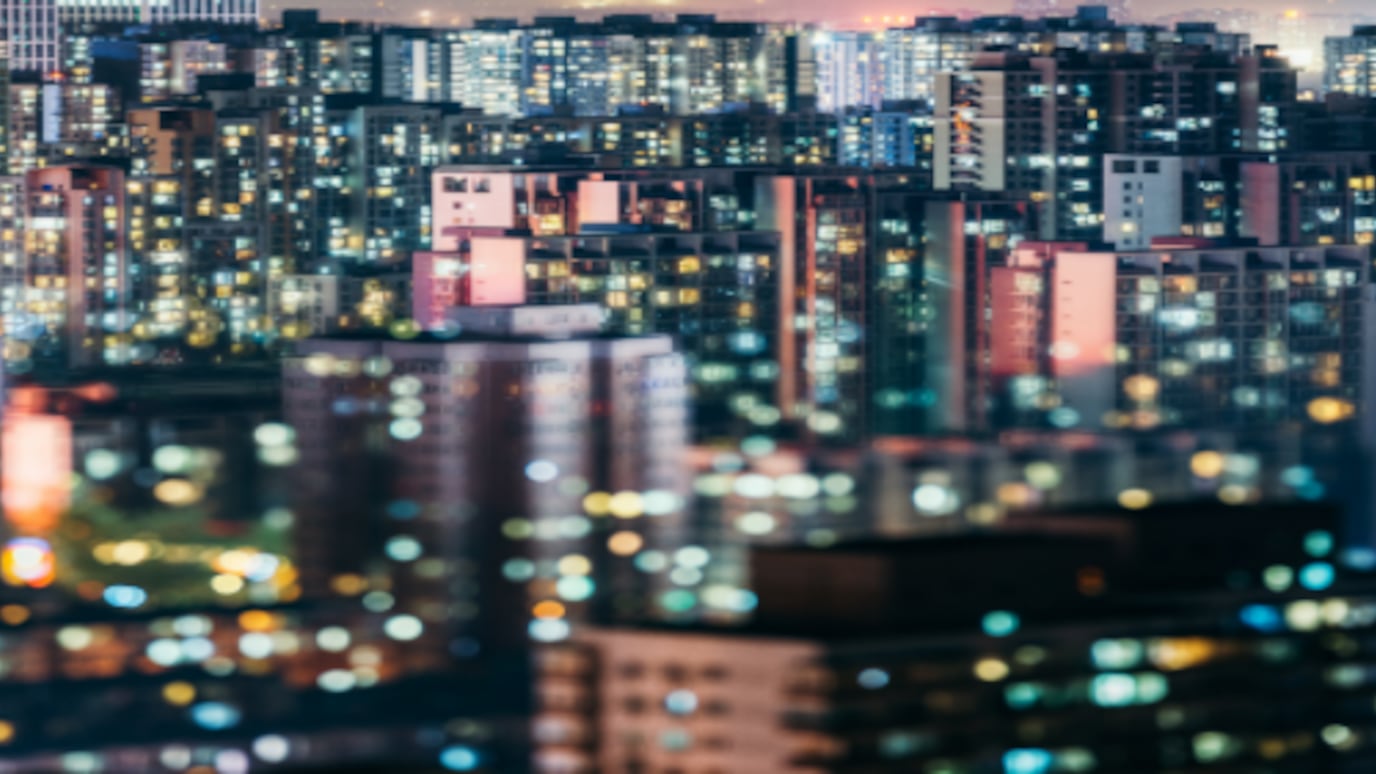
26 Jun 2025
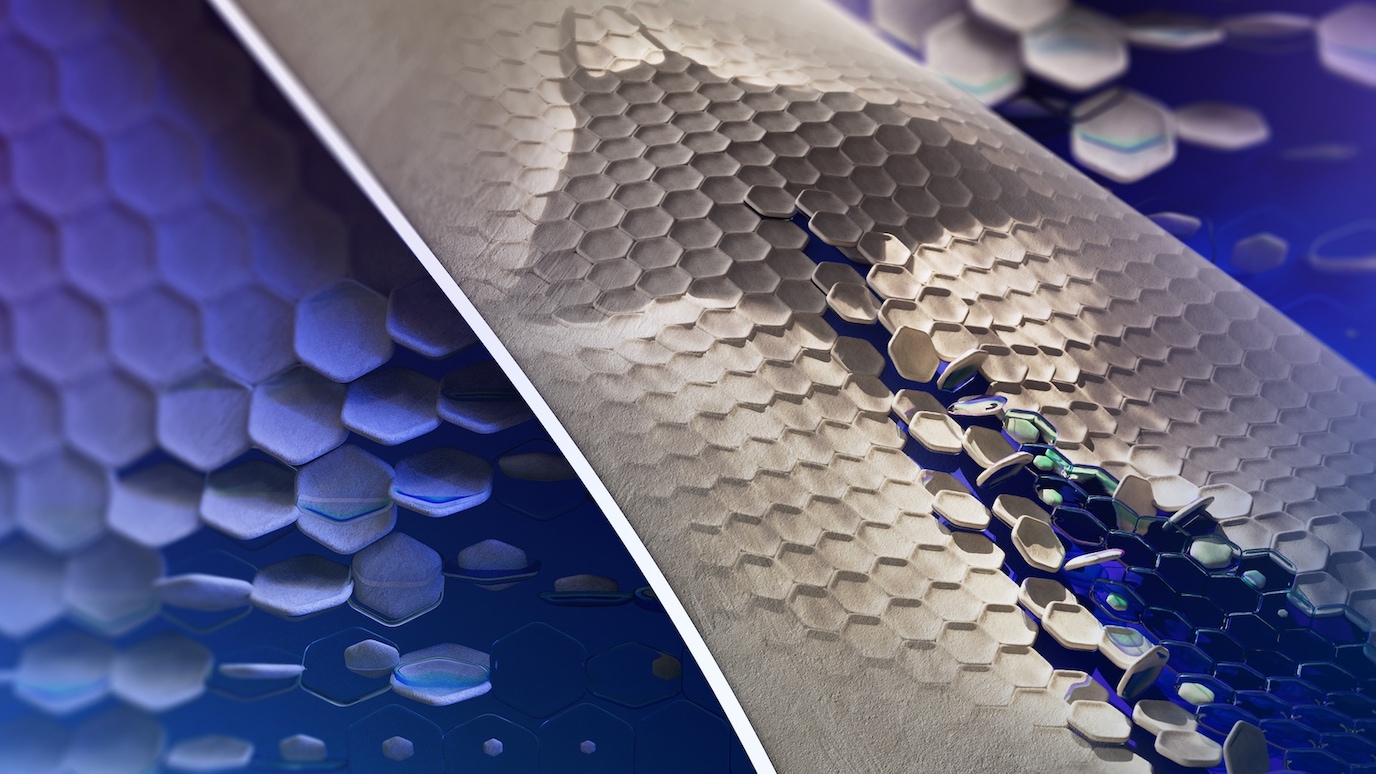
25 Jun 2025
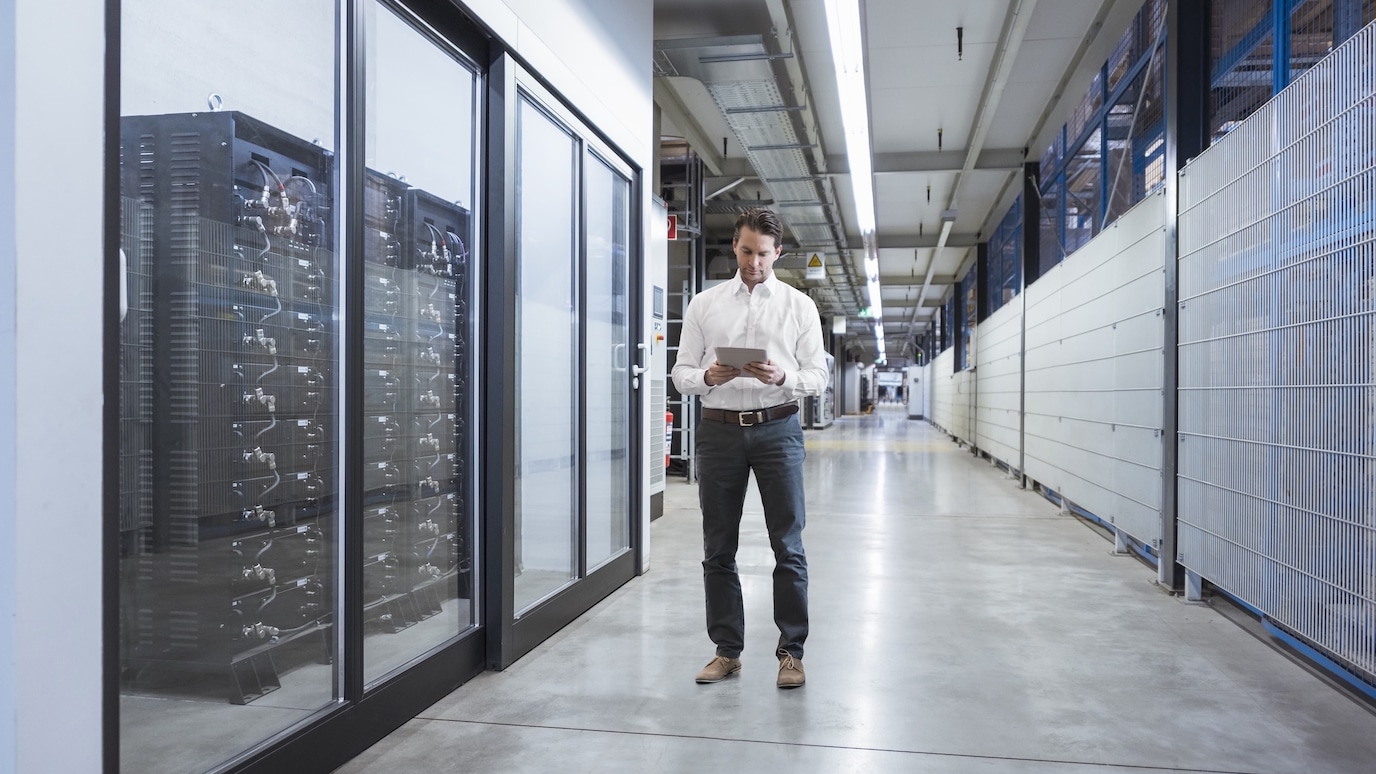
23 Jun 2025
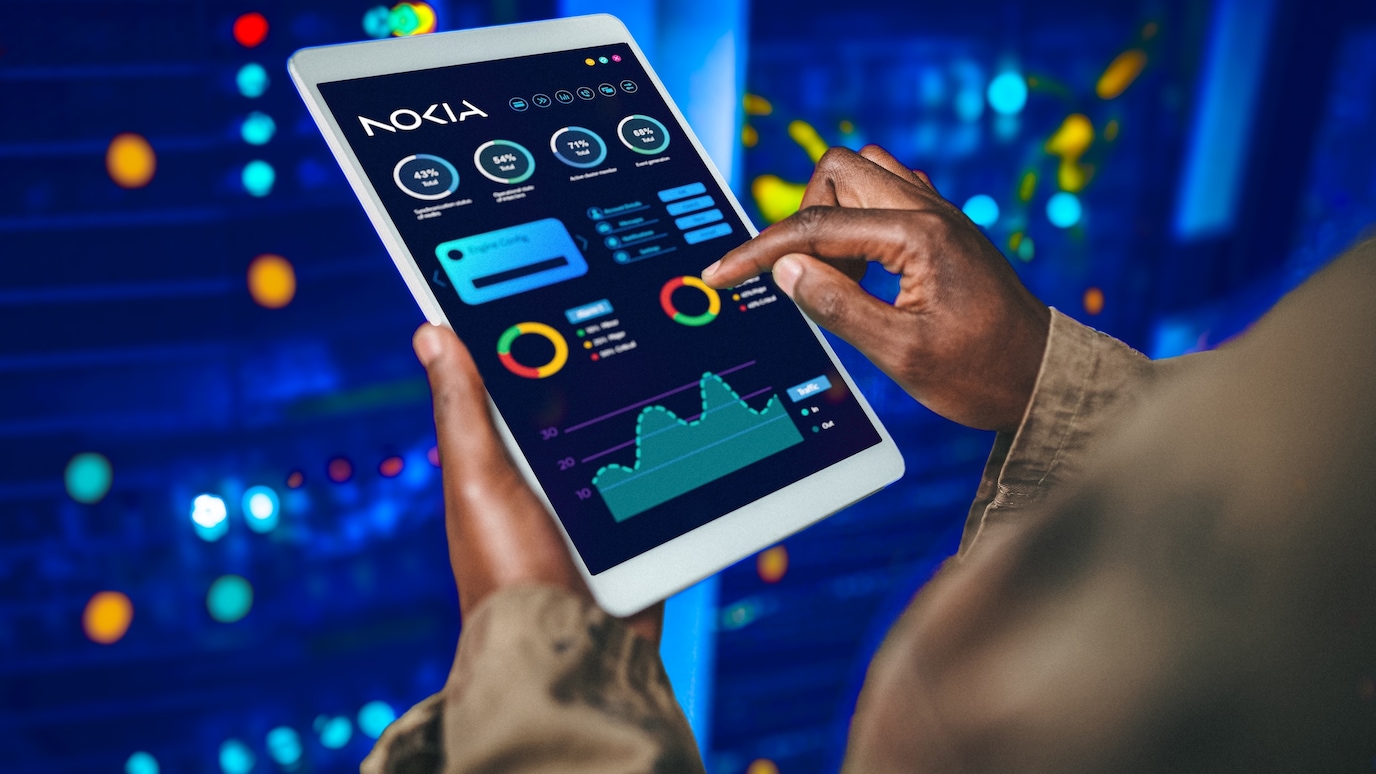
20 Jun 2025
Innovation
Nokia Bell Labs
Enterprises
Partners and licensing
Sustainability
Company
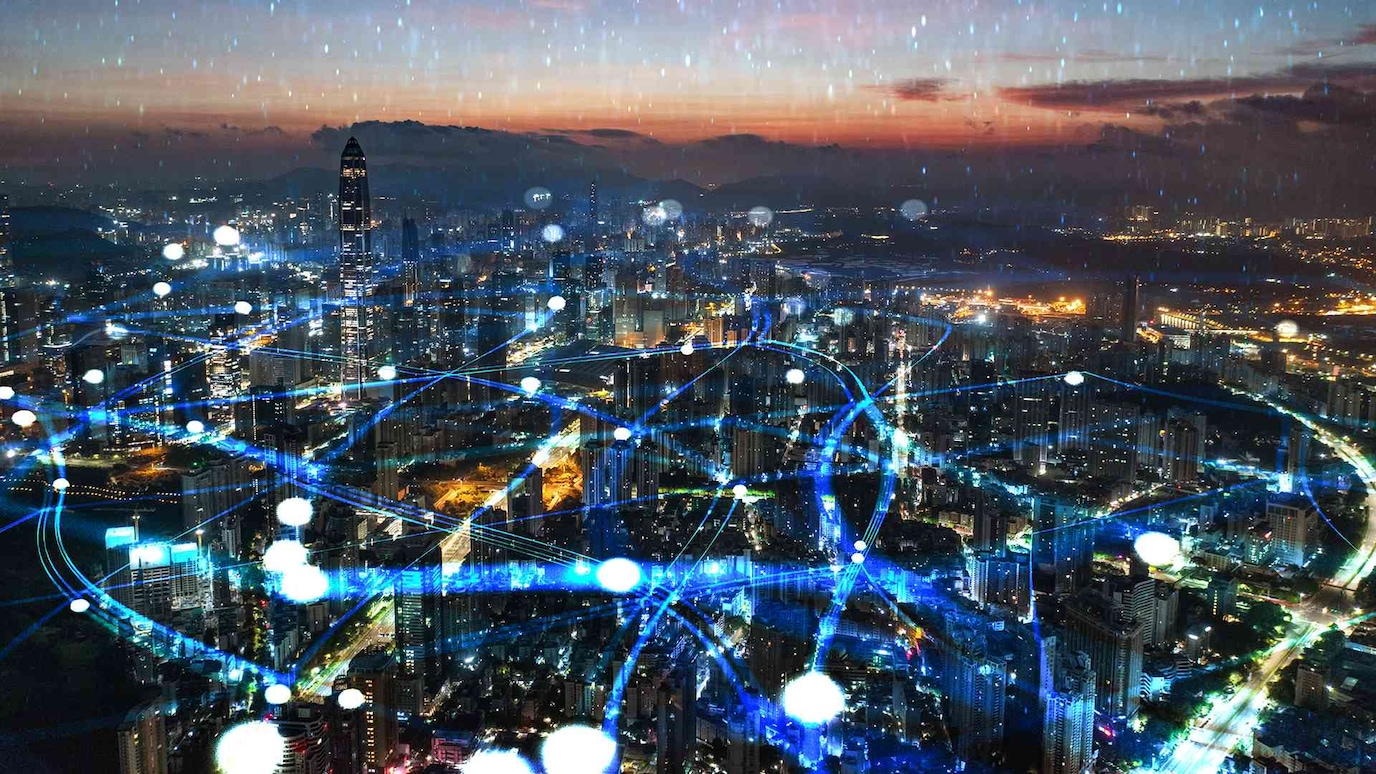
8 Jul 2025
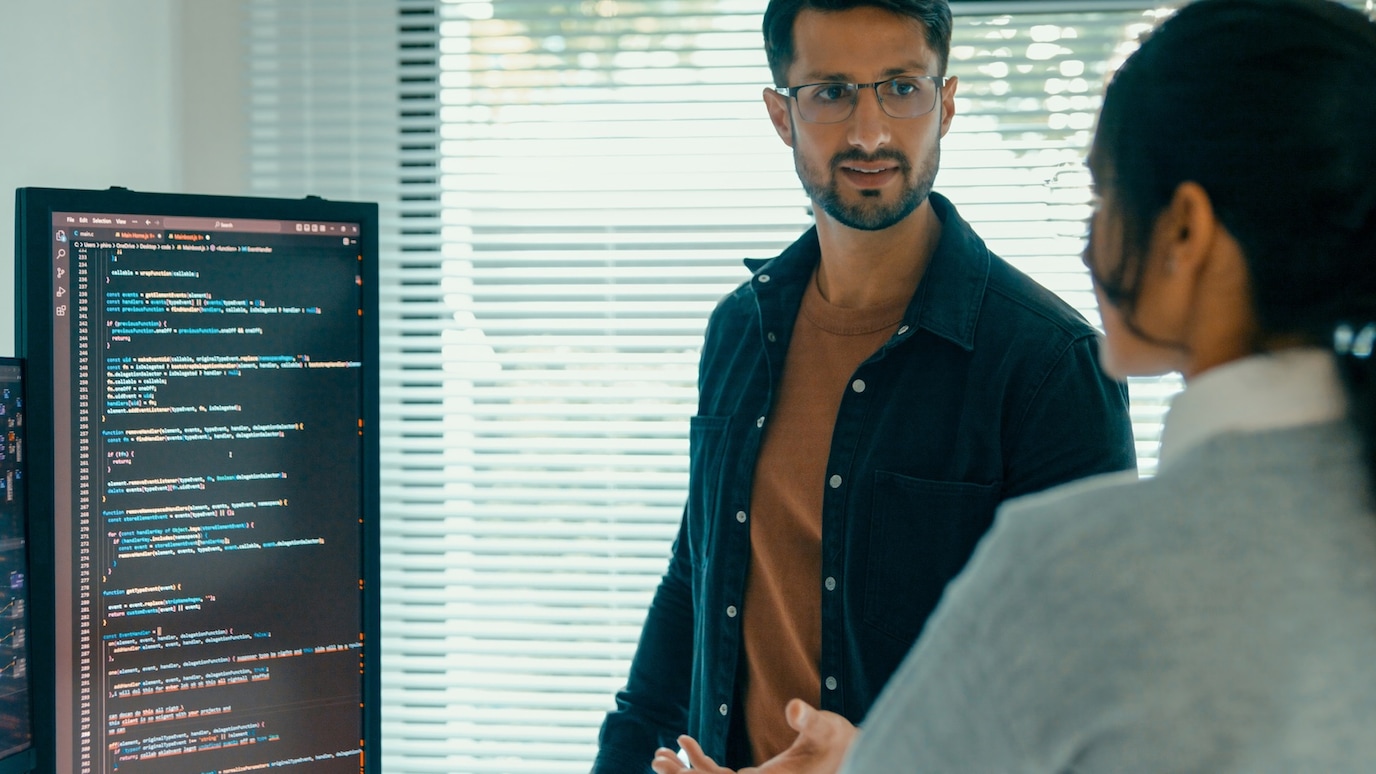
8 Jul 2025
