Moneybaud: Using data and automation to get the most from optical networks
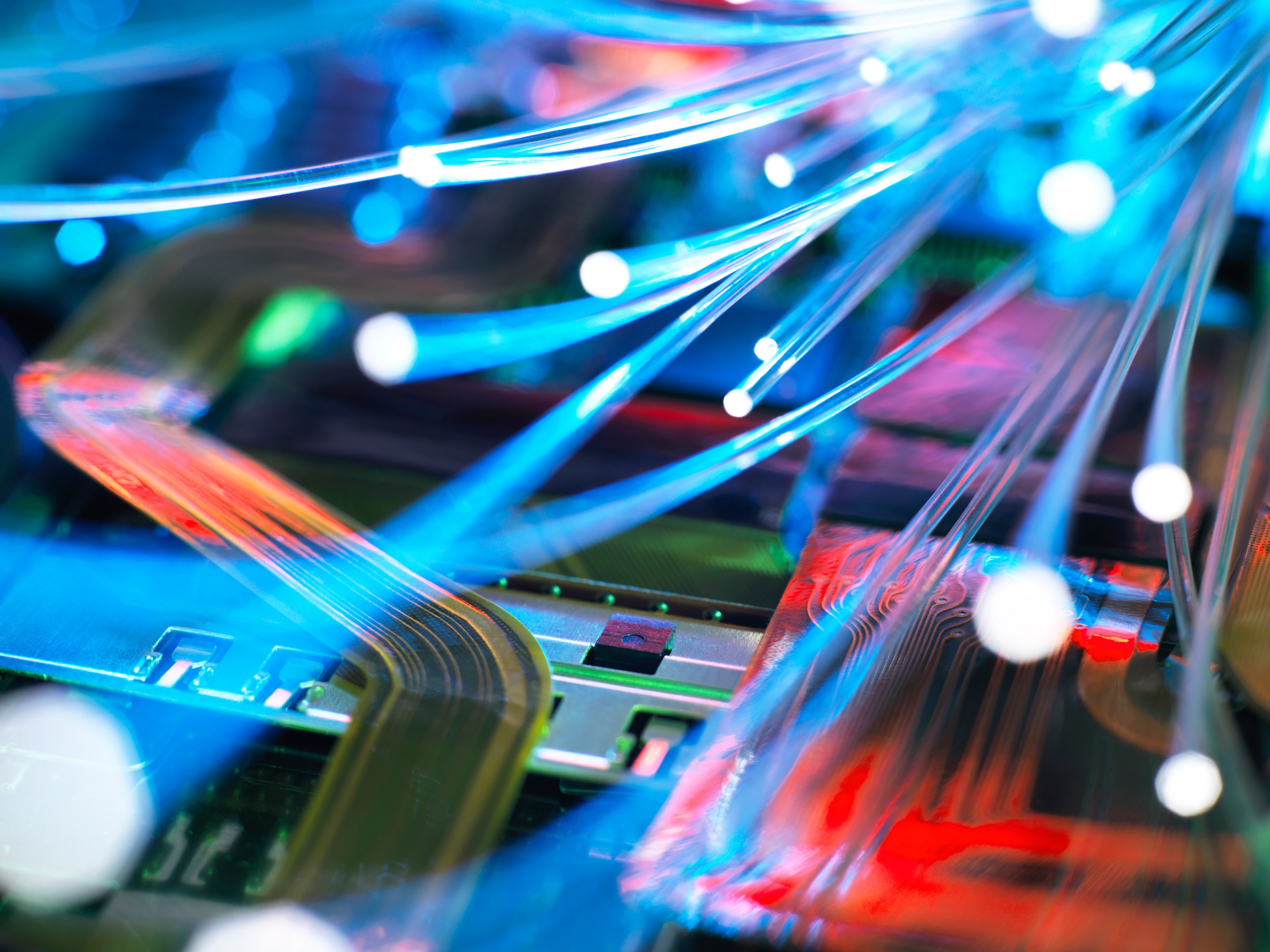
As the world becomes more dependent on optical networks, their design and operation are increasingly focused on reliability. Network reliability is usually measured in terms of availability – in other words, the uptime percentage. Operators and their customers generally expect five-nines availability from the optical network, meaning that any demand should be available 99.999 percent of the time. This represents an average of 5 minutes of downtime per year, a little less than one second per day. Needless to say, this does not come easy or cheap.
