Using AI and automation to protect optical networks from fiber cuts
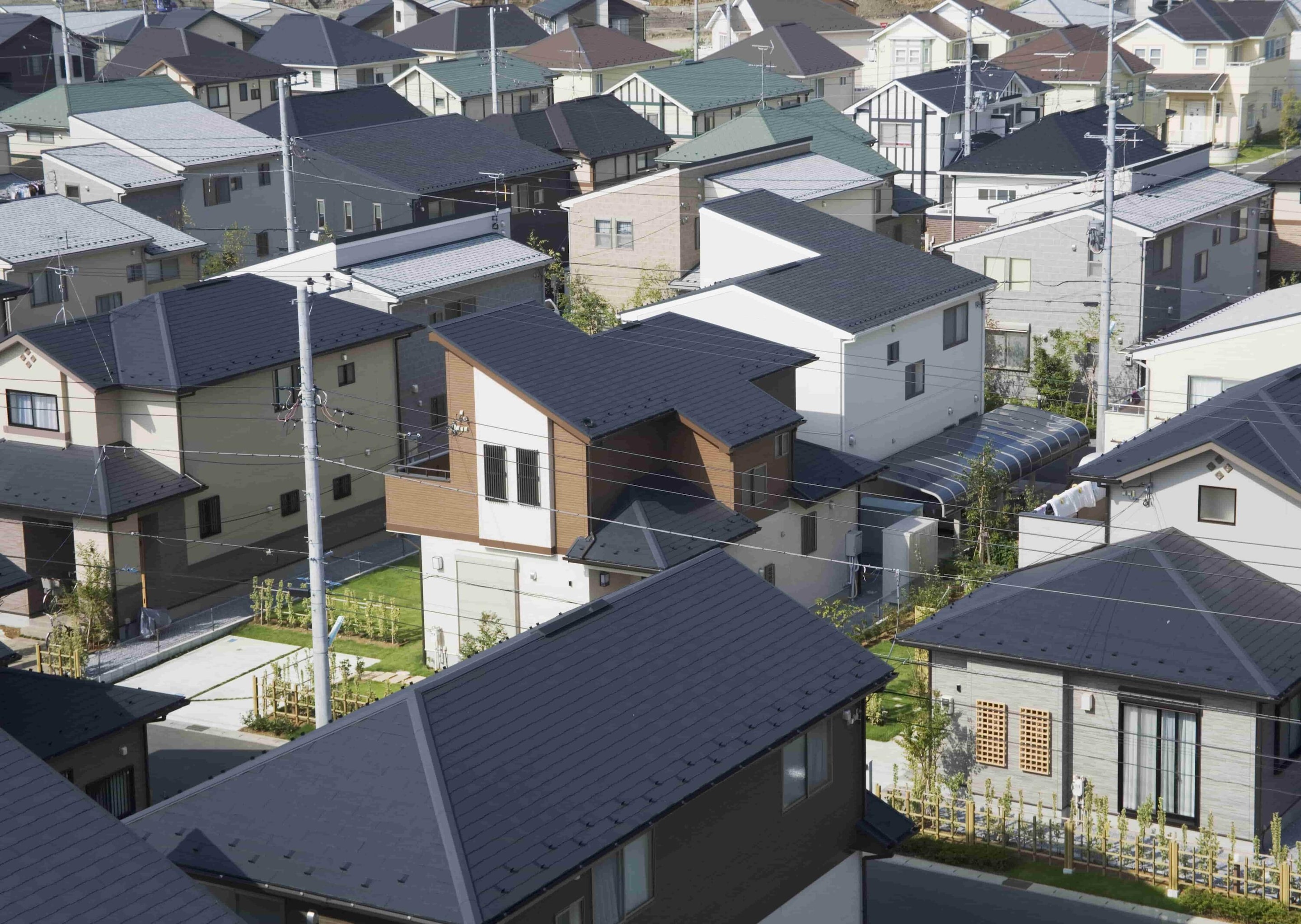
Artificial intelligence (AI) is gaining traction within the optical community as researchers investigate new ways to apply it to solve interesting problems. One of these applications is the proactive detection of fiber cuts.
Today’s solutions against fiber cuts
Fiber cuts are a large issue for terrestrial optical networks because most telecom fibers are buried underground, about 1 m deep. Dedicated road signs (see Figure 1) do a good job of helping to prevent cuts in rural areas. However, these signs aren’t always seen or adhered to in urban areas, so digging and drilling sometimes yield embarrassing surprises, especially when internet services are disrupted.
Figure 1. Warning sign for buried fiber optic cable
Most fiber cuts in urban areas are caused by civil or road works. But urban rodents contribute to the problem, too. Fortunately, work crews and cable-chewing animals leave optical signatures that we can detect. With help from AI, we can determine the nature and location of a given threat or cut and automate the triggering of restoration algorithms.