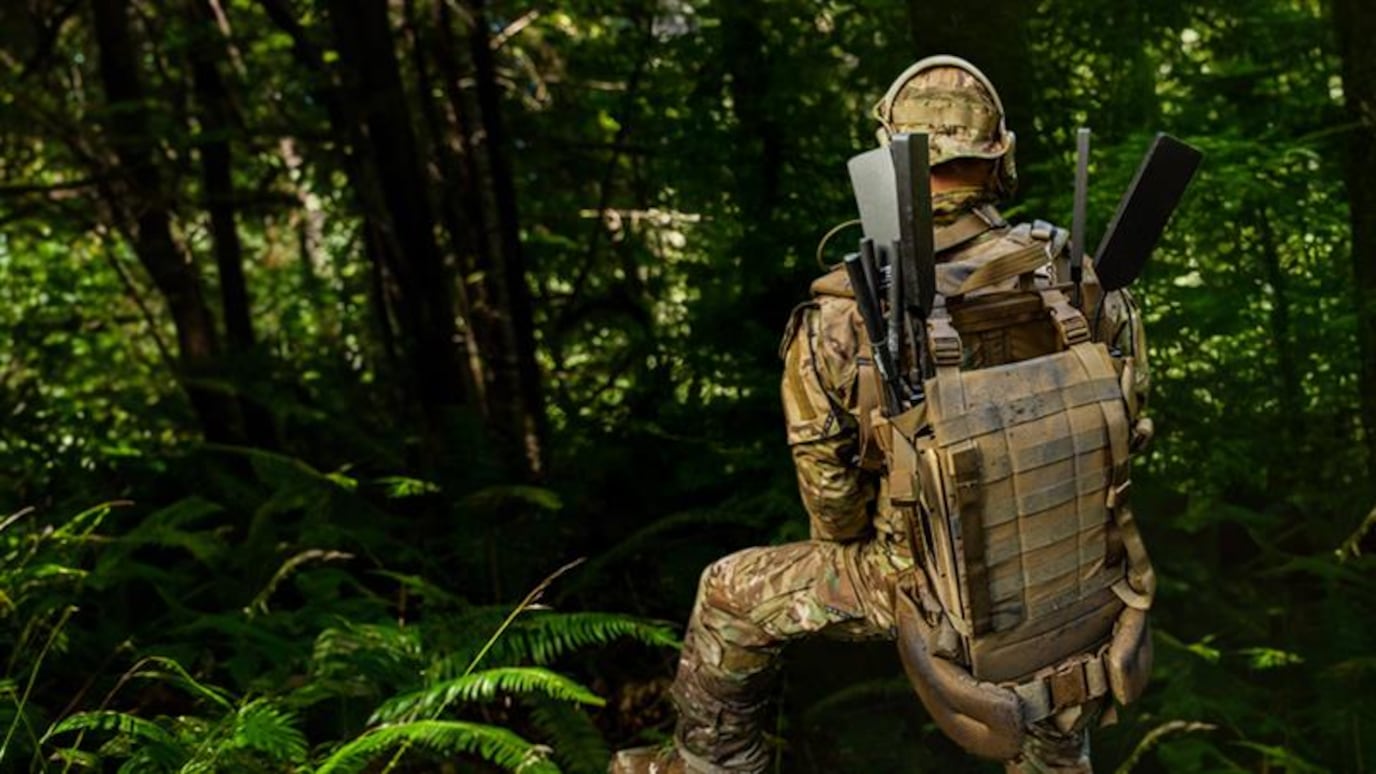
3 Jul 2025
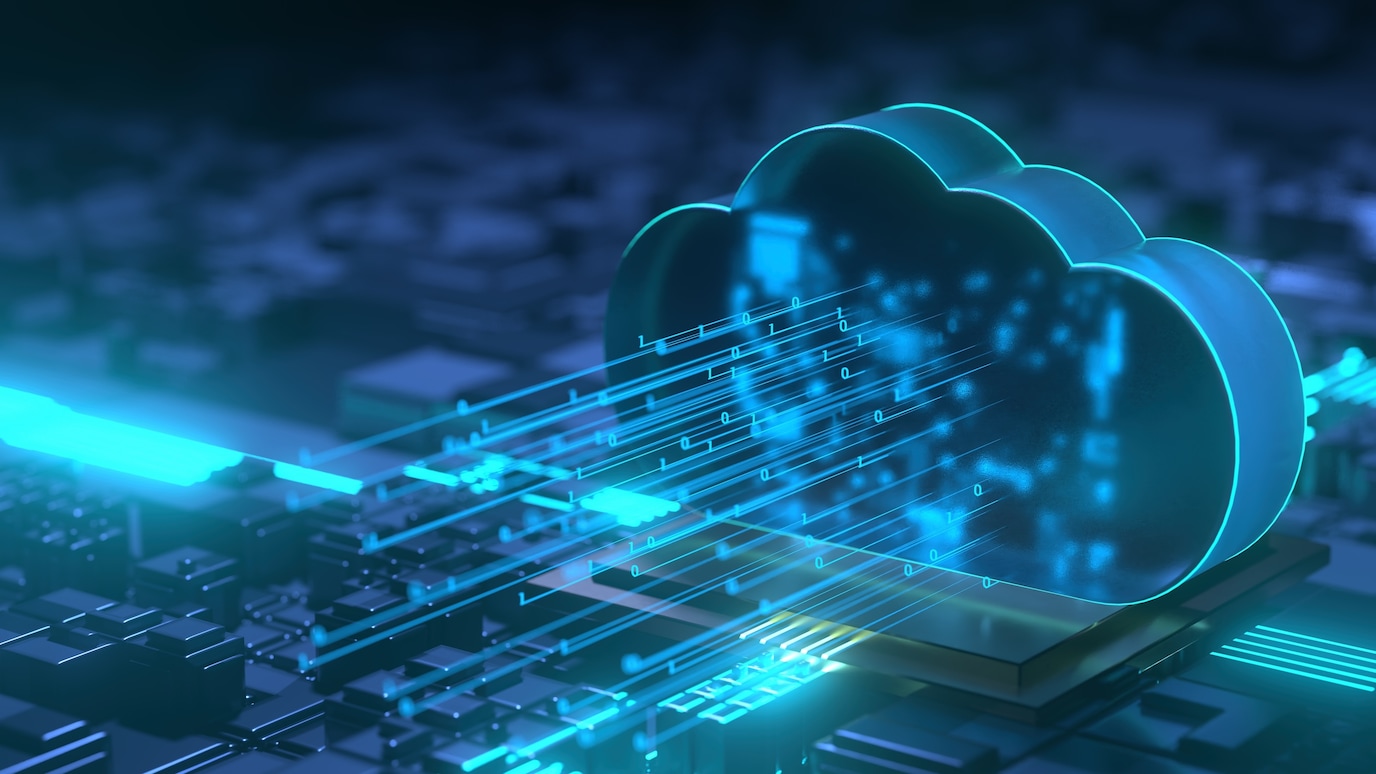
30 Jun 2025
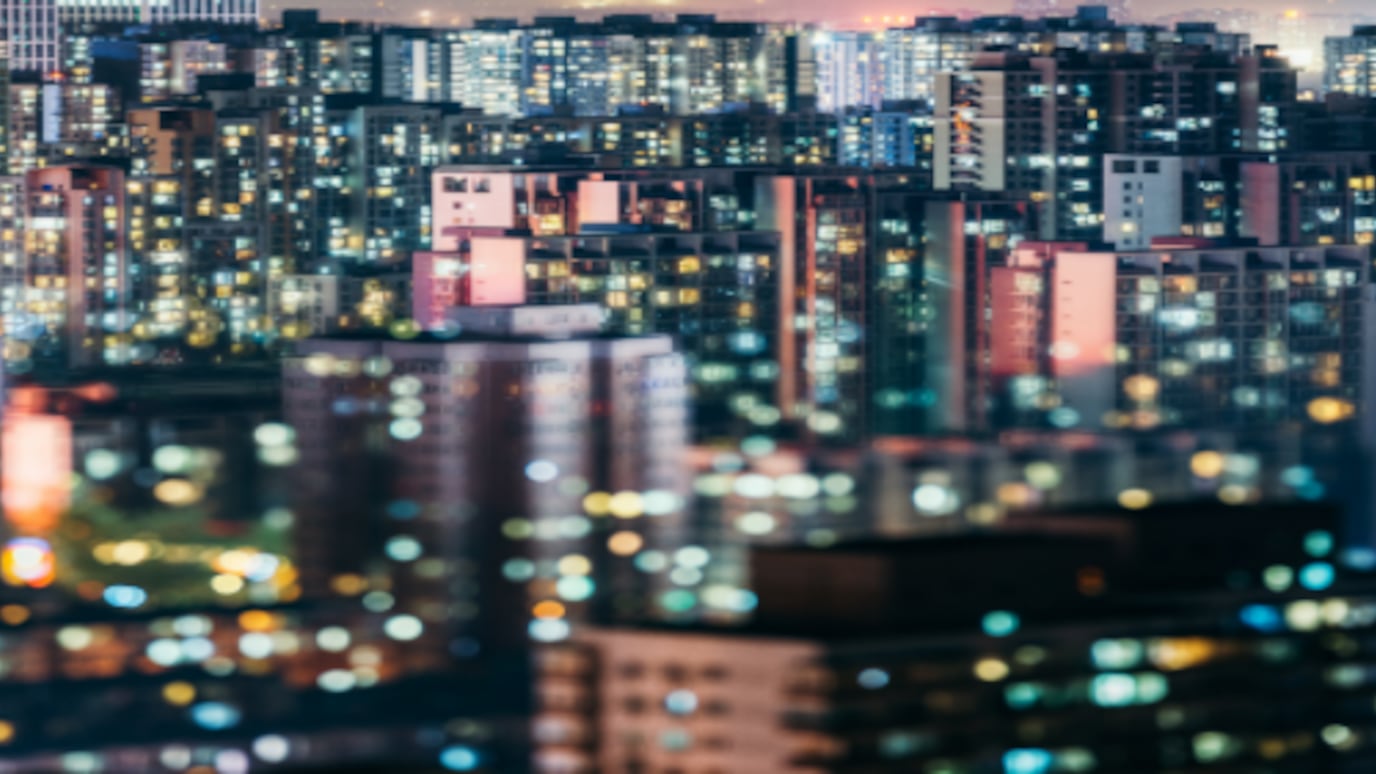
26 Jun 2025
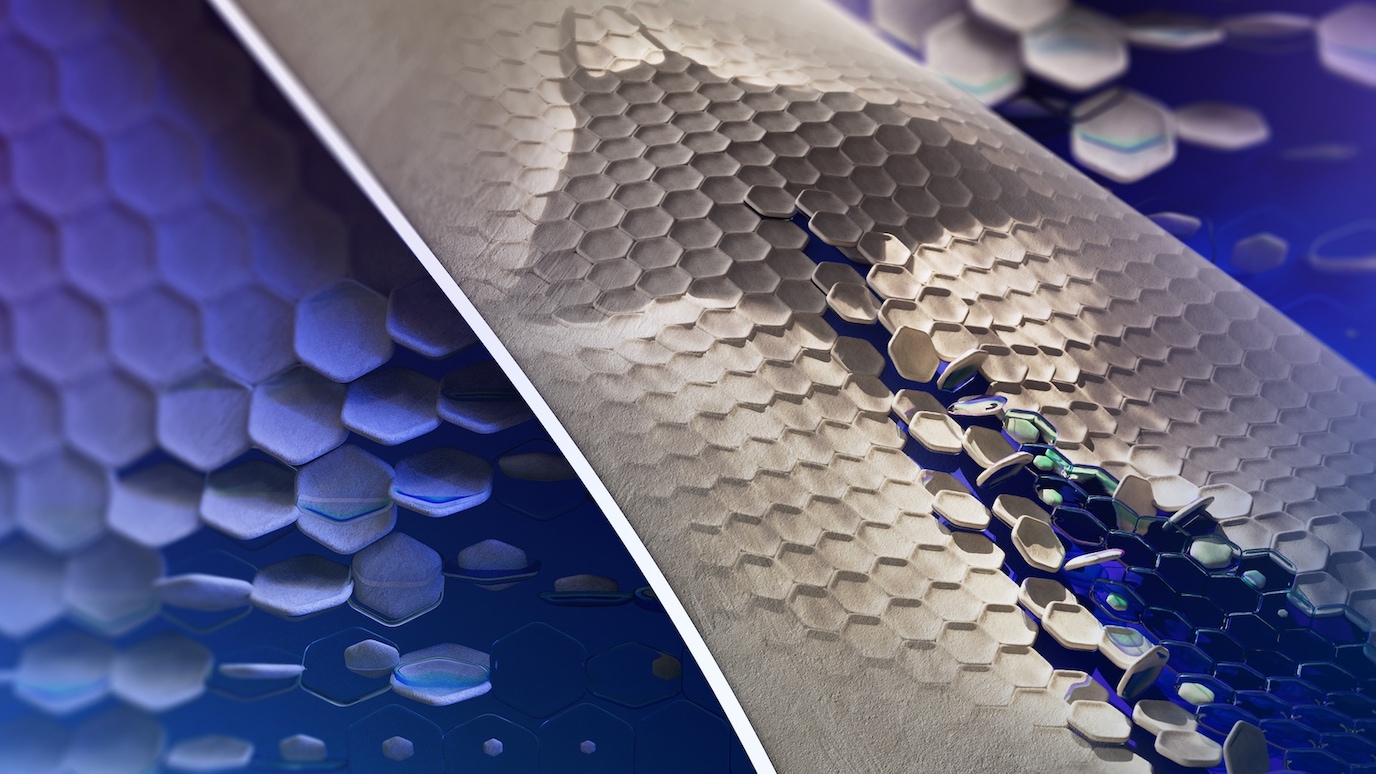
25 Jun 2025
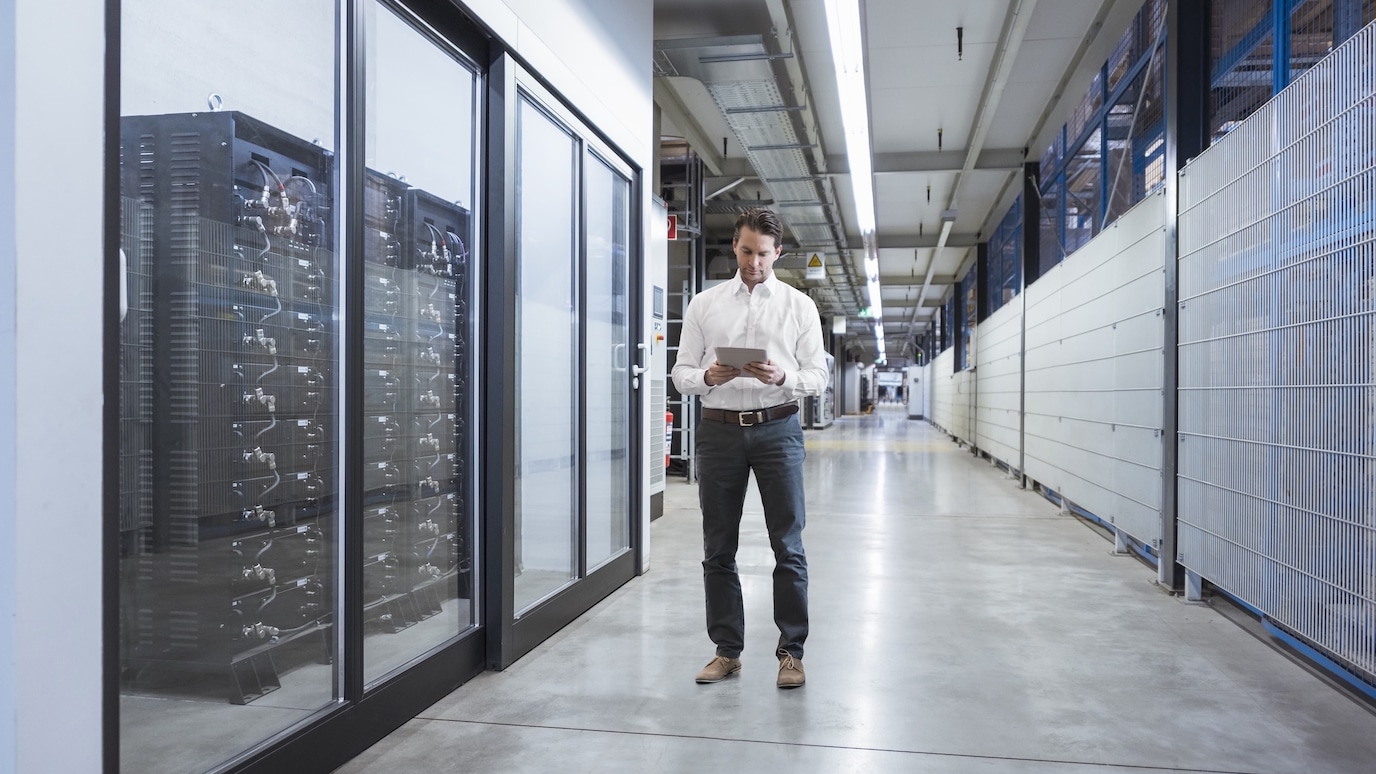
23 Jun 2025
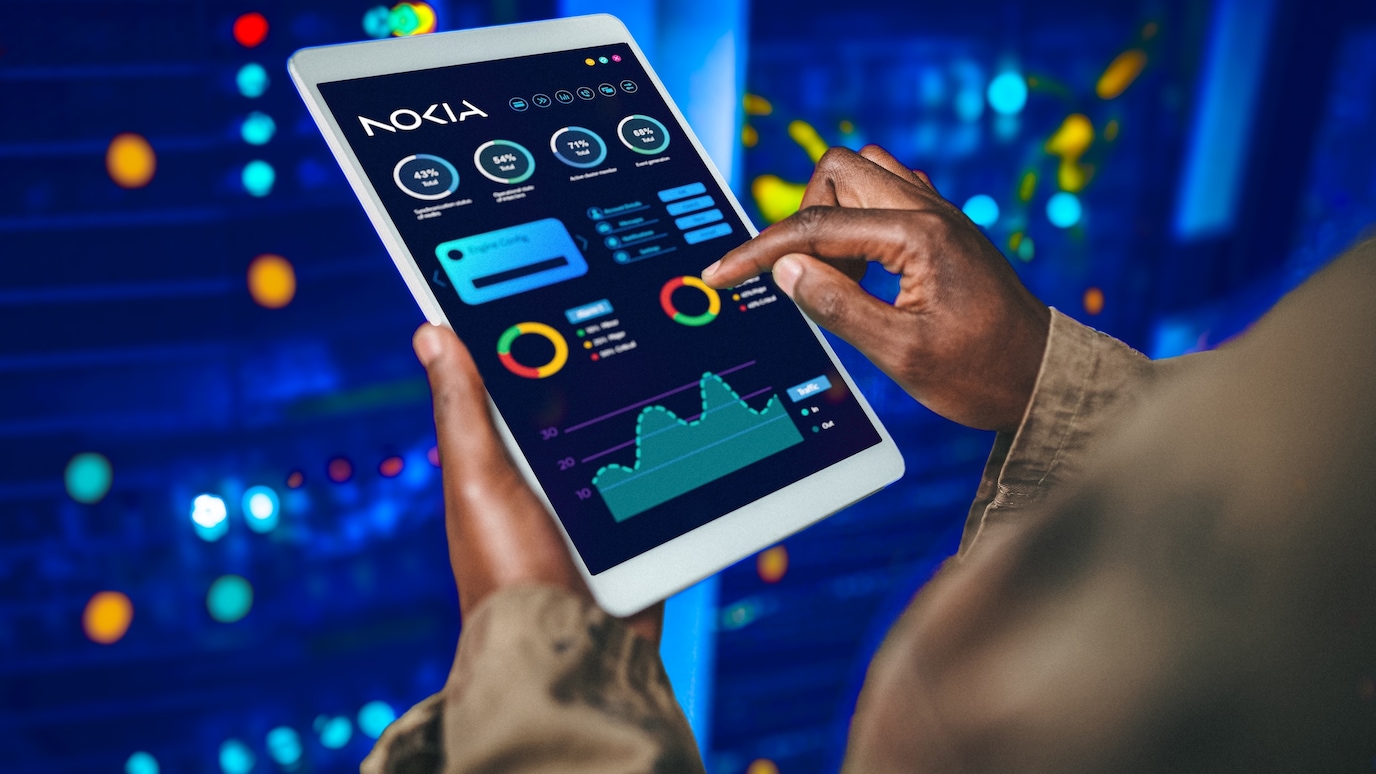
20 Jun 2025
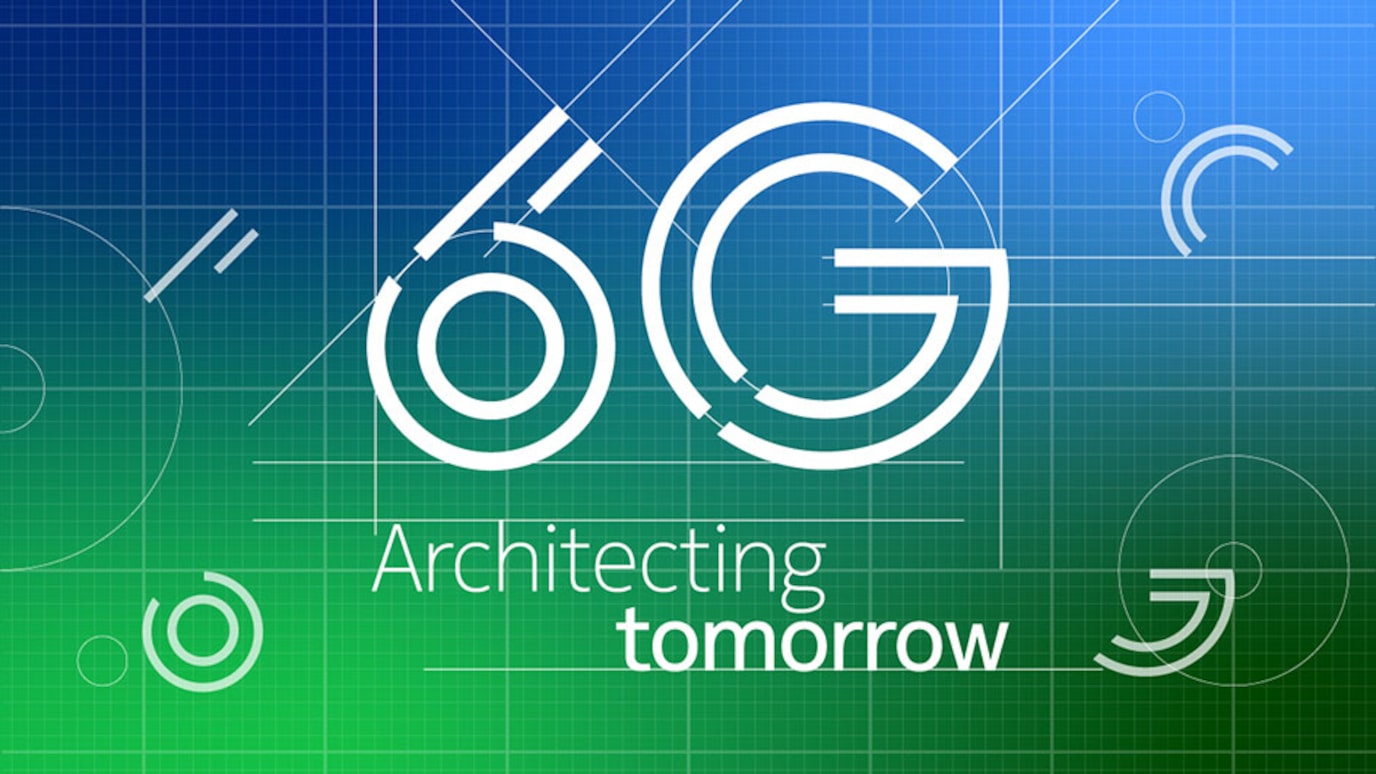
13 Jun 2025
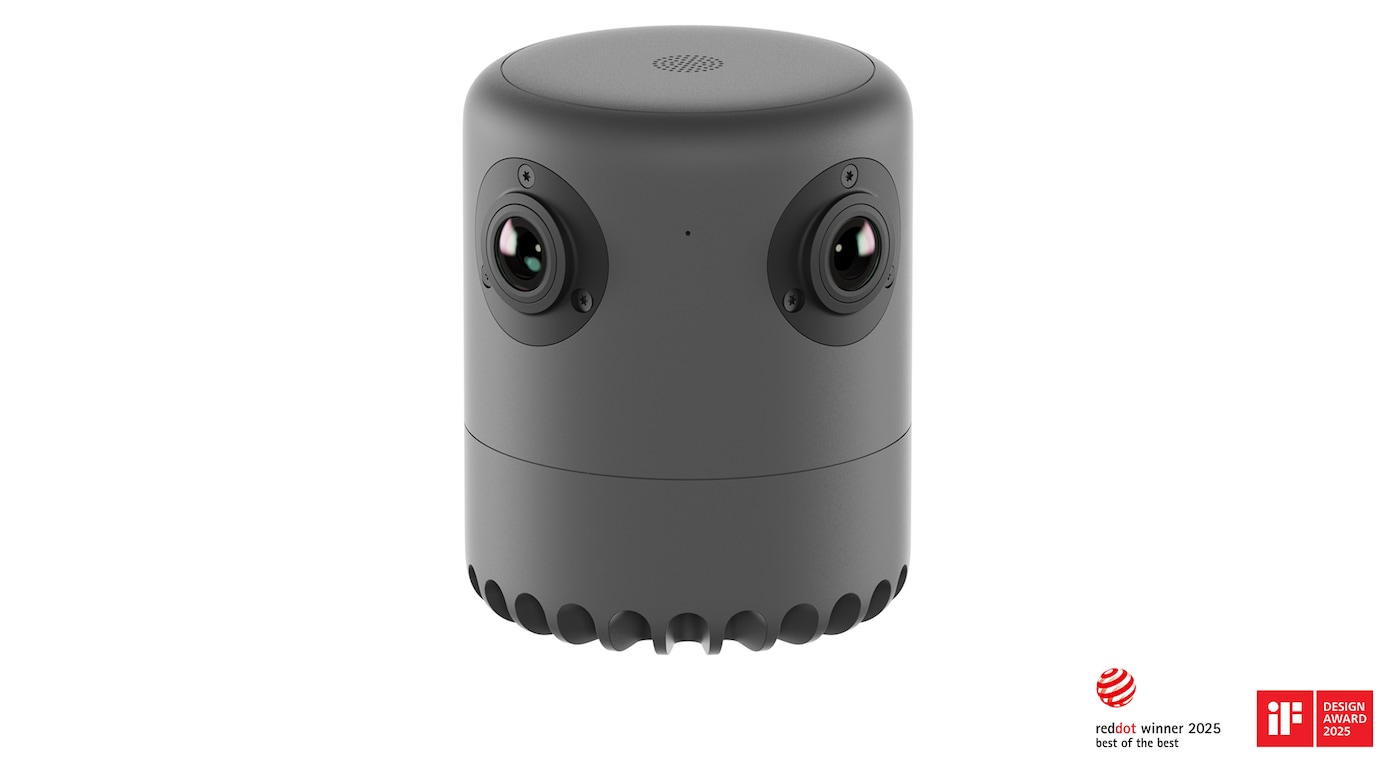
12 Jun 2025
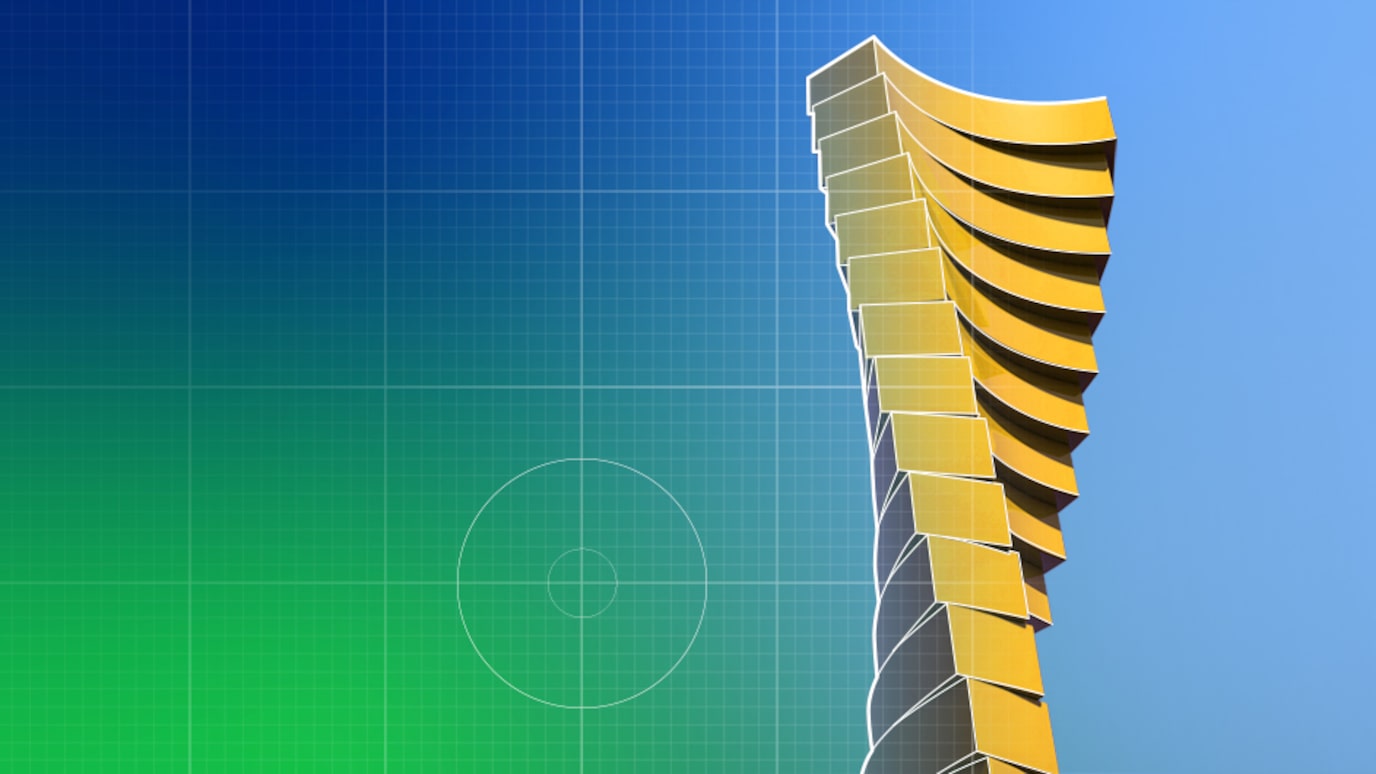
26 May 2025
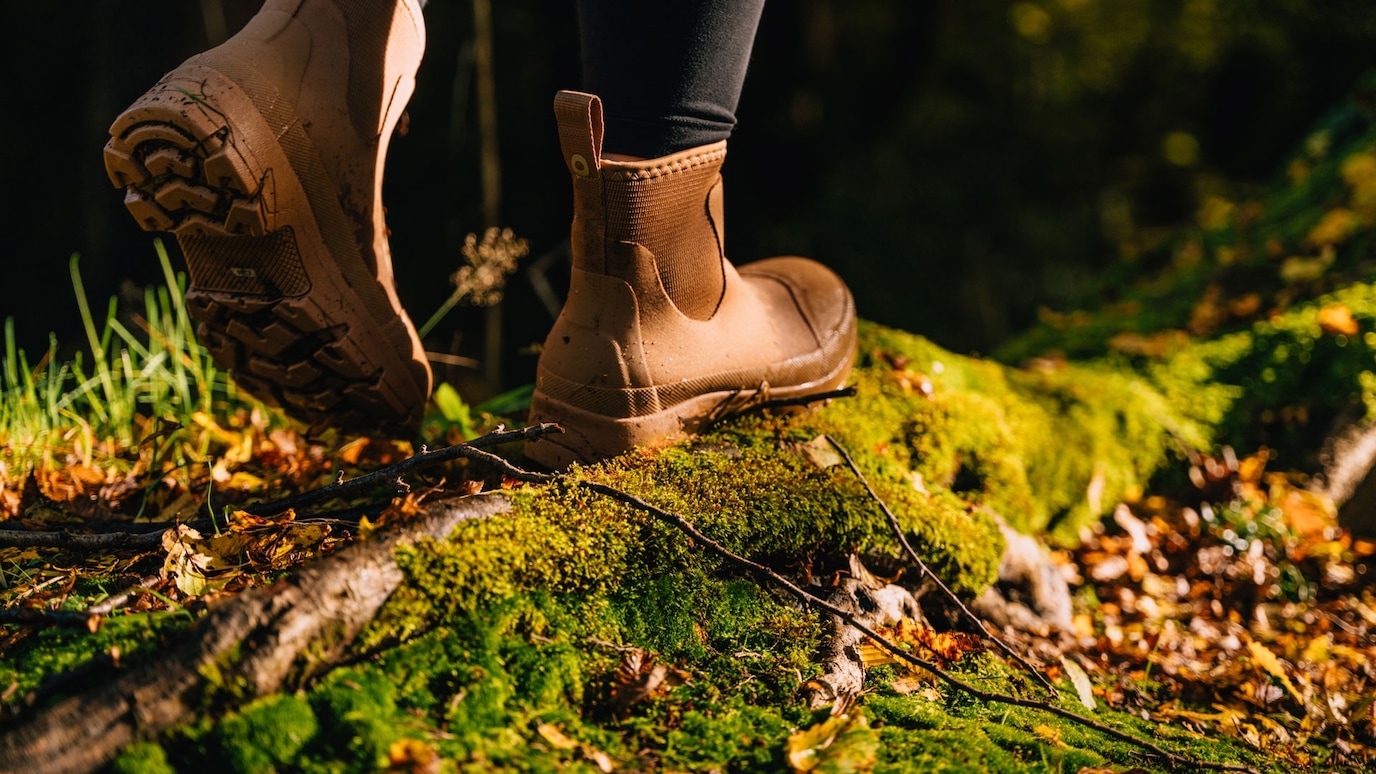
7 May 2025
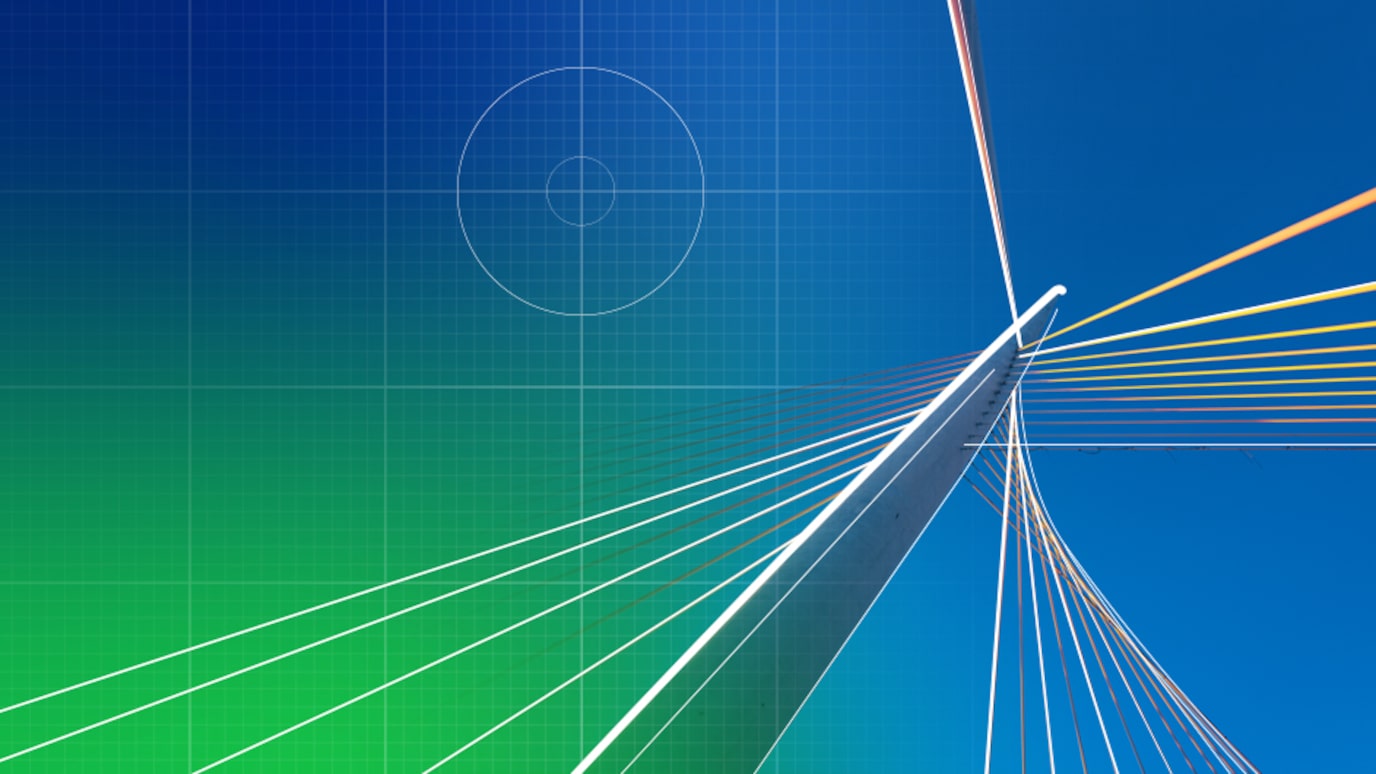
8 Apr 2025
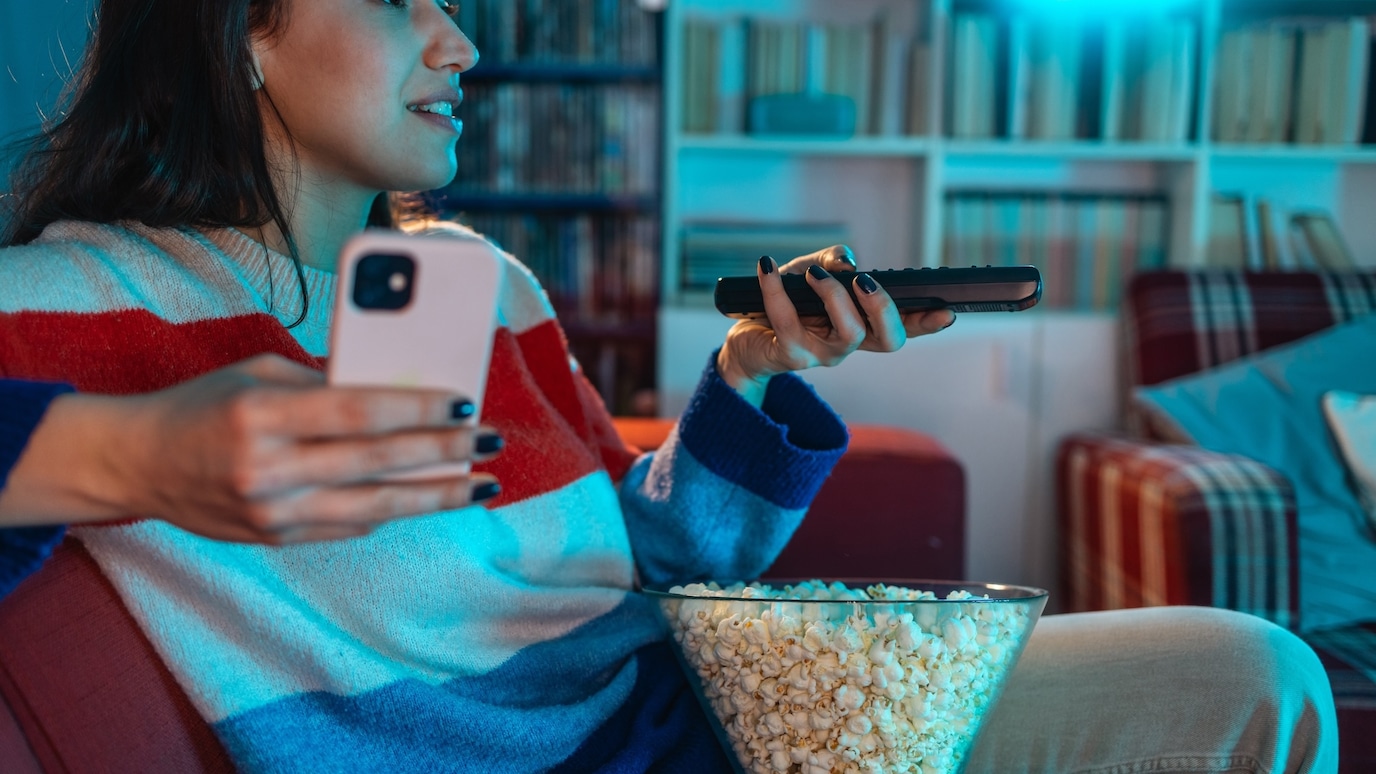
31 Mar 2025
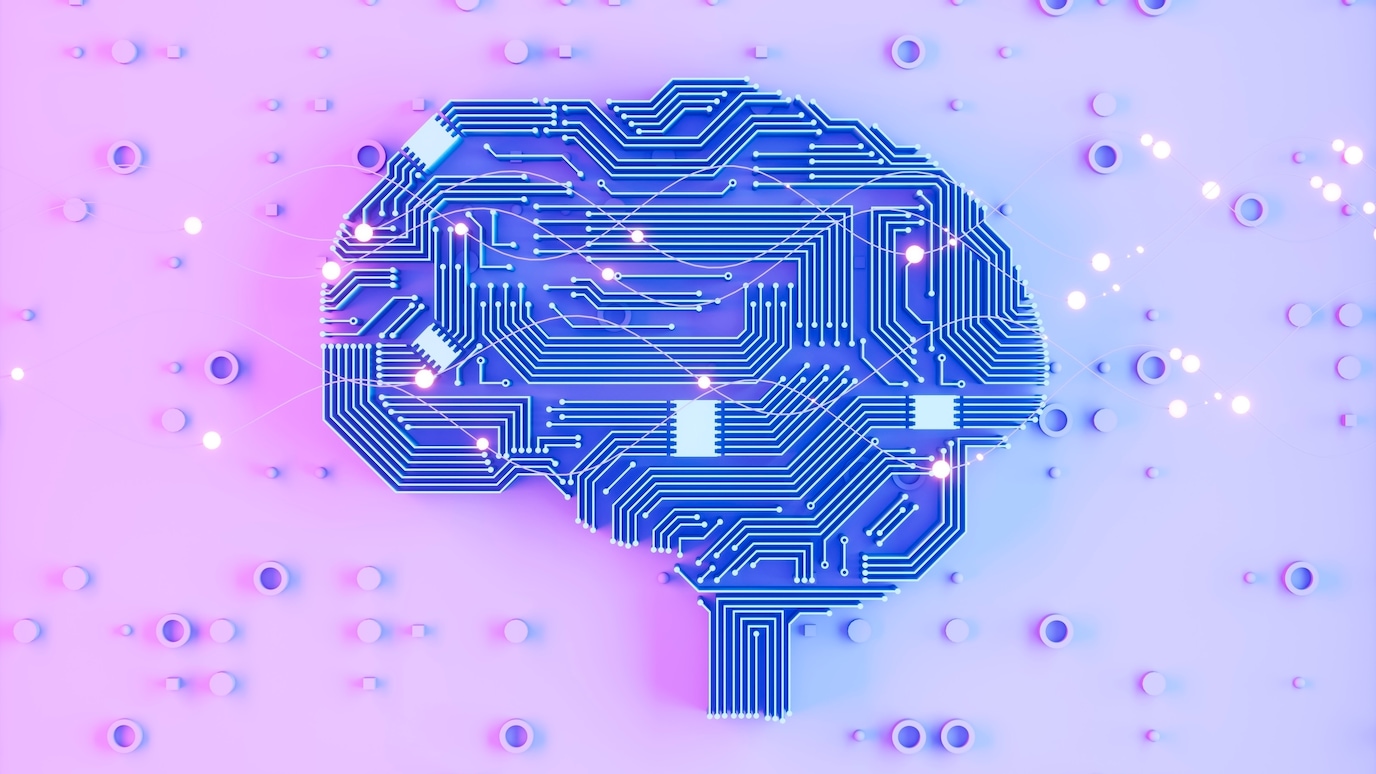
26 Mar 2025
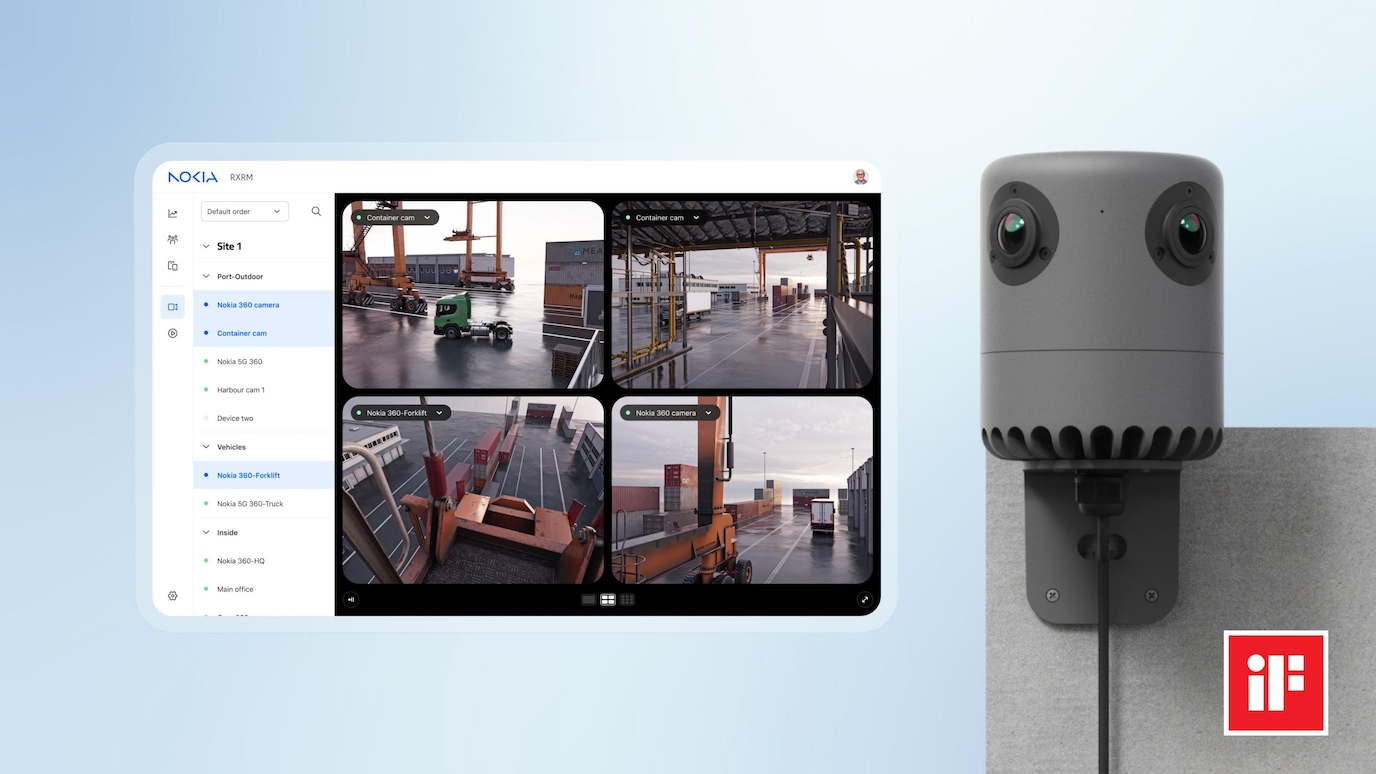
12 Mar 2025
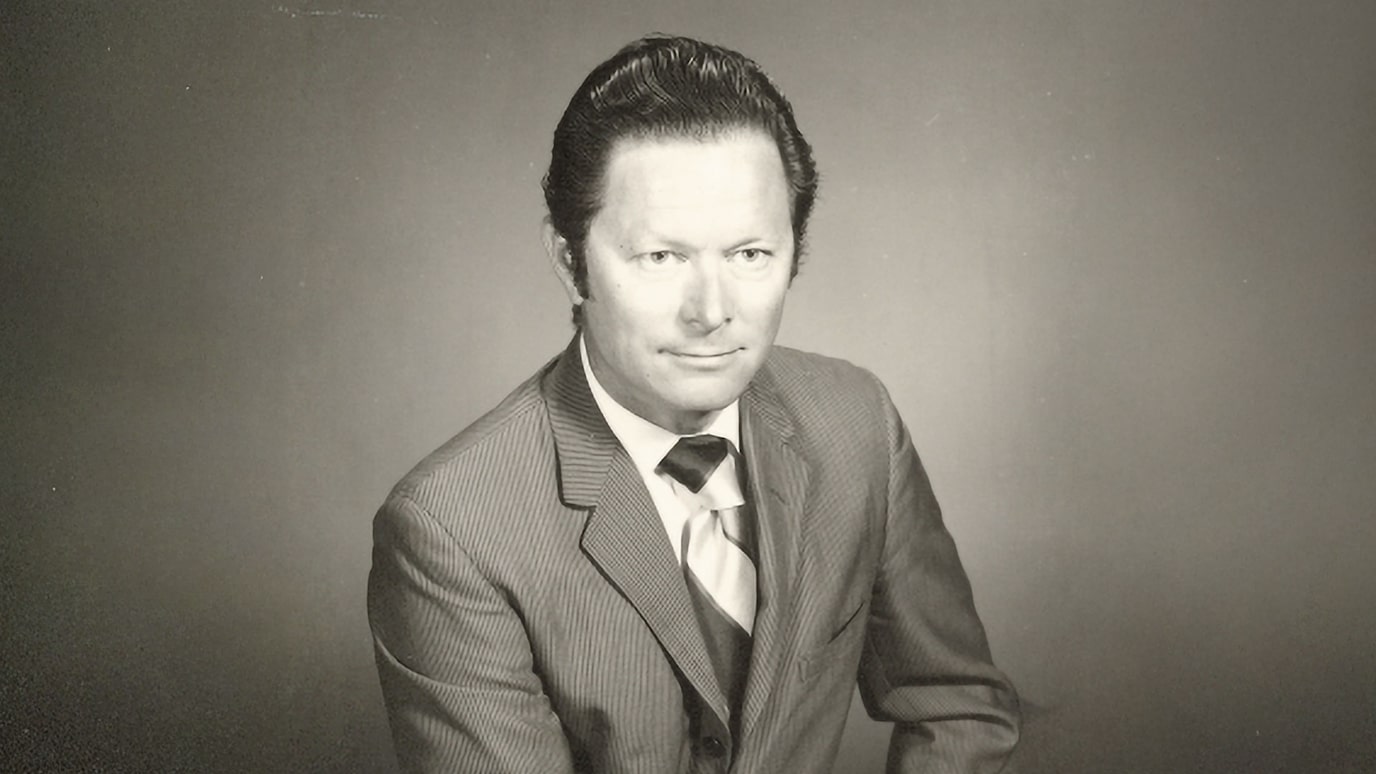
9 Jun 2025
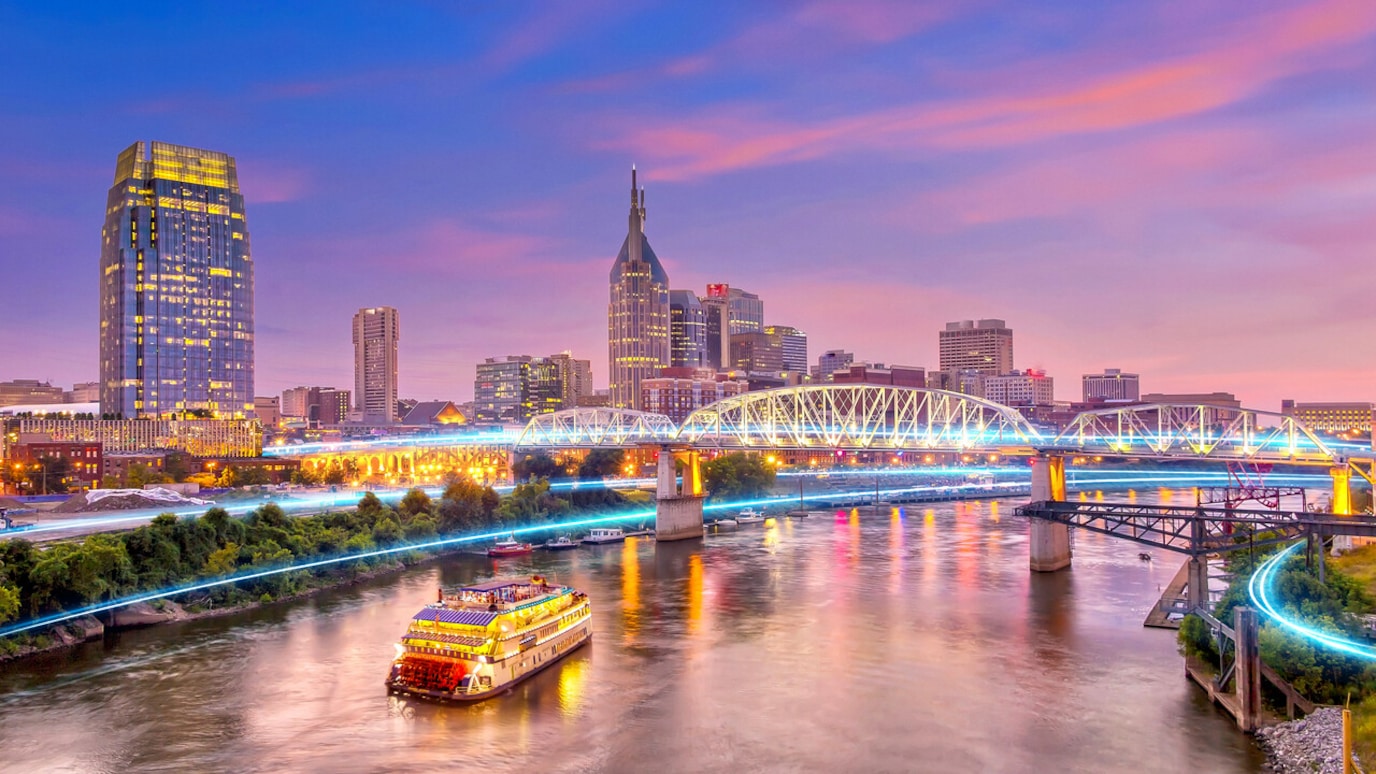