There are two current solutions for protecting against fiber cuts. The first is dedicated 1+1 protection, which puts 50 percent of network capacity in reserve and makes it possible to switch between fibers in less than 50 ms by duplicating signals across disjoint fiber routes from source to destination. The second is to use fast and intelligent algorithms to power network protection and restoration capabilities such those provided by the Nokia GMPLS wavelength routing solution. This approach computes back-up fiber routes to get around faulty fibers and reconfigures network resources to use higher-availability routes.
Protection and restoration can also work together to deliver maximum availability for critical wavelengths. With the addition of AI, we can proactively trigger restoration just before it’s needed, enabling very fast switching to alternate fiber routes and extremely efficient use of network resources.
Using the power of data to see into the future
AI opens the door to approaches that increase network availability through proactive failure detection and optimization of increasingly complex systems. It can also support new service definitions enabled by just-in-time restoration. To unleash this innovation potential, we need massive monitoring of the optical infrastructure. This becomes a tangible reality with advanced coherent technology, which offers a significant amount of data with no need to deploy extra hardware.
To help prevent fiber cuts, we use fiber as a sensor to detect nearby mechanical events, such an excavator that is beginning to dig. It will take a while for the excavator to reach a depth of 1 m, so we believe we can identify a dangerous situation before the fiber is cut.
Modern optical variable bit rate transponders transmit information on the two orthogonal polarizations of the light. Their receivers track any fluctuation of these two polarizations to decode the transmitted information correctly. It is also possible for the receiver to monitor these fluctuations by reconstructing and tracking the so-called state-of-polarization (SOP) thanks to existing filters used in advanced digital signal processing. The SOP is made up of three parameters, denoted as S1, S2 and S3. It can be represented geometrically using the Poincaré sphere, as shown in this video of the proof of concept we developed.
Identifying and classifying event signatures
AI-enabled machines have a unique ability to learn patterns. For example, they can be trained to classify and distinguish between people’s signatures based on handcrafted marks. In signatures, features that could be classified may include the number of letters, the presence of an underline and the space between two letters.
We can use these capabilities in a similar way to help prevent fiber cuts. We start by collecting thousands of SOP measurements to populate the database required for AI. Each of these SOP measurements has a duration of about two seconds and is like a signature that can permit us to identify a specific type of event.
Event classification with SOP measurements is determined by a set of easily interpretable features, such as the minimum amplitude value required for a given period of the measurement. In our lab, we used a robot arm to play with the fiber to generate four different reproducible events, which we referred to as “bending,” “shaking,” “small hit” and “up and down.” The figure below shows examples of the SOP evolution for these events.
We would like to be able to detect any risky event that could lead to a fiber cut with a given probability. Our proposal is to classify events as “high risk,” “medium risk” or “low risk” based on their threat to fibers and define appropriate actions for each of the classes.
Triggering rapid restoration
When a real-time network health and analytics application detects, classifies and correlates risky events from different lightpaths (i.e., wavelengths), it can localize the fiber span where the damage is about to occur and send a notification to an SDN controller and/or GMPLS implementation to take the appropriate actions.
The SDN controller and/or GMPLS implementation are in charge of calculating the new service restoration routes. They configure the restoration paths while the working paths are still operational. When the fiber breaks and a loss of light is detected, they can rapidly switch network traffic from the working paths to the restoration paths. Proactive detection of fiber cuts allows us to minimize the impact to services by using back-up resources only when needed, reducing network total cost of ownership (TCO).
Helping optical network operators sleep better at night
This blog post describes just one example of the promising applications of AI in optical communication that will be enabled by integrating our deep expertise in physical layer insights and analysis of real network telemetry into easy-to-use Nokia WaveSuite Network Insight tools. Soon, optical network operators will sleep better at night knowing that a new application of AI will recognize the signatures of fiber cuts associated with civil works or hungry, enterprising rodents that choose to ignore our warning signs.
Learn more from a product perspective below.
Application notes:
Nokia Insight-driven optical networks
Nokia WaveSuite Network Insight
Datasheet
WaveSuite Network Insight – Health and Analytics
Web page:
Nokia Insight-driven optical networks
Further technical reading
- F. Boitier, J. Pesic, V. Lemaire, E. Dutisseuil, J.-M. Estaran, P. Jennevé, N. Le Moing, H. Mardoyan, P. Layec, “Seamless Optical Path Restoration with Just-in-Time Resource Allocation Leveraging Machine Learning,” in Proc. ECOC, 2018
- F. Boitier, V. Lemaire, J. Pesic, L. Chavarría, P. Layec, S. Bigo, E. Dutisseuil, "Proactive Fiber Damage Detection in Real-time Coherent Receiver", in Proc. ECOC, 2017