5 Jun 2025
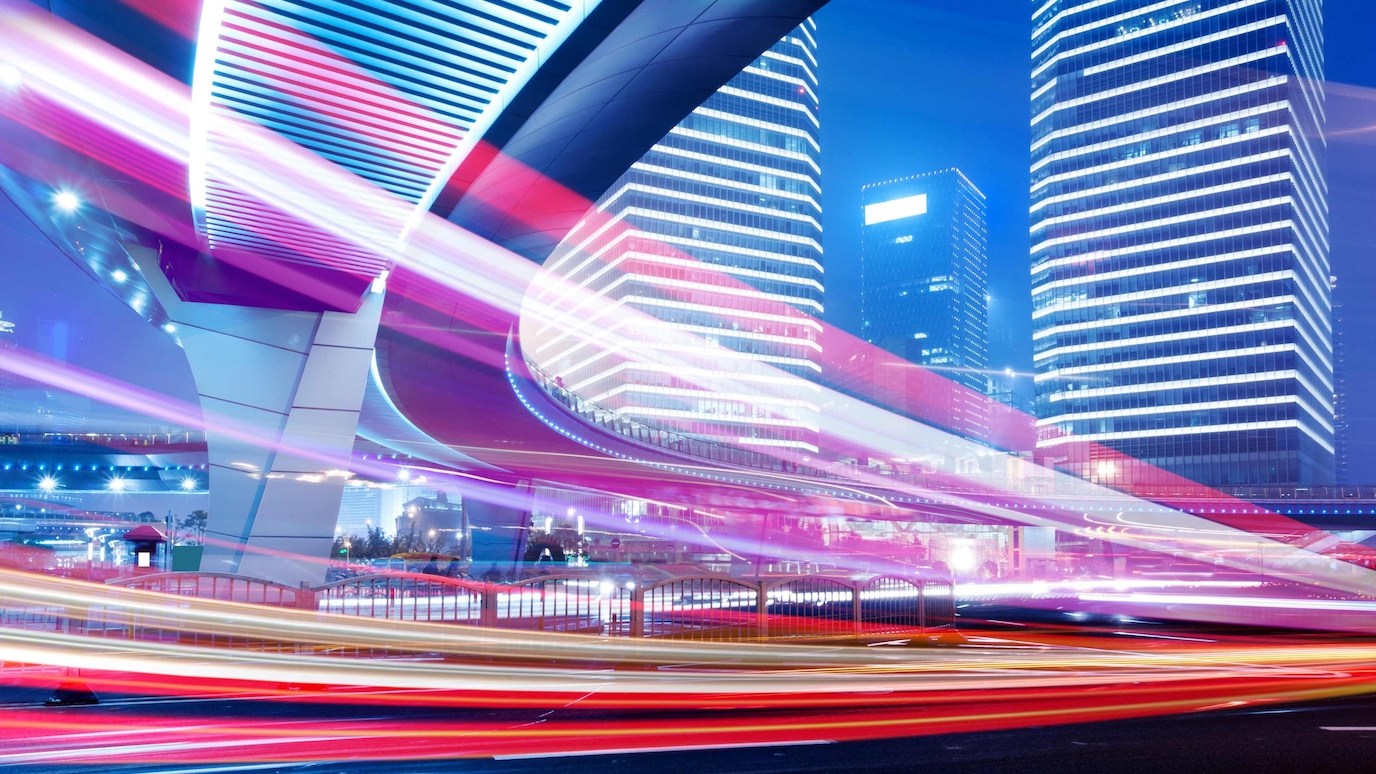
27 Mar 2025
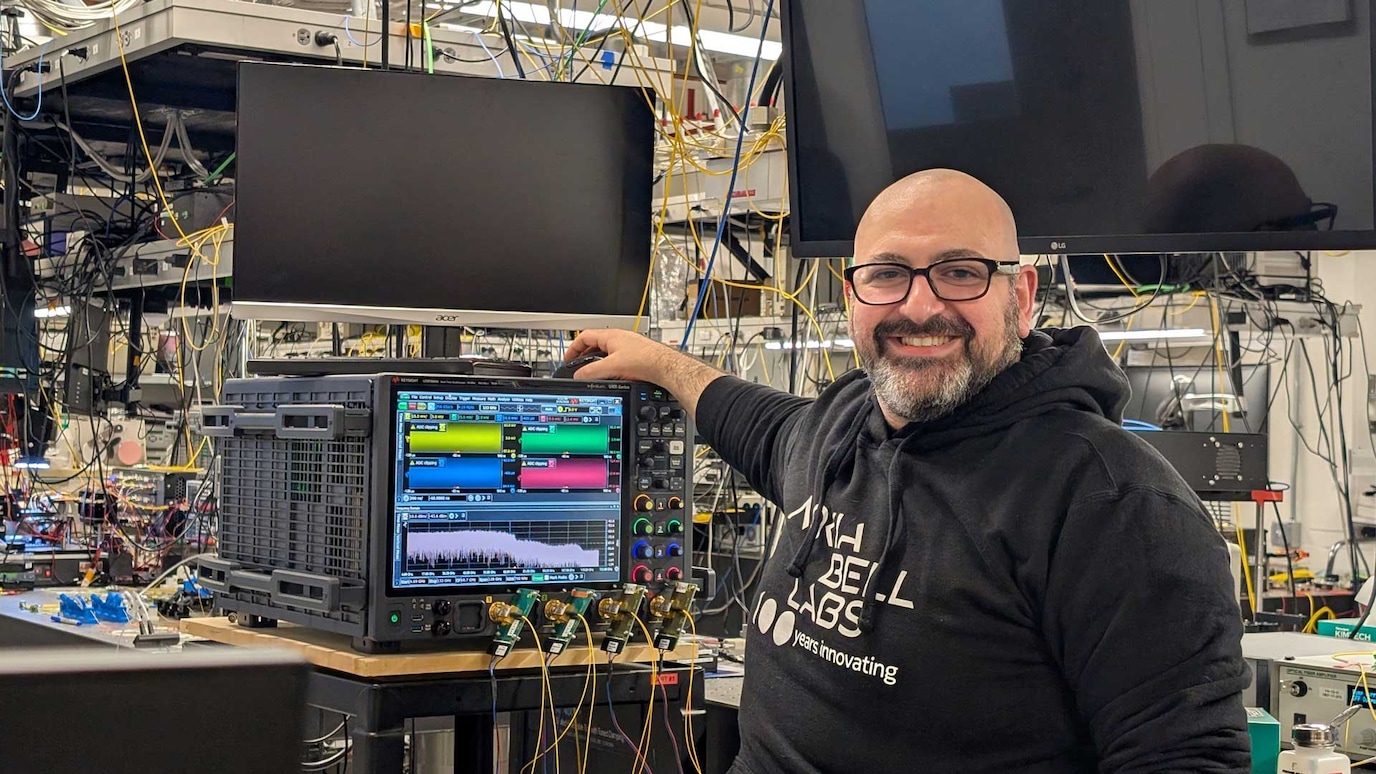
25 Mar 2025
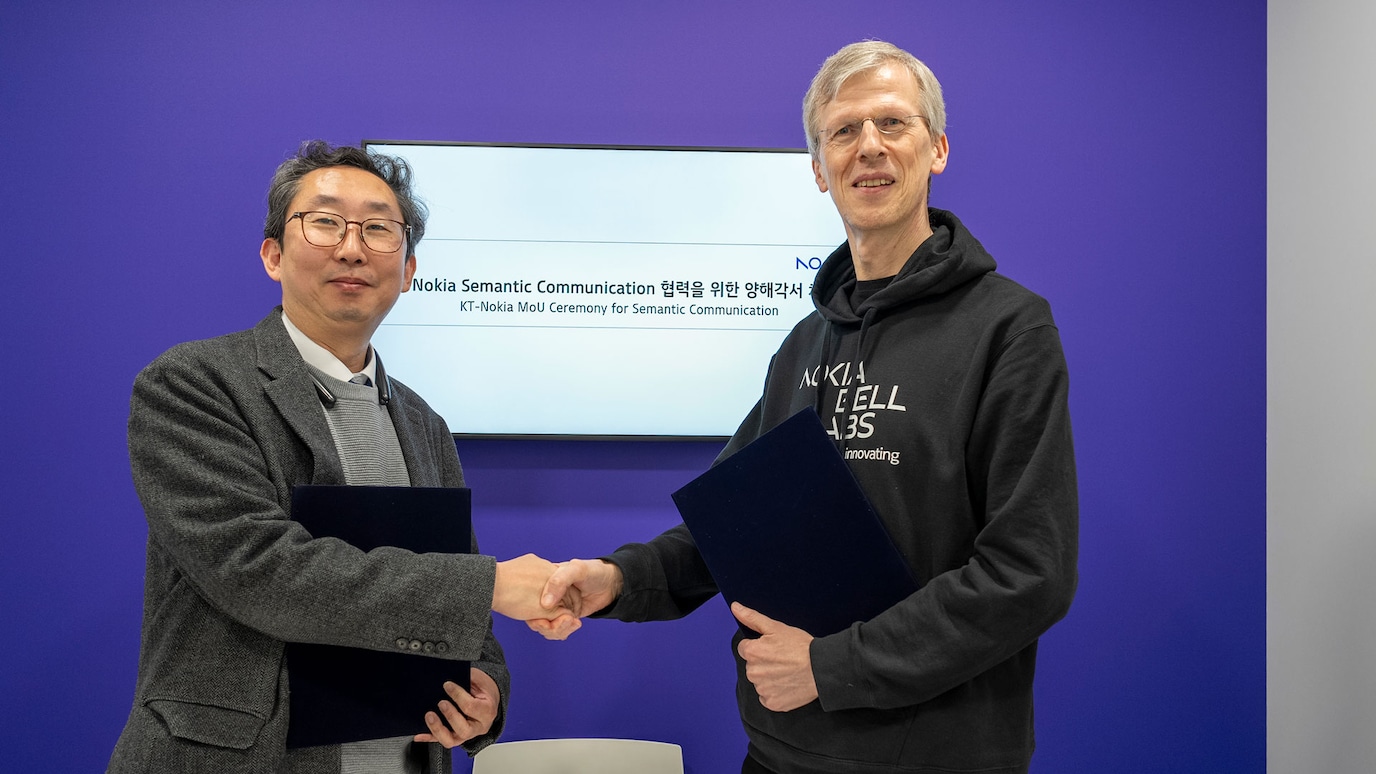
3 Mar 2025
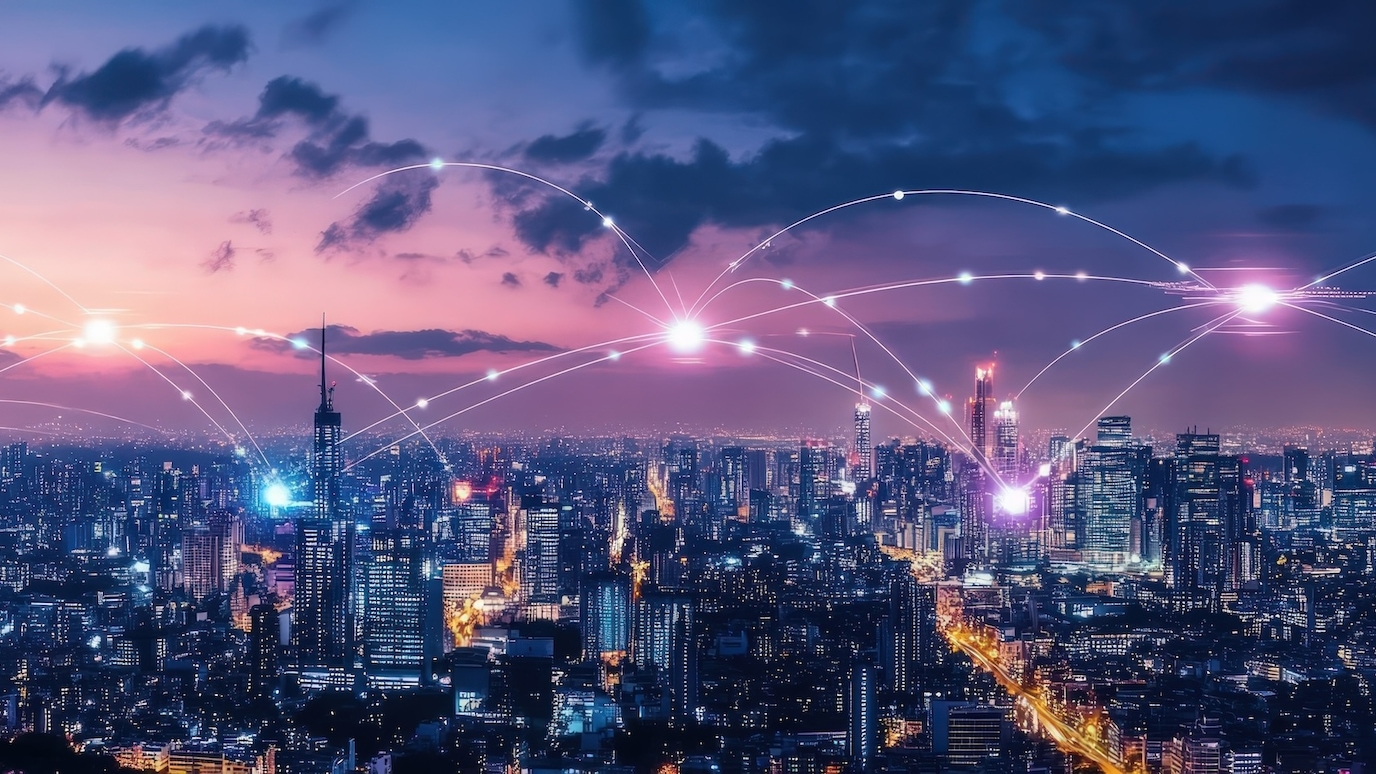
26 Feb 2025
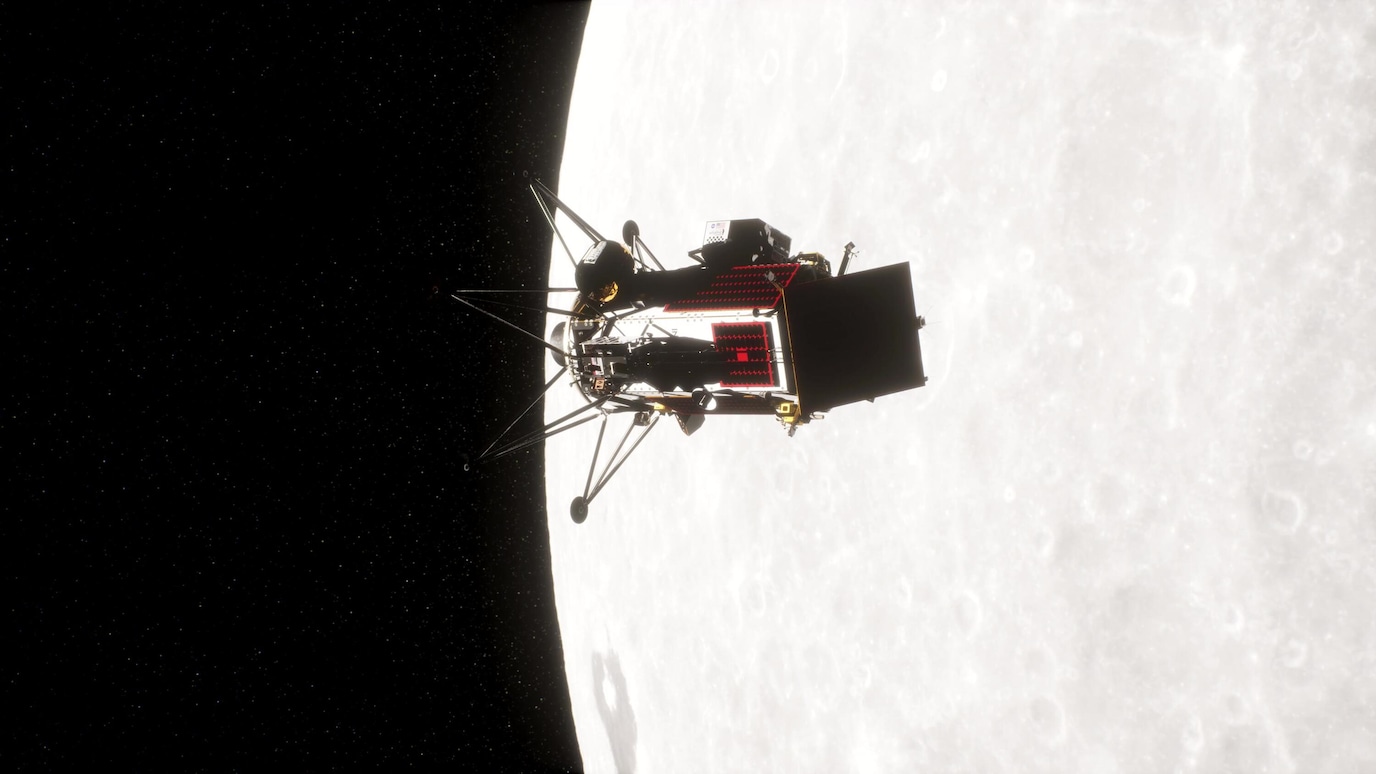
21 Feb 2025
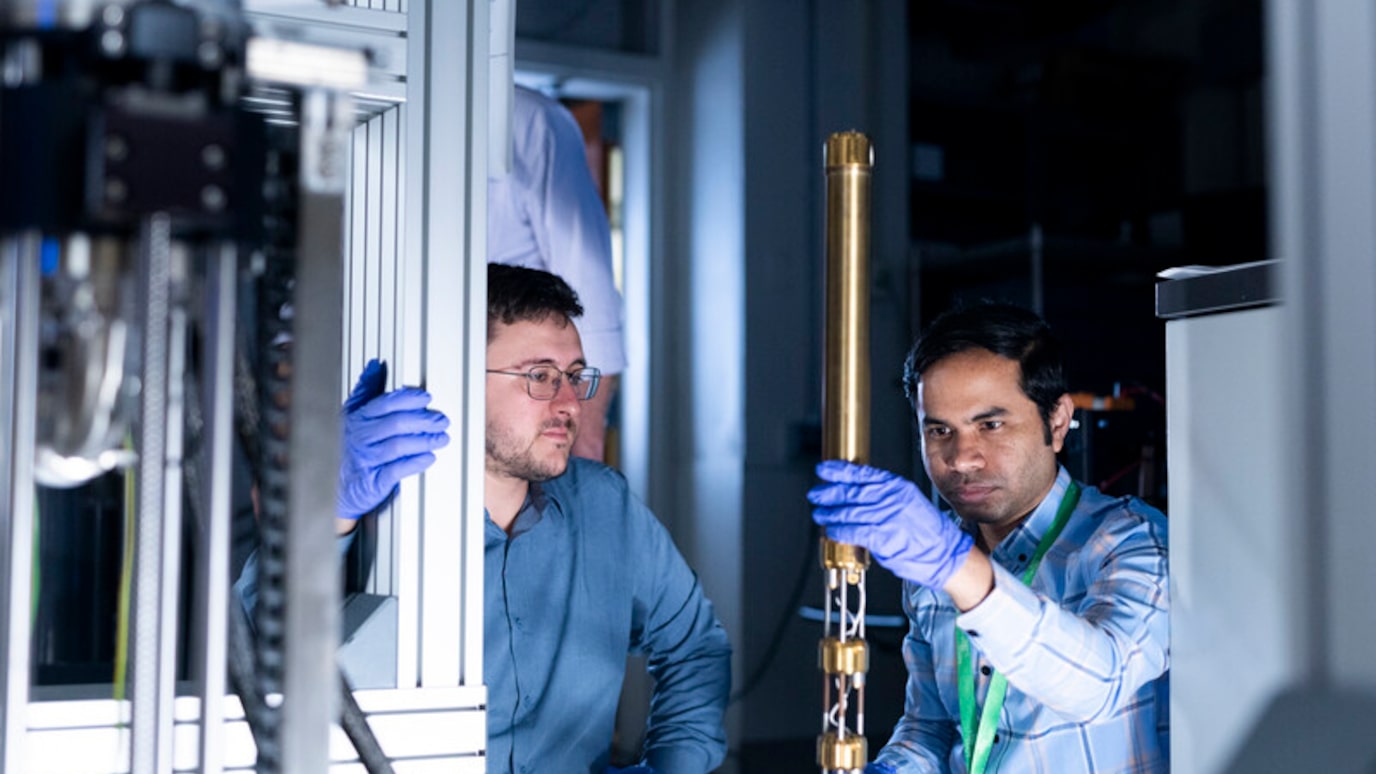
4 Feb 2025
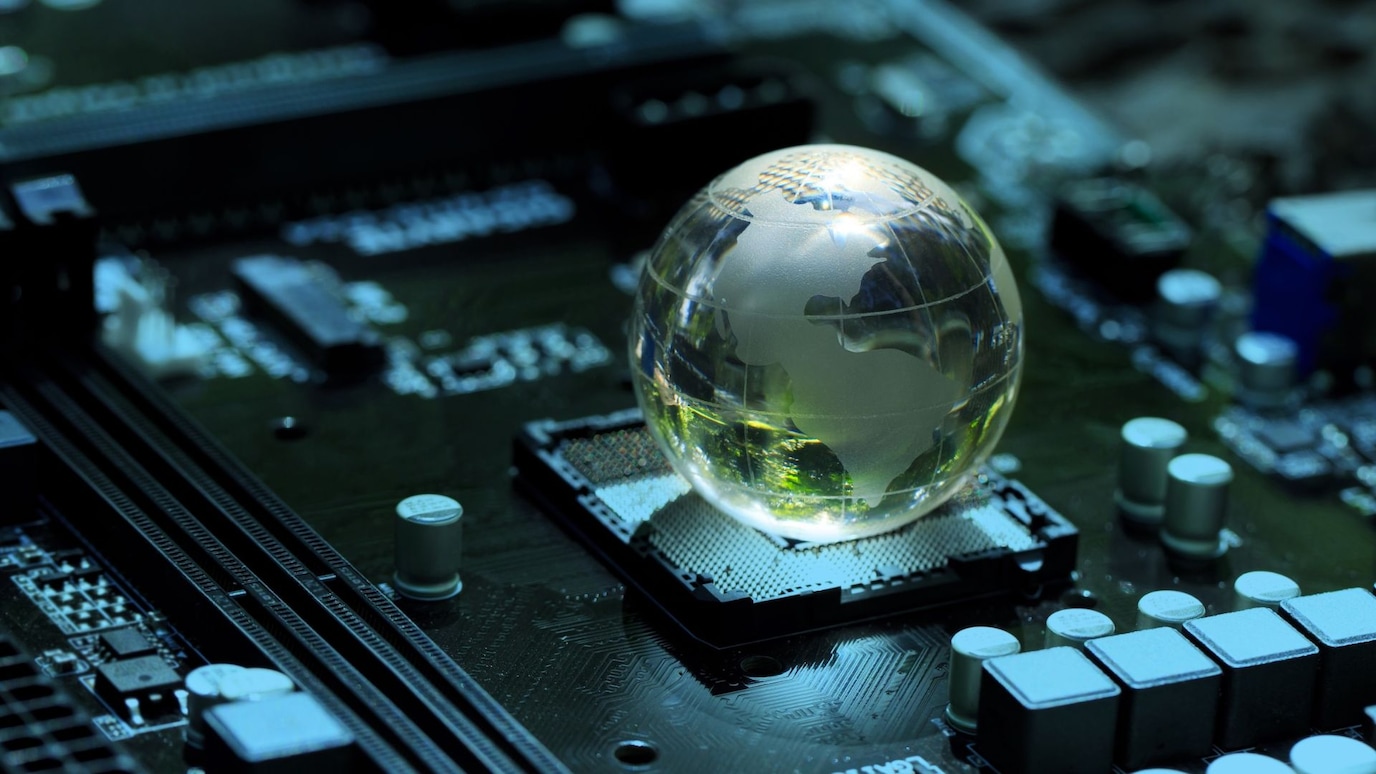
27 May 2025
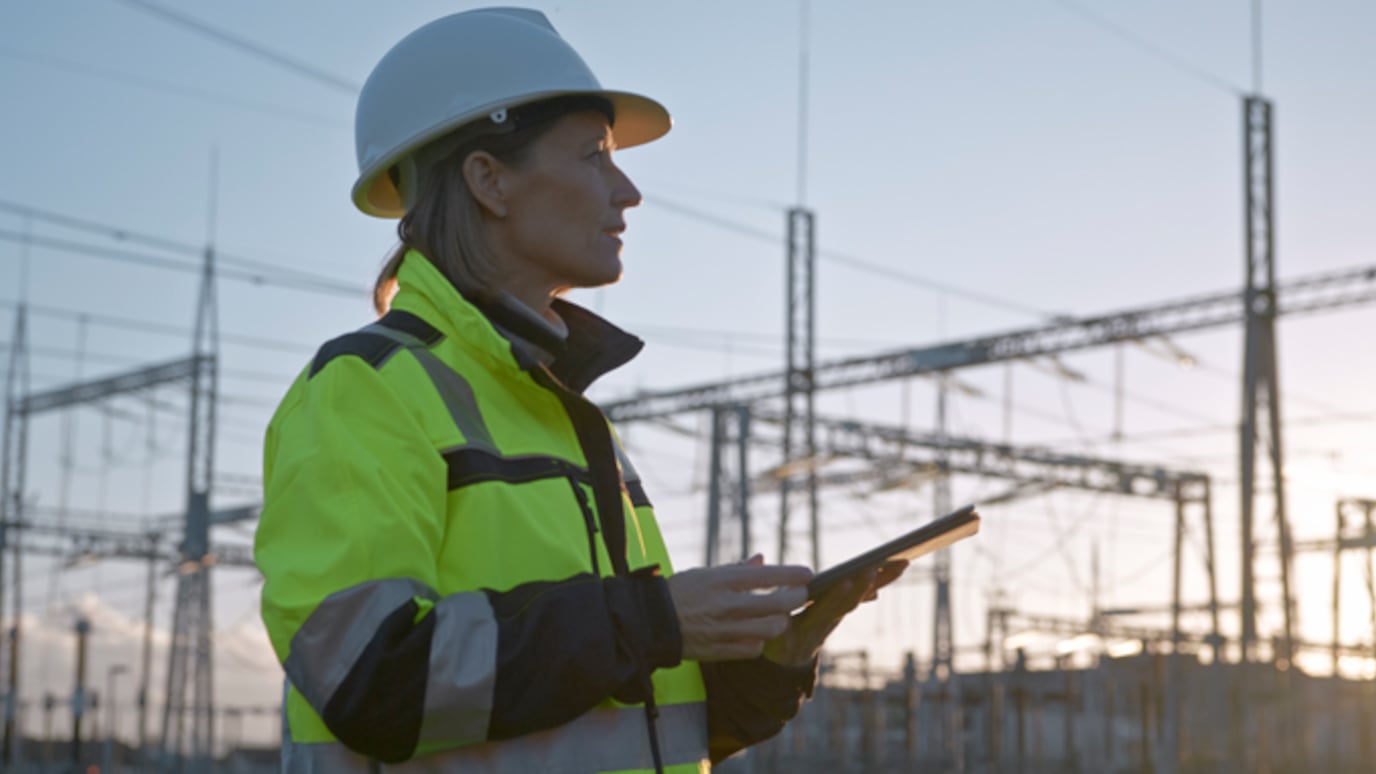
6 May 2025
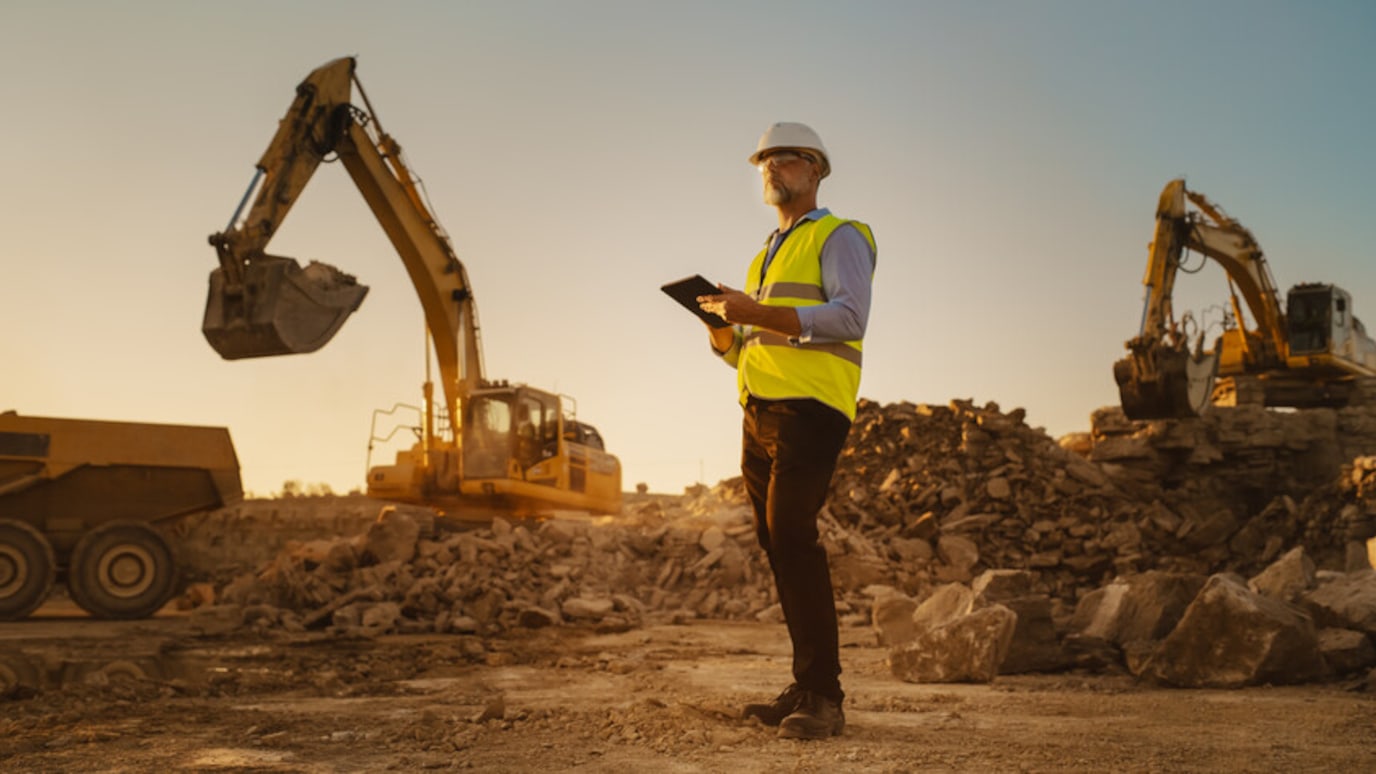
23 Apr 2025
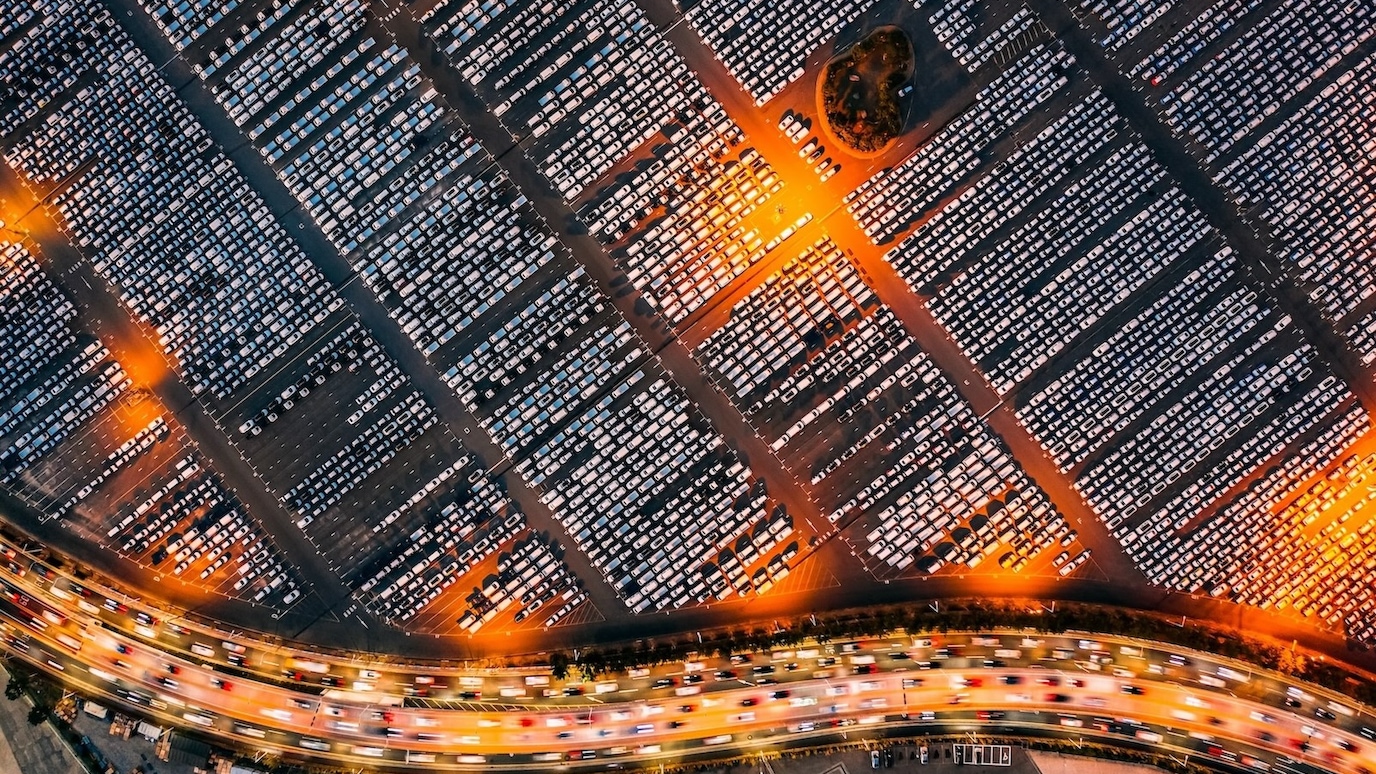
28 Mar 2025
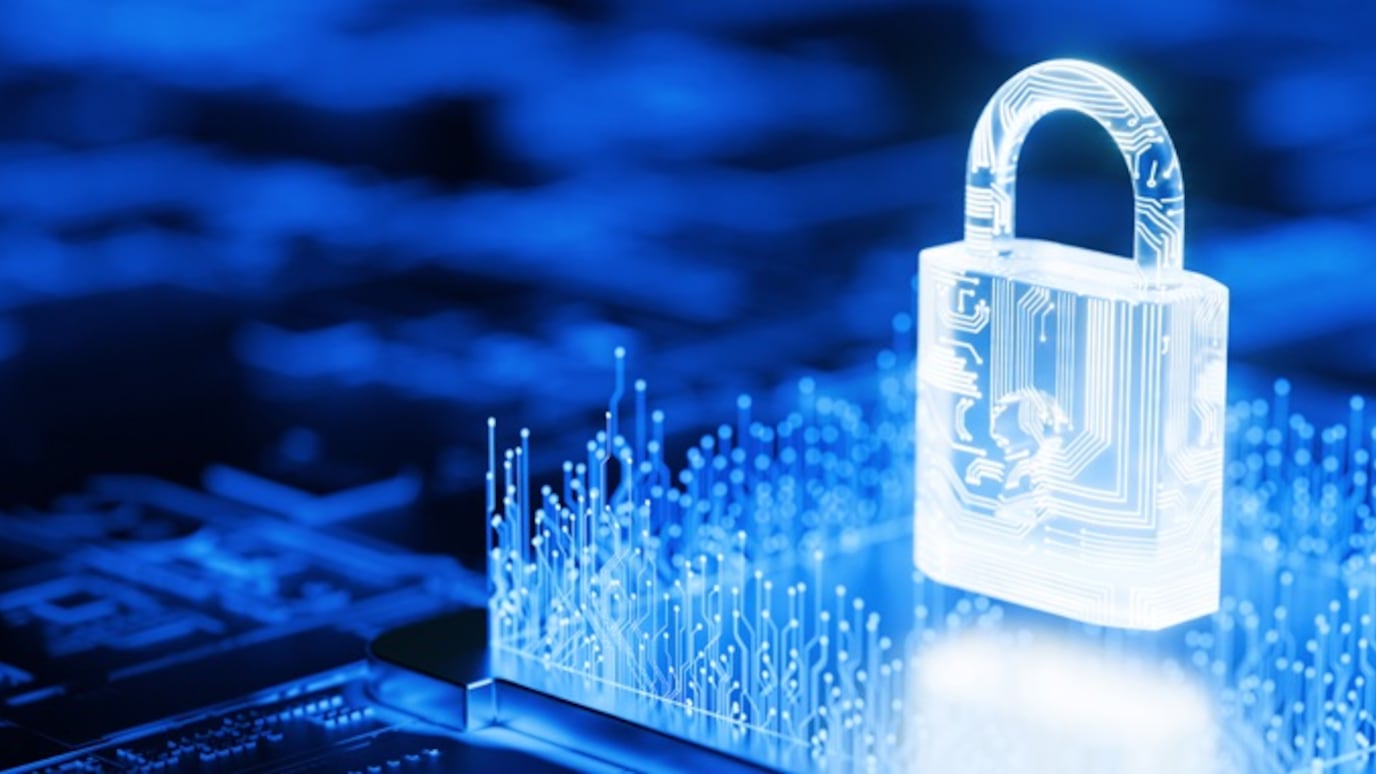
24 Mar 2025
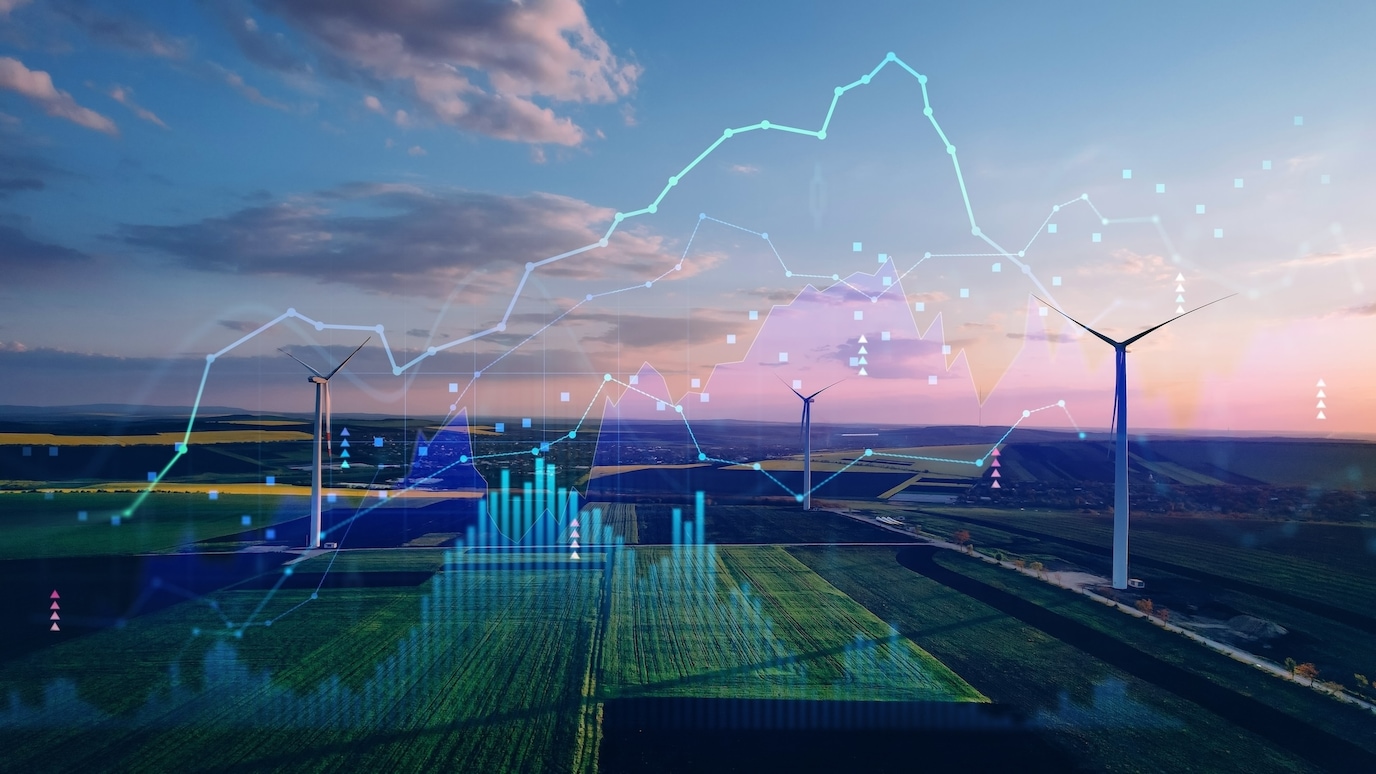
20 Mar 2025
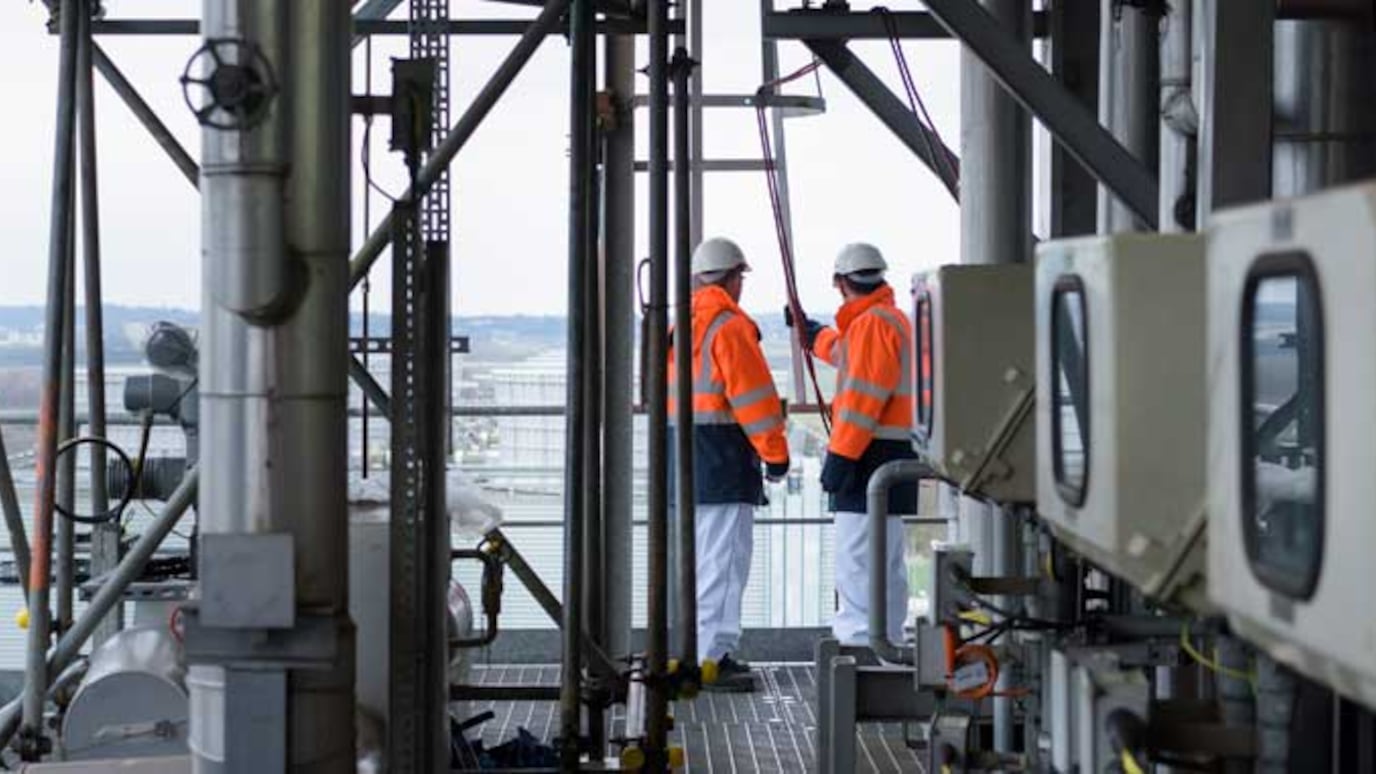
19 Mar 2025
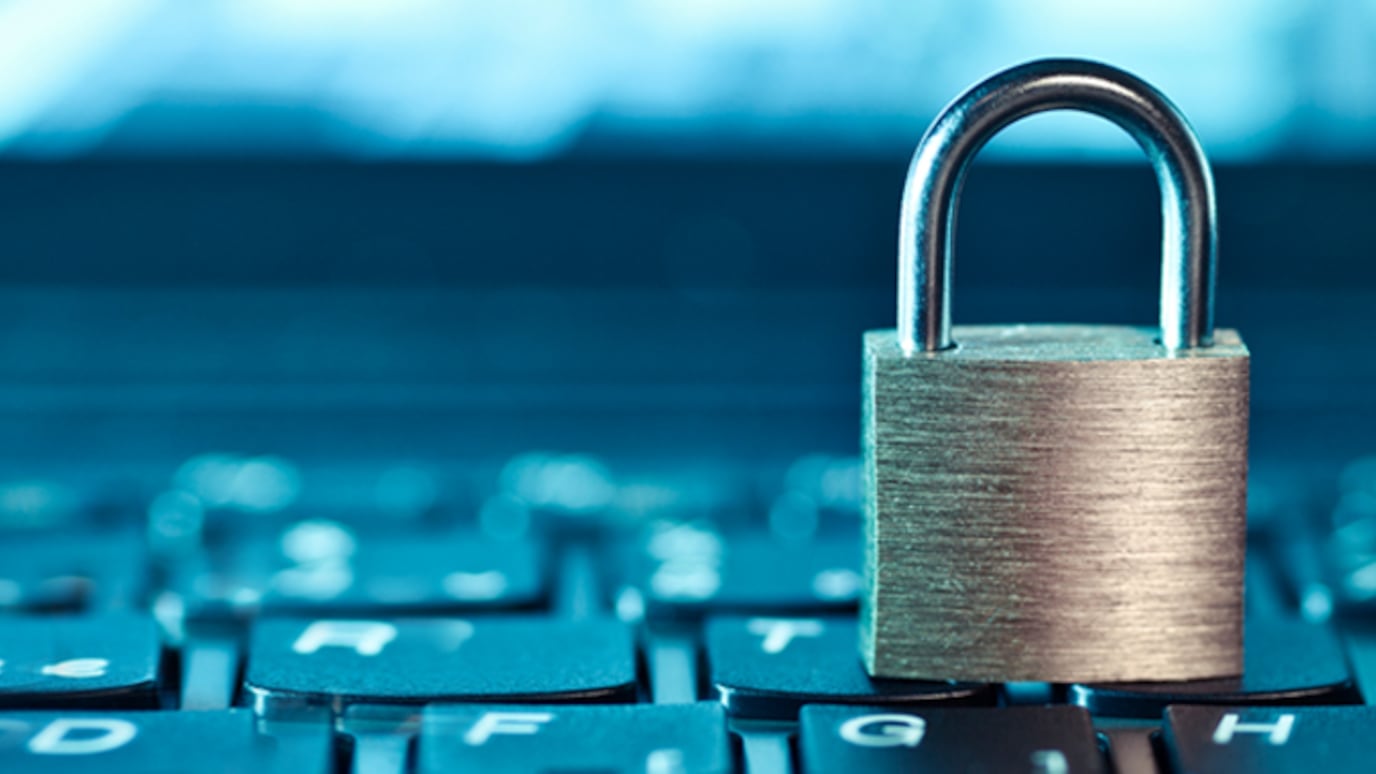
5 Mar 2025
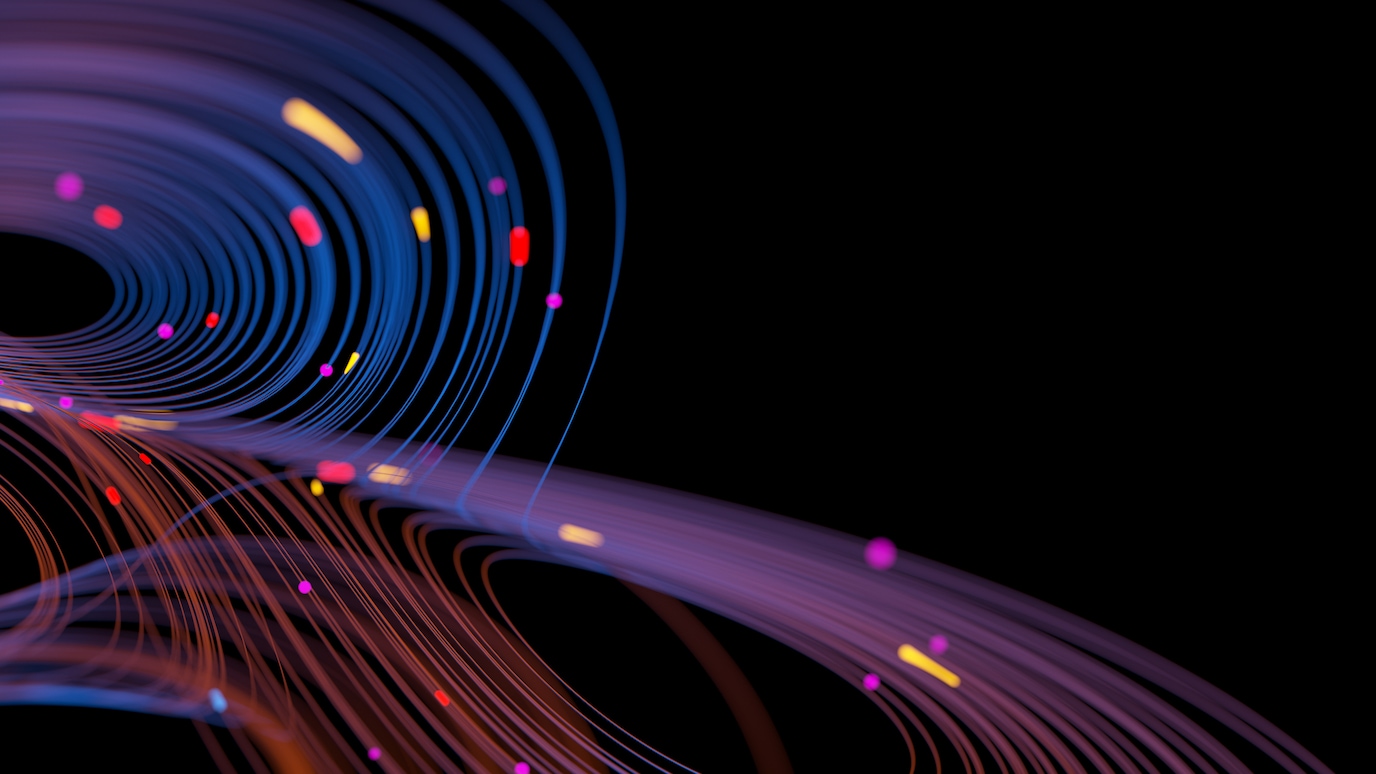
28 Apr 2025
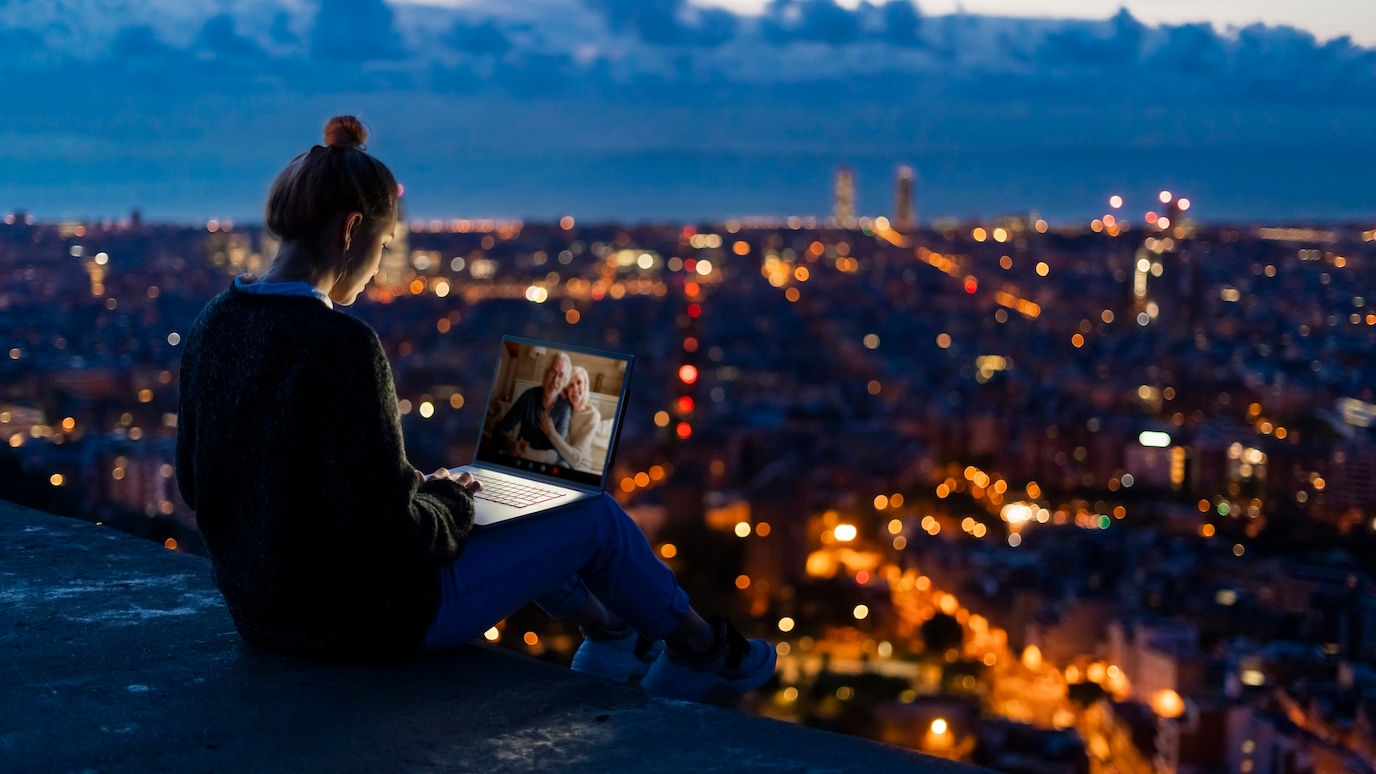
1 Apr 2025
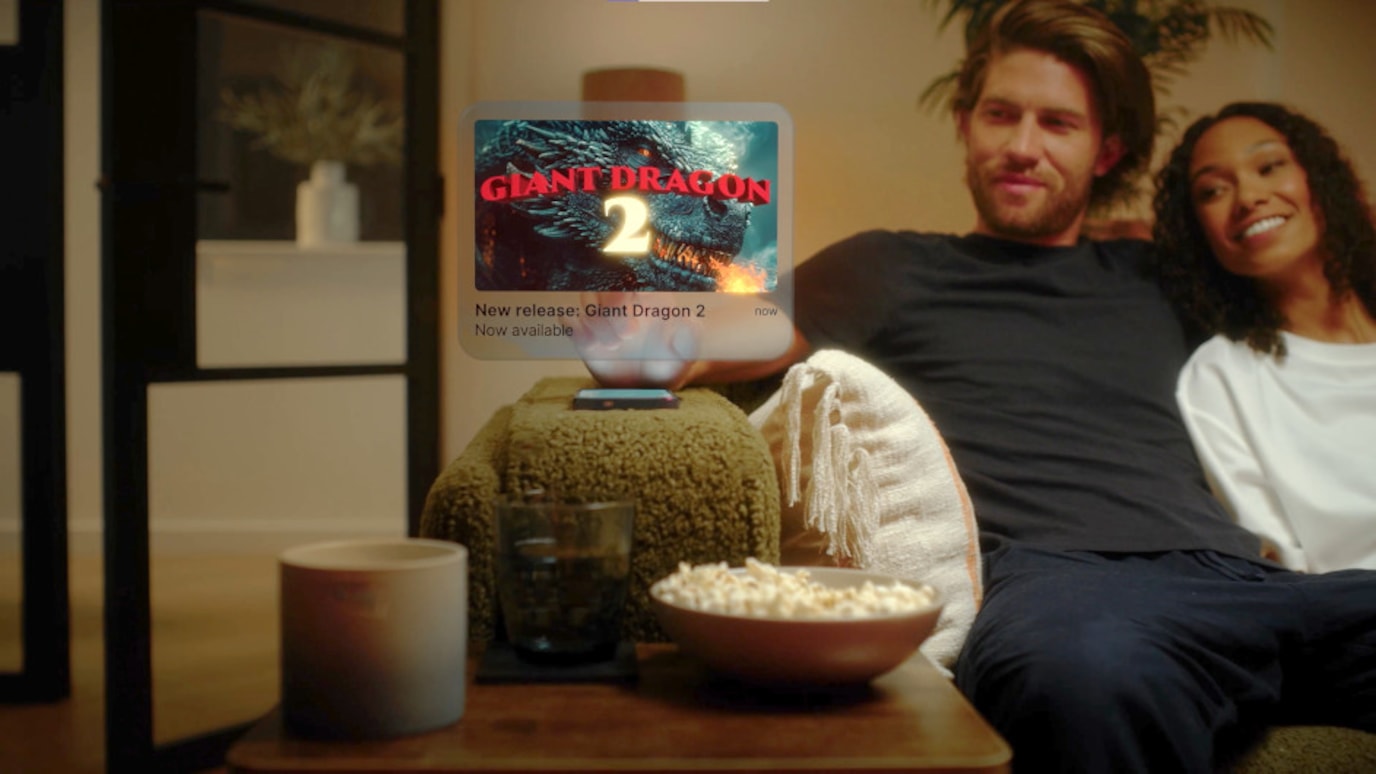
26 Feb 2025
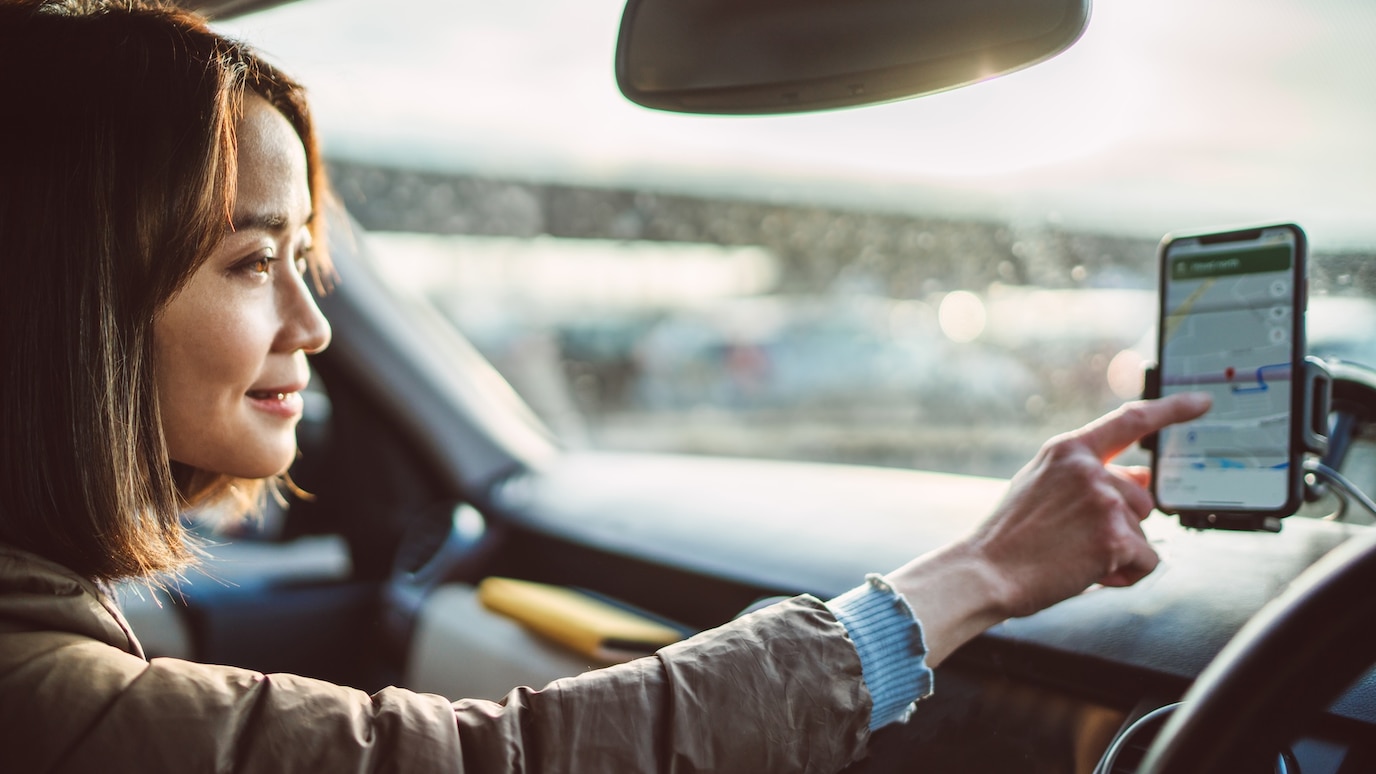
17 Dec 2024
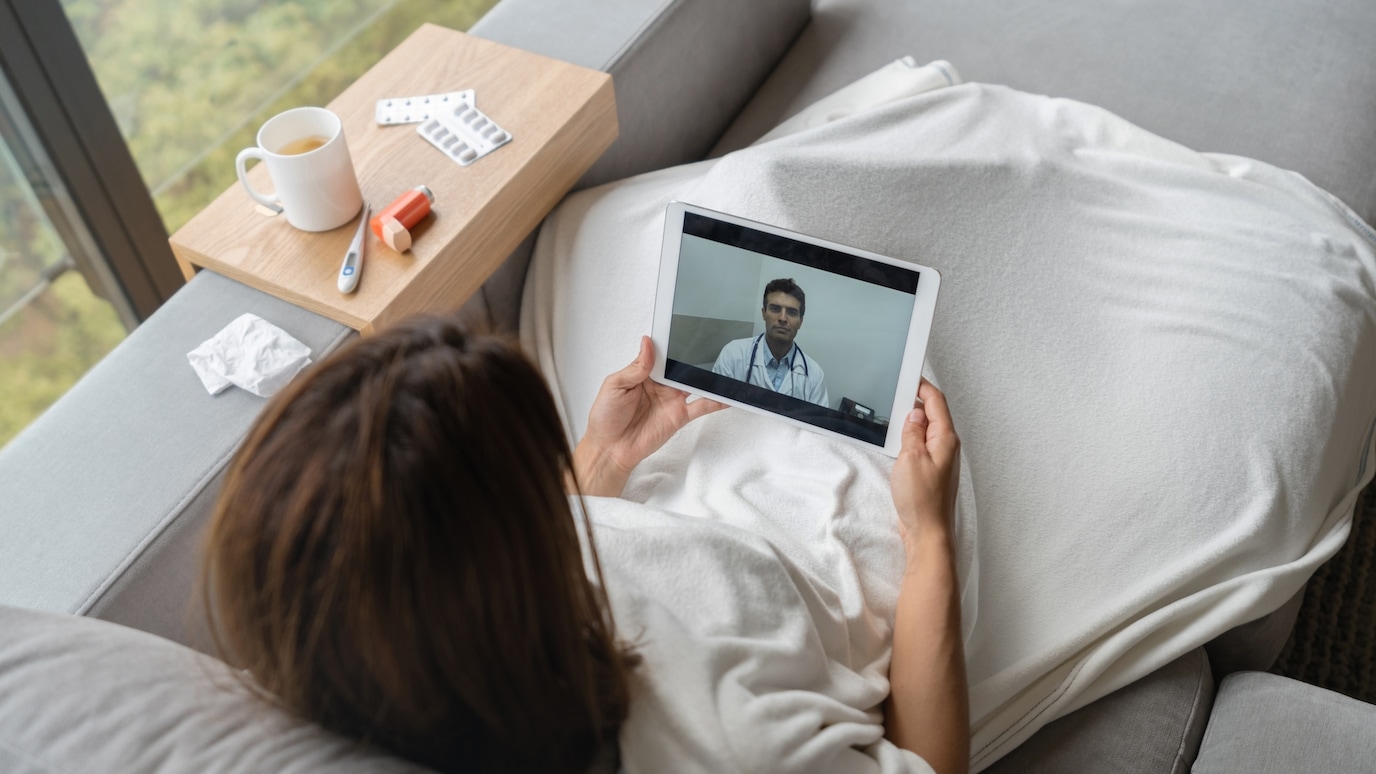
27 Sep 2024
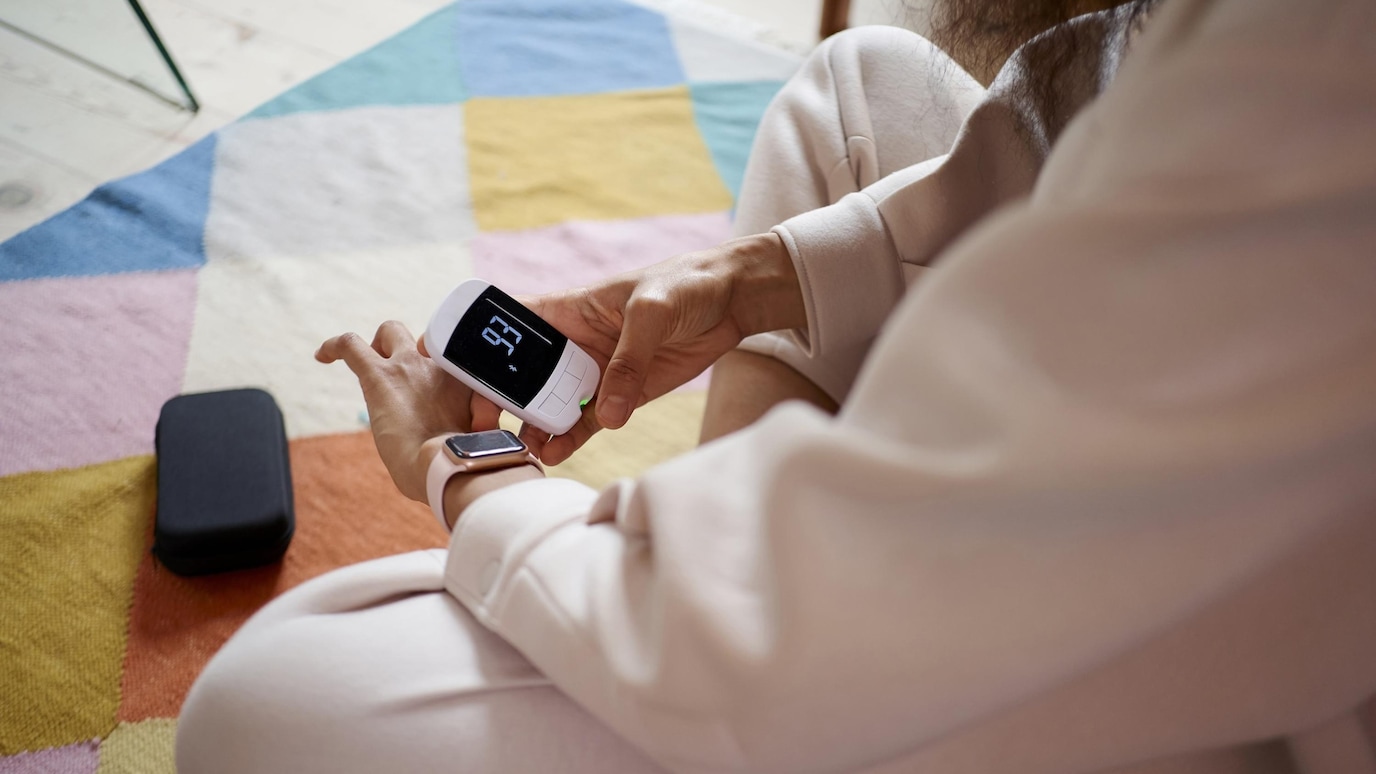
9 Sep 2024
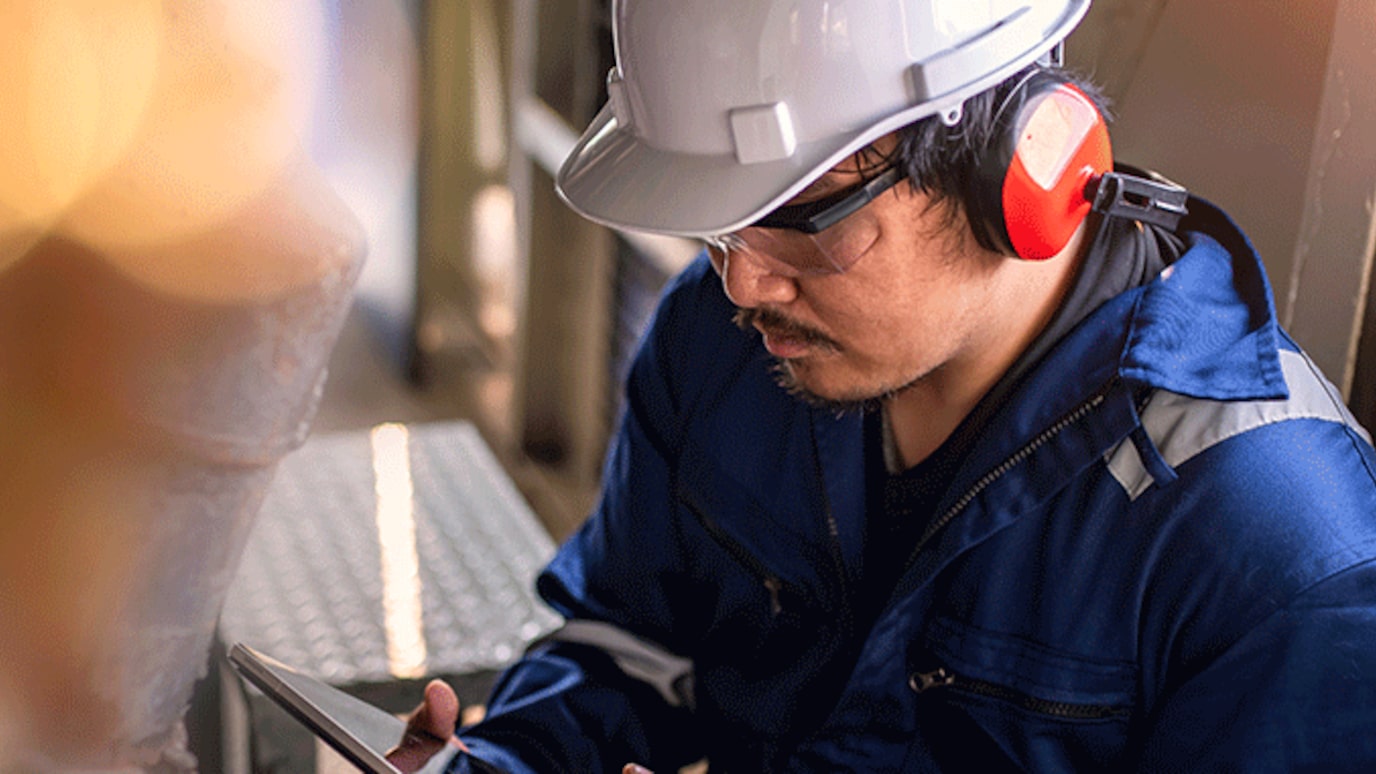
15 Aug 2024
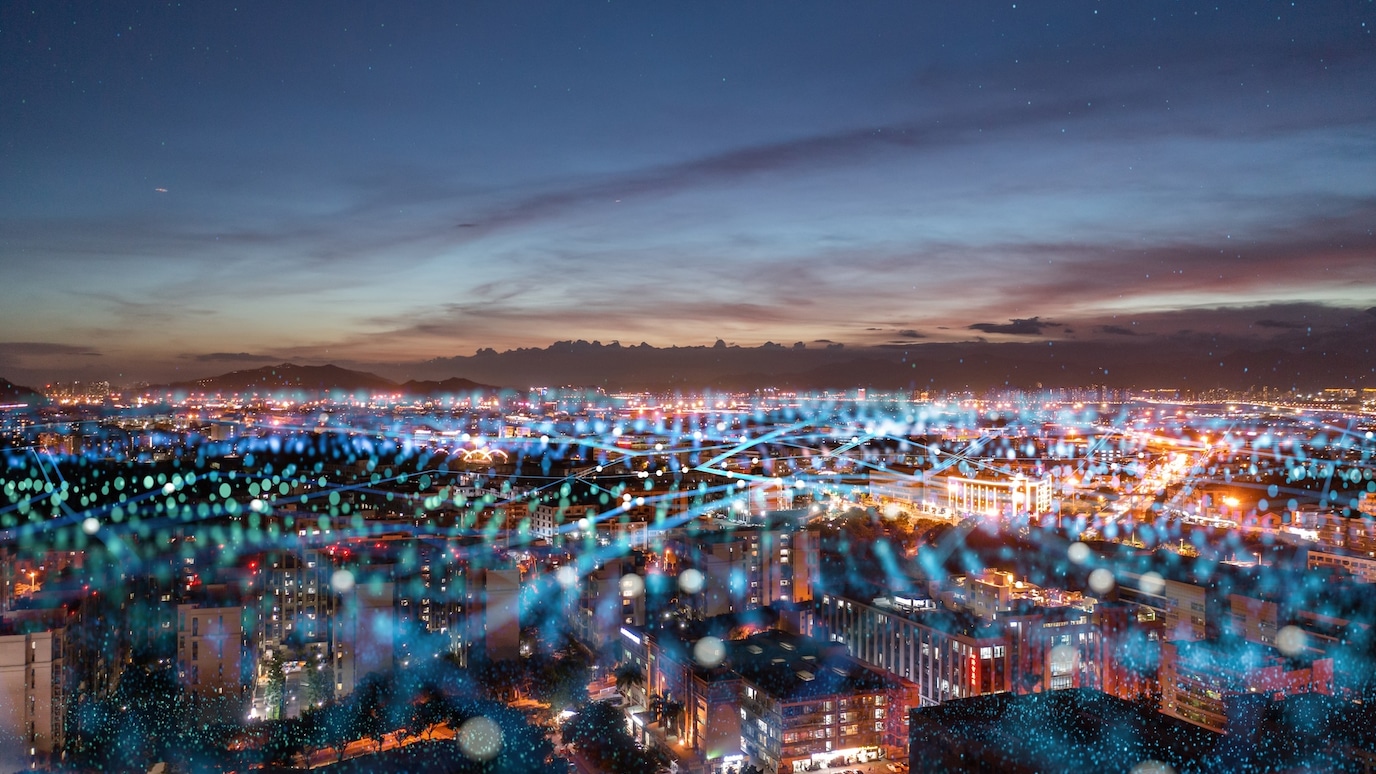
13 Aug 2024
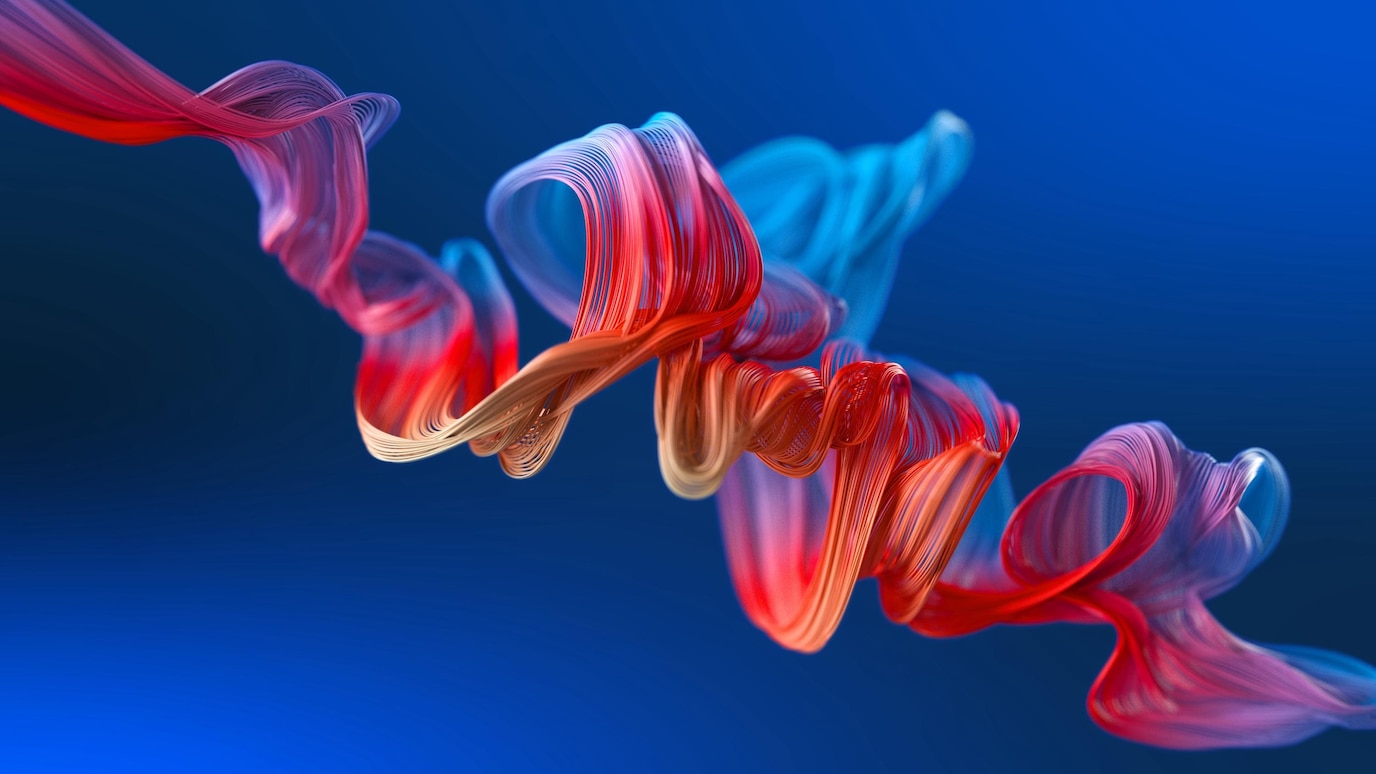
27 Feb 2025
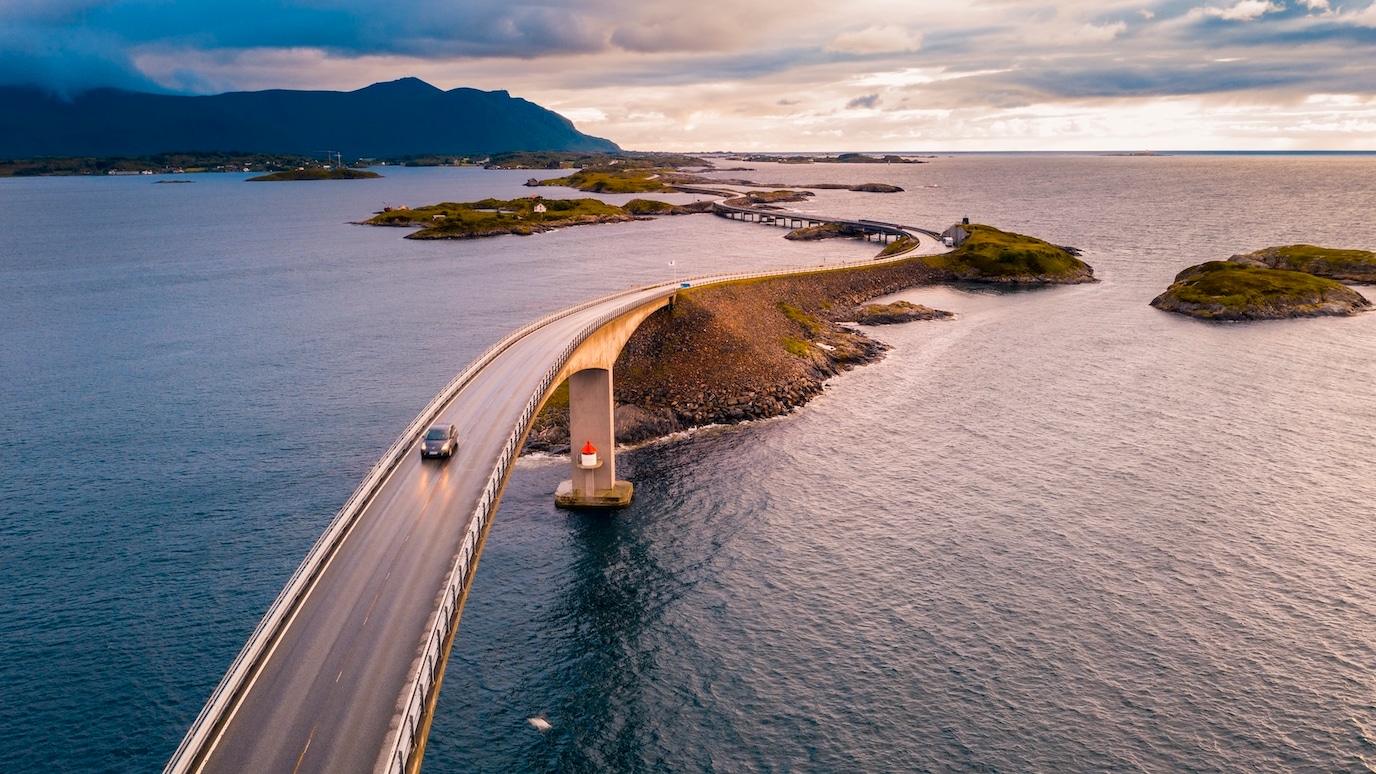
28 Nov 2024
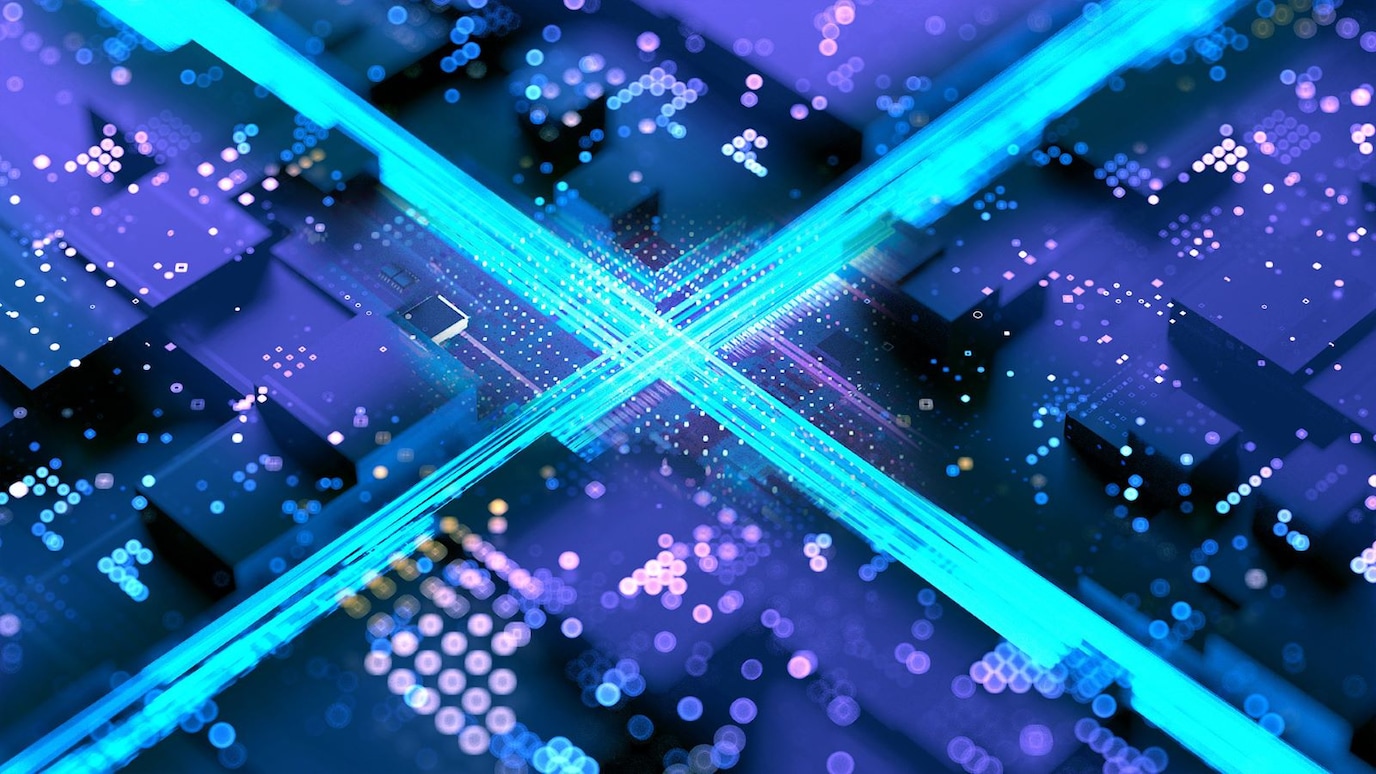
26 Nov 2024
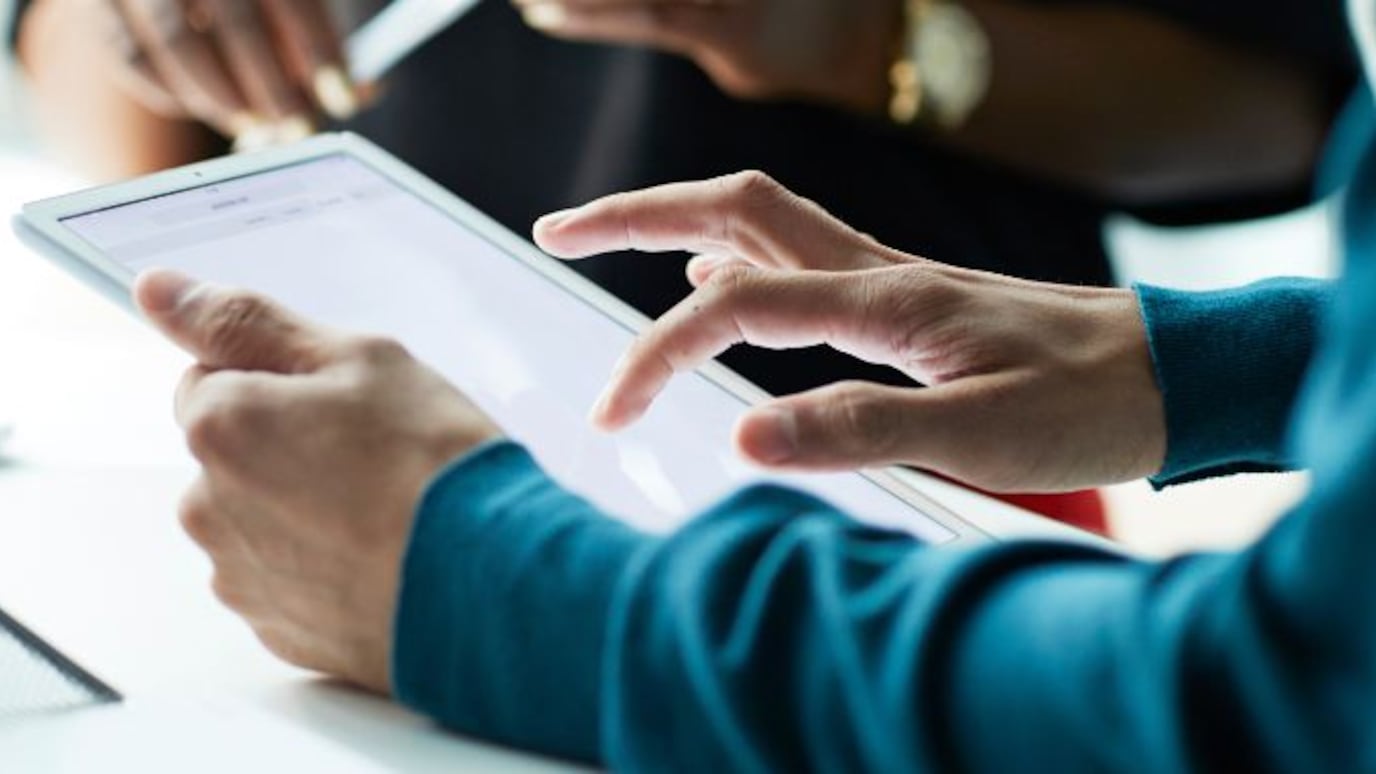
22 Nov 2024
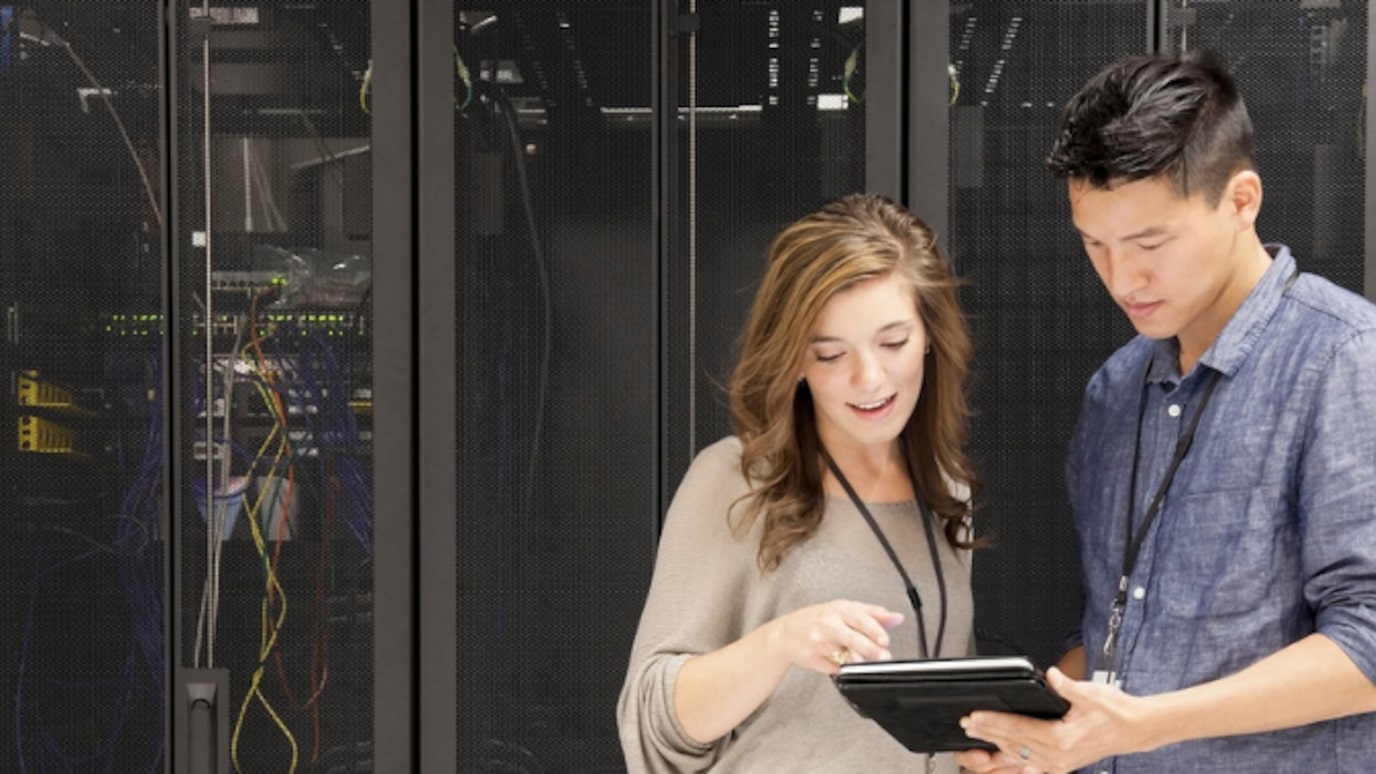
4 Oct 2024
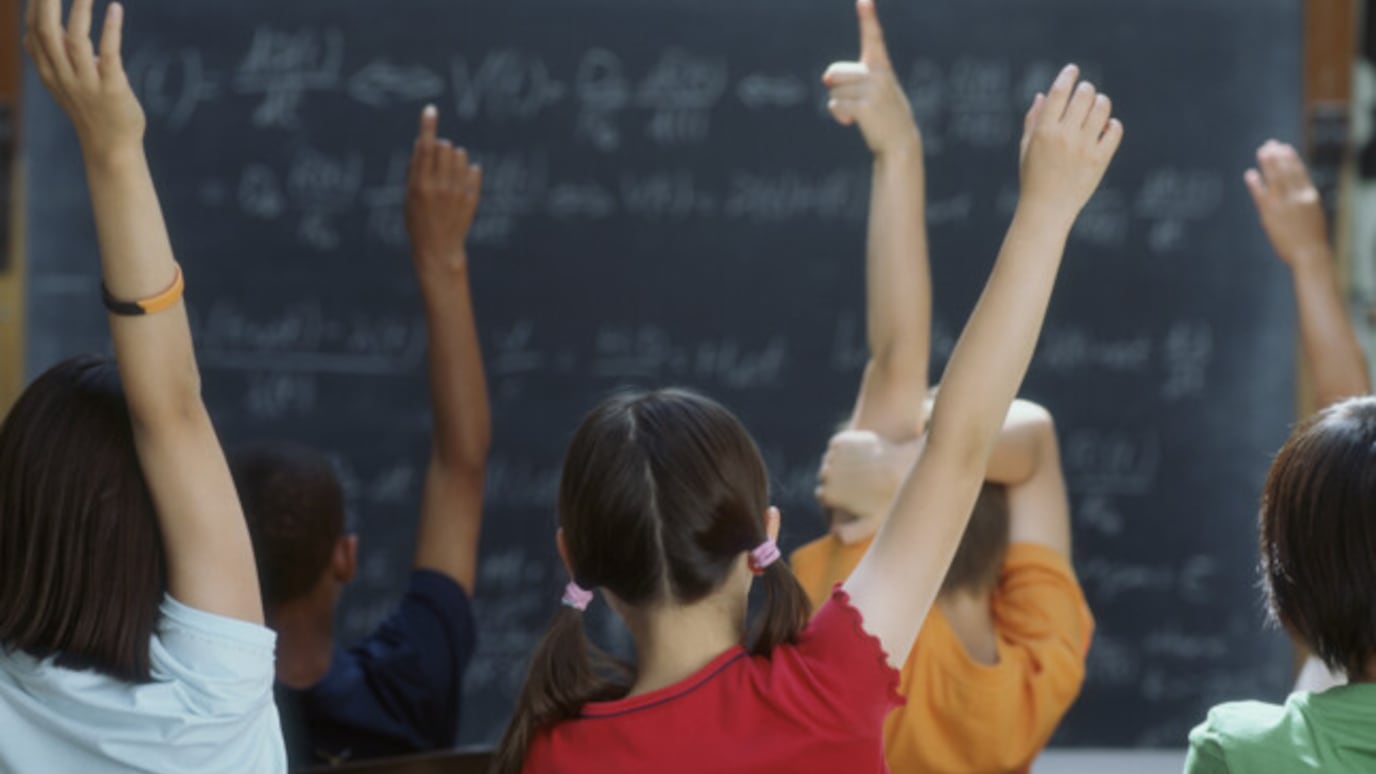
9 Jul 2024
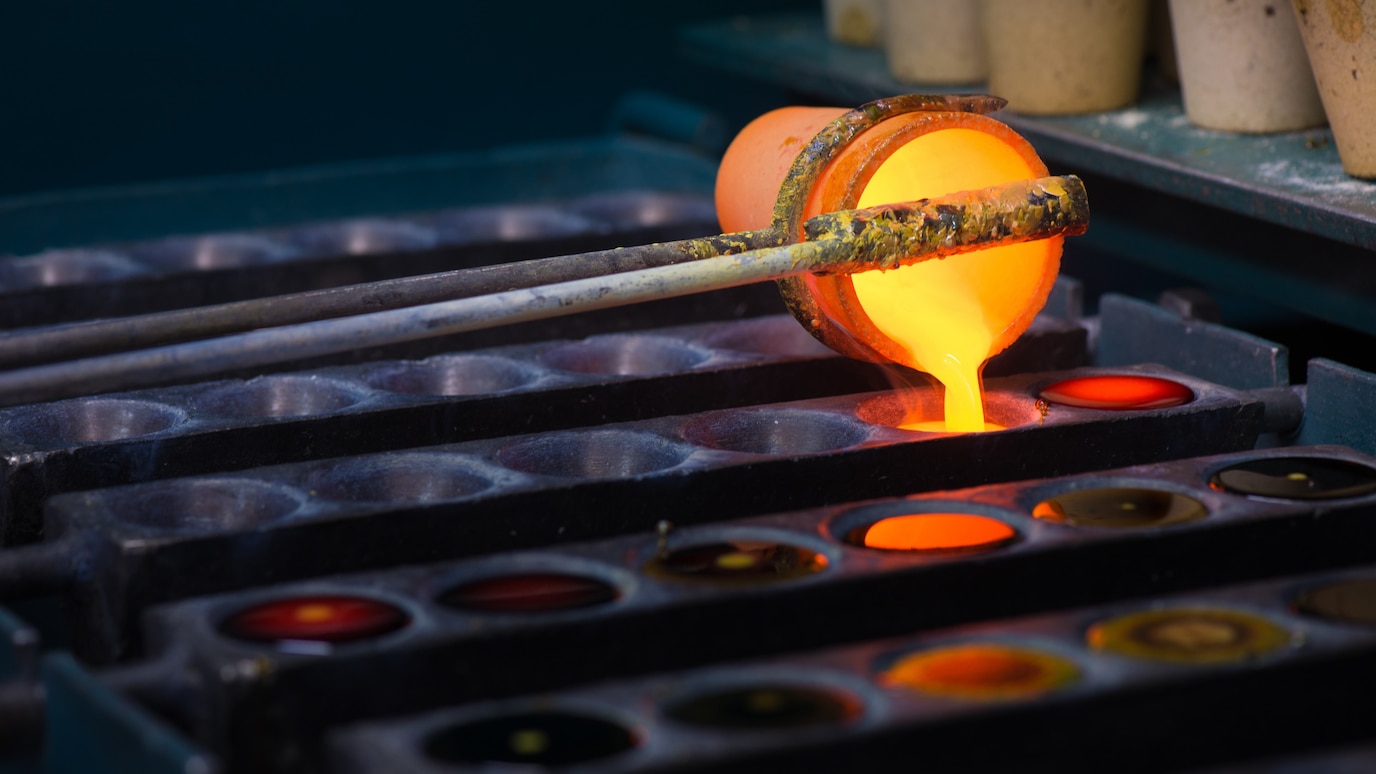
7 Jun 2024
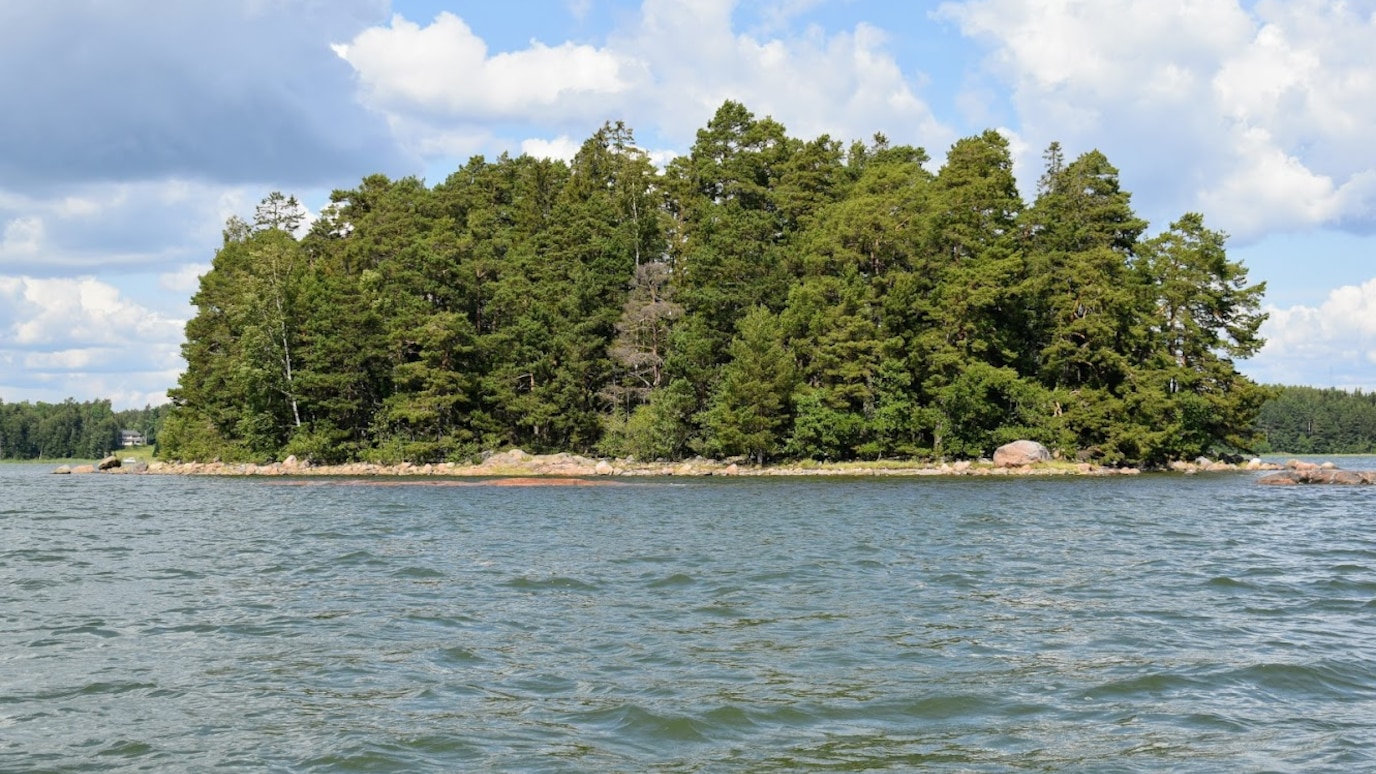
22 May 2024
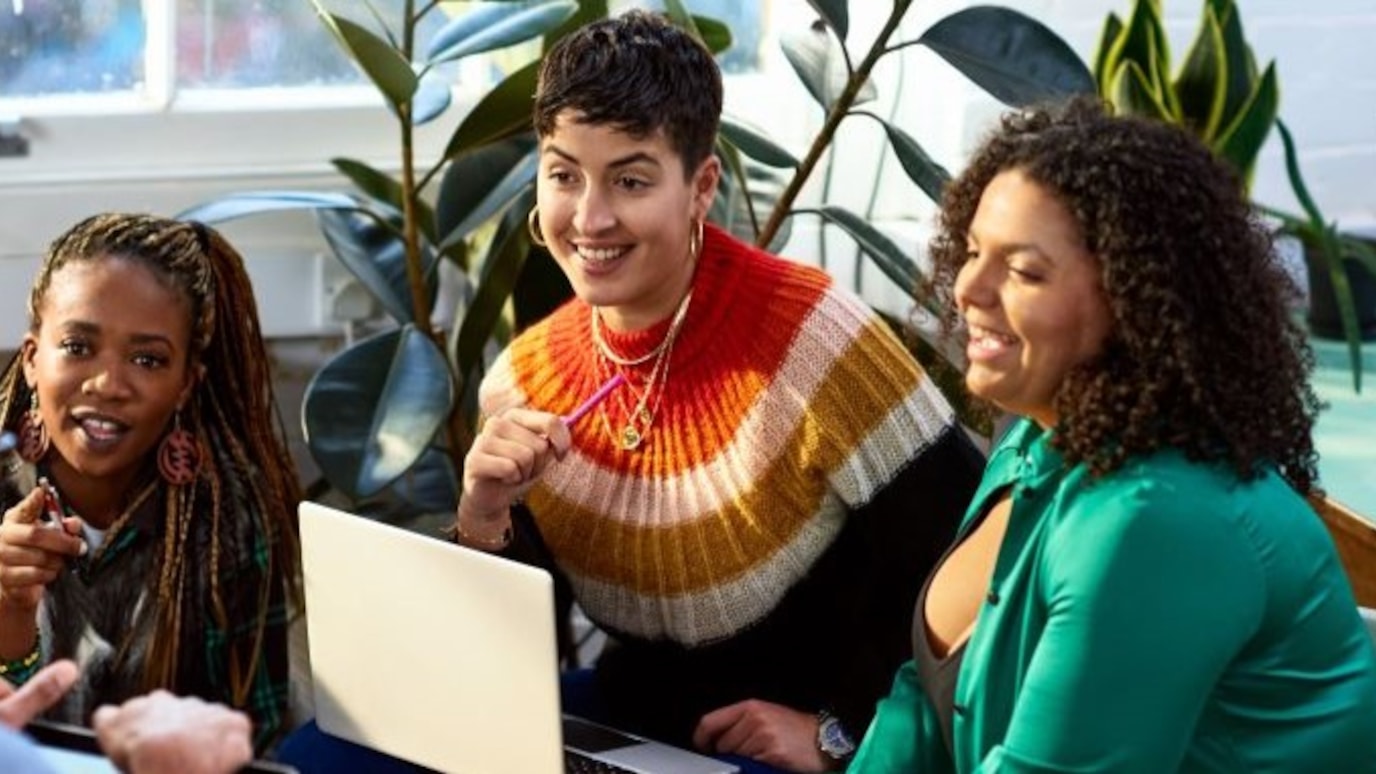
10 Oct 2023
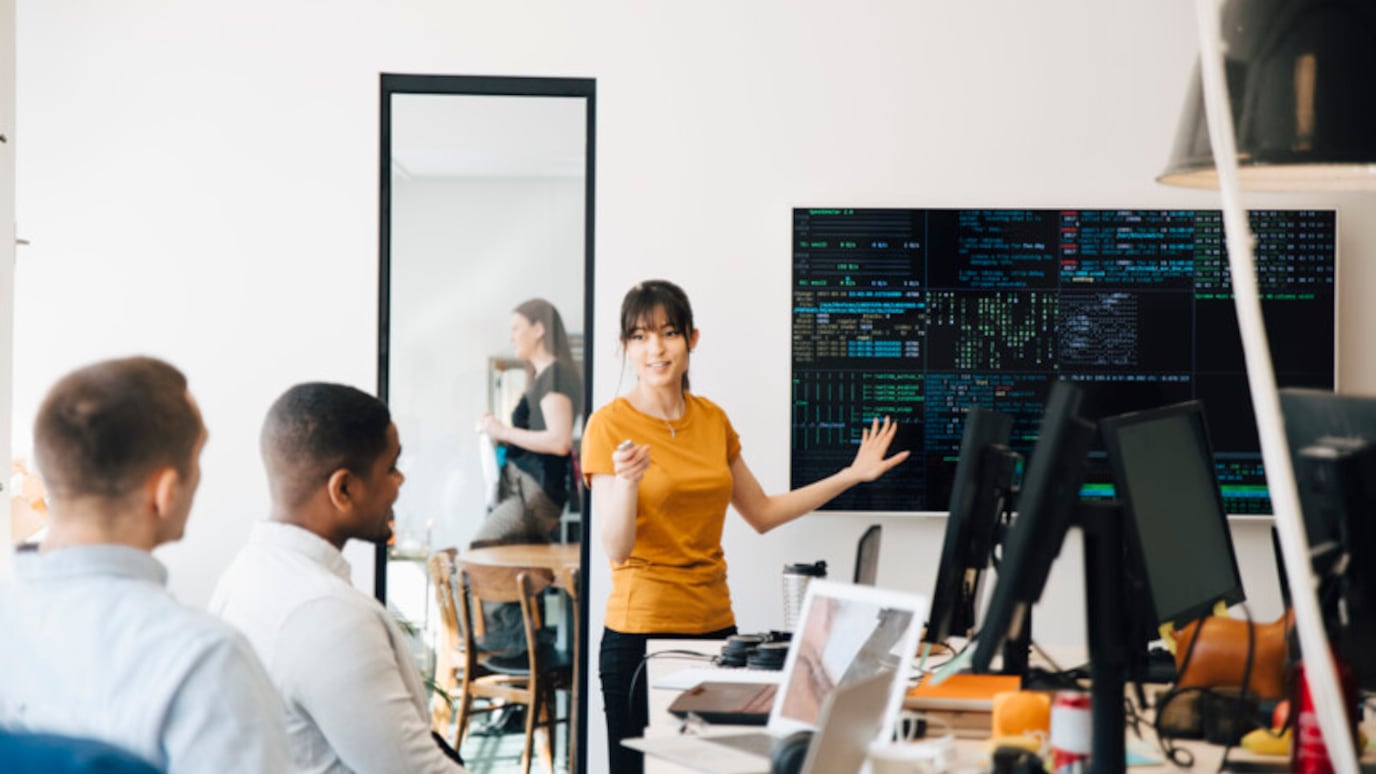
8 May 2023
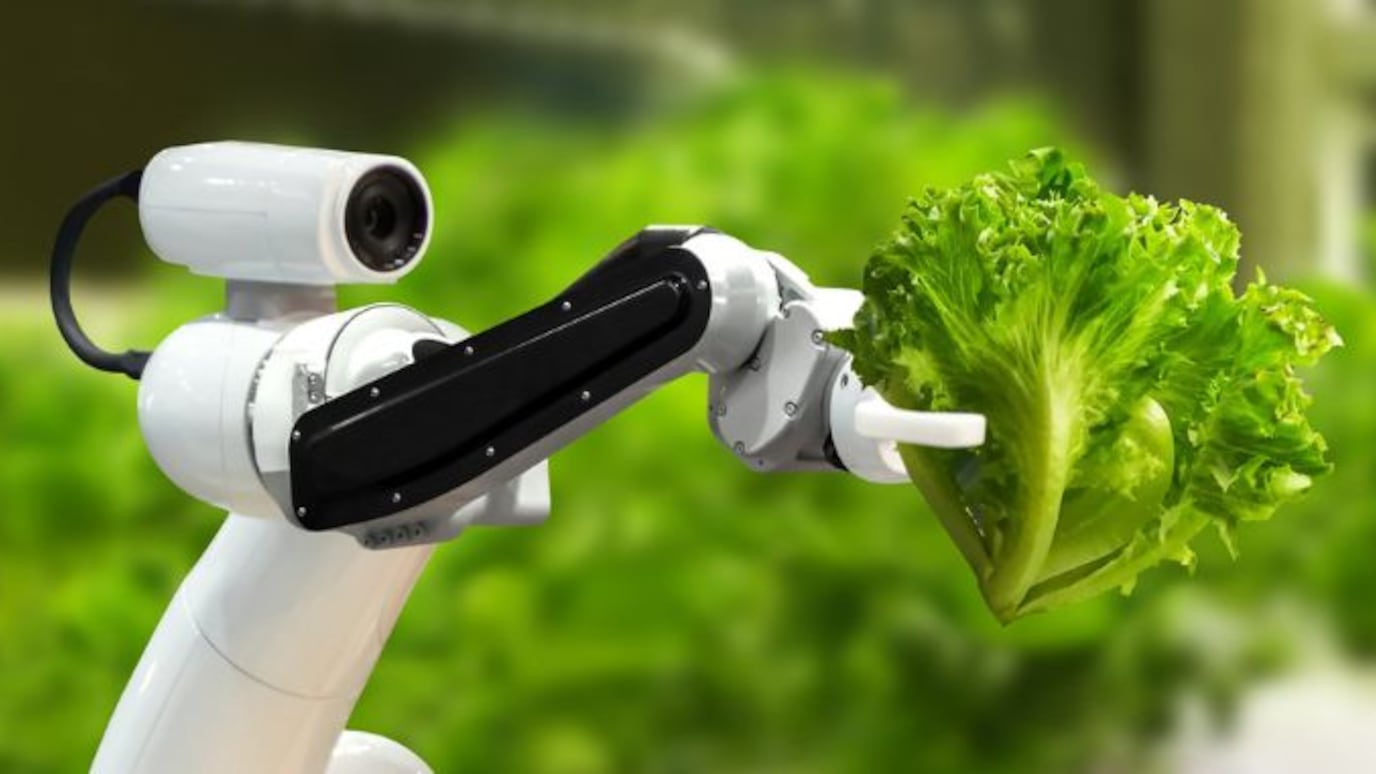
23 Mar 2023
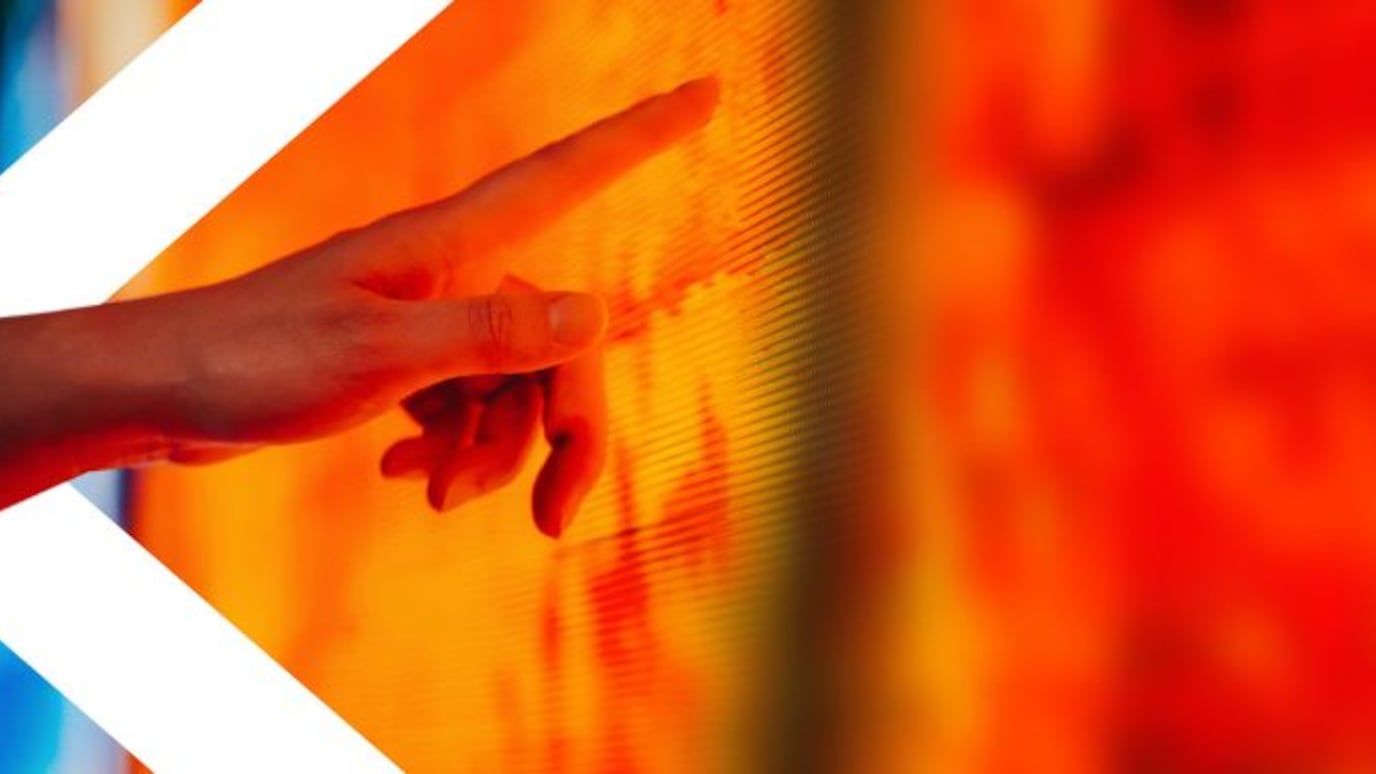
13 Mar 2023
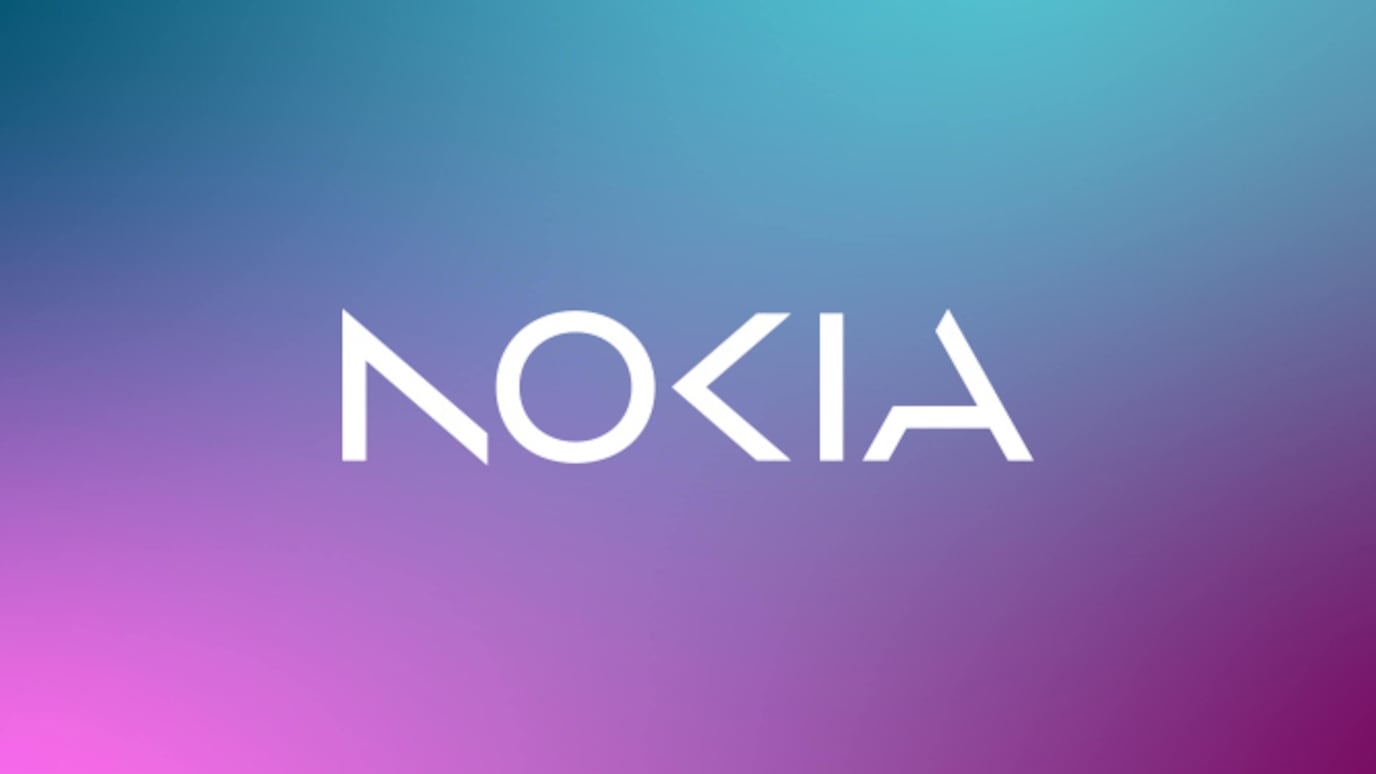
26 Feb 2023
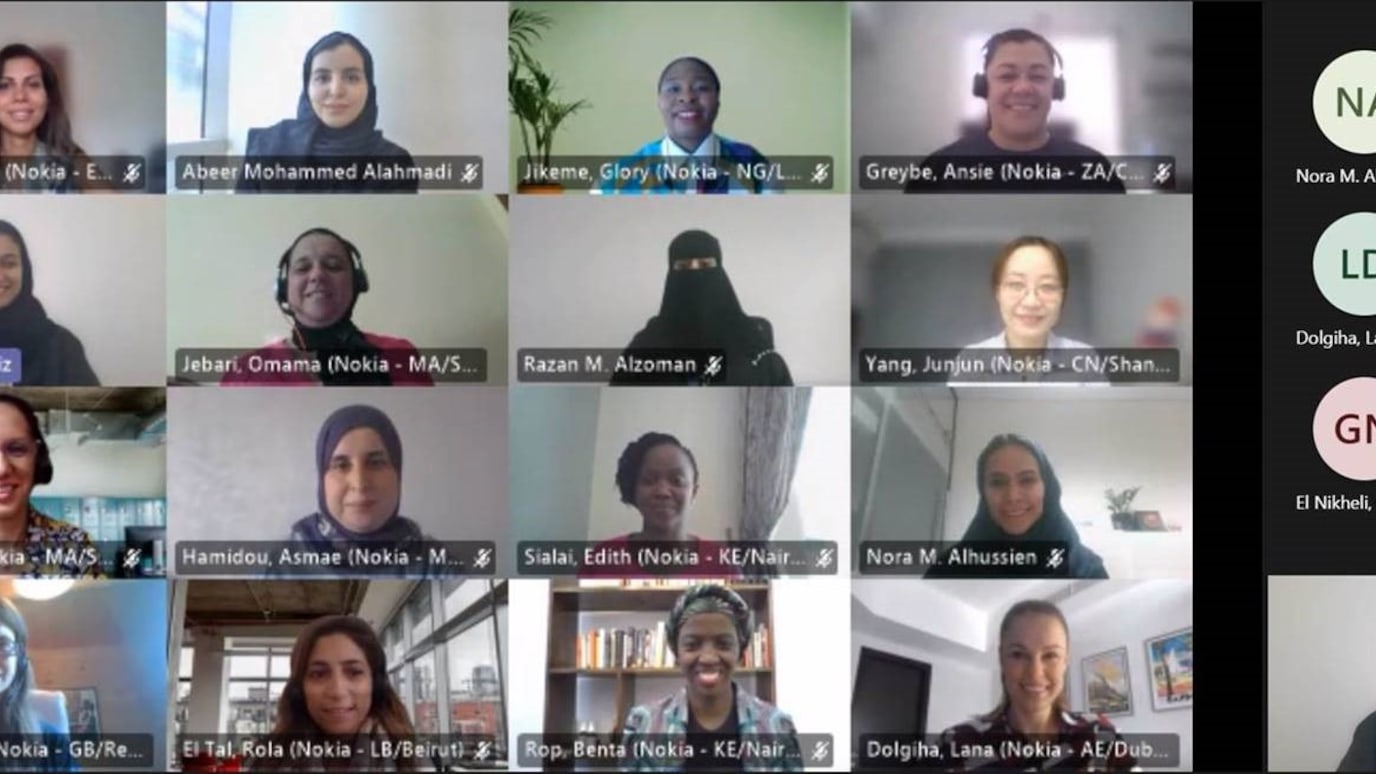
21 Dec 2021
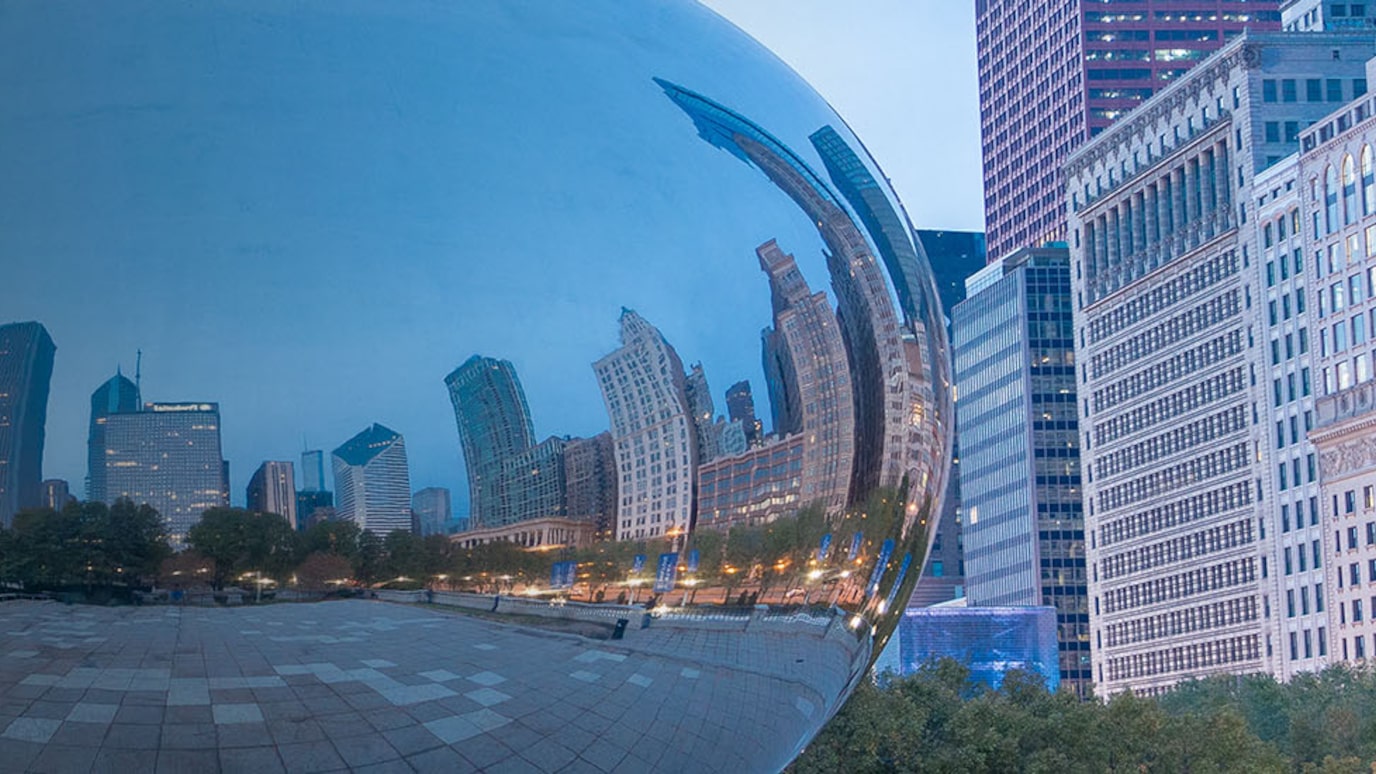
22 Oct 2021
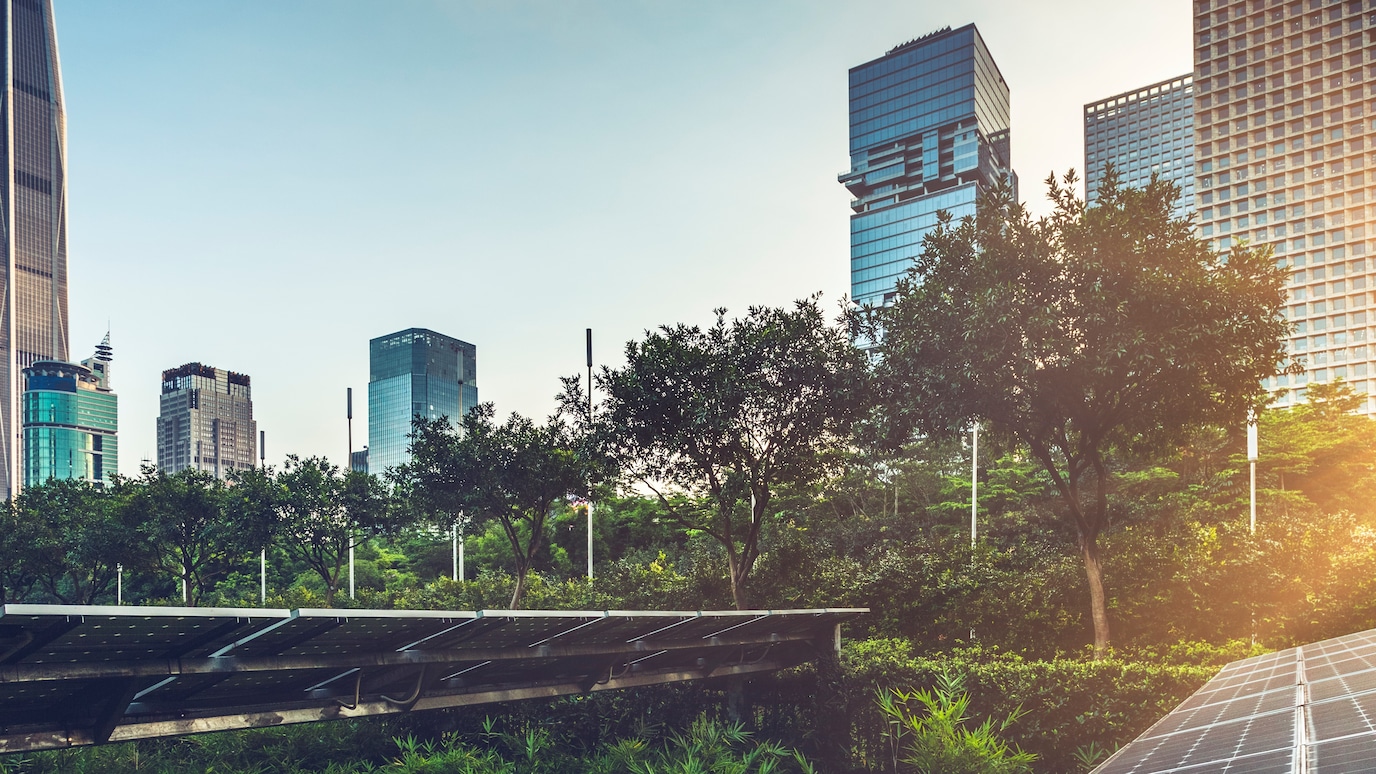
30 Sep 2021