Let’s say I want to design a network with five-nines availability and a 15-year lifetime. Many things can (and will) happen in 15 years, including fiber cuts and repairs, power outages, equipment aging or failing, fires and orange-toothed critters. You name it. What’s more, unless I’m willing to fully characterize the performance of all network elements prior to field deployment, I can’t accurately predict what the quality of transmission (QoT) – for example, the OSNR or Q2 – will be for any of my connections at Day 1. If I underestimate QoT impairments, there is a high risk that there will be too many transmission errors. That means the connection won’t work, so goodbye five-nines, hello angry customer!
Because of Day 1 QoT uncertainty and the network’s long lifetime, I have to drastically overestimate potential QoT impairments at the design stage. In network designer parlance, I have to add margins. The challenge is that I only have a five-minute downtime budget per year, and two possible types of failures: hard failures and soft failures.
A hard failure occurs, for instance, when a falling tree severs an aerial fiber cable. Not to point the finger, but beavers are known to do this. Because all signal-carrying photons are lost at the cut, this generates downtime no matter how much QoT margin there was initially, unless a redundant lightpath is ready to take over.
In contrast, a soft failure such as a fiber bend results in a limited decrease of QoT. If the QoT falls below the forward error correction threshold, I have downtime. If not, I’m OK. The more QoT margin I have, the less likely it is that a soft failure will result in downtime.
Since the impact of soft failures is the only thing I can effectively mitigate through margins, this is typically where I don’t want to take any chances. So I’m going to build a worst-case scenario to estimate the lowest possible QoT I will have during the 15 years, and design my network based on it. I call this Murphy’s law design because I assume that everything that can go wrong eventually will go wrong – except beavers, who do not give a dam about margins.
I account for beavers (and other creatures) in a later, independent stage of design when I evaluate the need for optical protection. With the worst-case approach for QoT and optical protection to deal with disastrous events such as fiber cuts, I can deliver five-nines and everything is fine. Right?
Well, it used to be fine. But as Greek philosopher Heraclitus famously stated, “Change is the only constant in life.” So what changed? What makes us think we can do better now? Three things: observability, analytics and elasticity.
I remember my arrival at Bell Labs about six years ago. Coming from an experimental physics background, I was immediately curious about what was actually going on in the field, where we can’t control things as much as we do in simulations or in a physics lab. For instance, early in my career, taking biosensors out of the lab after years of careful development to do biology turned out to be extremely challenging. This seared in my brain the idea that the lab only takes you this far. Truth is in the field. The monitoring capabilities of coherent transponders based on the Nokia PSE digital signal processors (DSPs) further aroused my curiosity. Despite these capabilities, large-scale data collection was seen as impractical at that time.
Things did change. Why? Because of machine learning. The way the tech giants leveraged enormous quantities of data to improve many aspects of their businesses made our industry realize that our data could have tremendous value. Pioneering research at Bell Labs put us in the starting block to unlock this value.
Leveraging data will change many aspects of optical network design and operation. For instance, remember that margins are dimensioned for extremely unlikely worst cases. Once you can observe the field, you have a much clearer view of what to expect if you were to reduce margins. But what can you do with extra margins once the network is already deployed? Sadly, you won’t get your CAPEX back. But you can get the best from your investment. This is where elasticity and smart analytics kick in.
Let’s say that your network is initially designed for 100 Gb/s demands. With tools such as Nokia WaveSuite Health and Analytics, which deliver network-wide observability, analytics and triggers for automation, you can evaluate which demands could support more capacity – 150 Gb/s, 200 Gb/s or more. Transponder elasticity then allows you to implement such capacity upgrades. With probabilistic constellation shaping (PCS) implemented in the latest Nokia products, you get even finer rate adaptations. The best thing is that in most cases, you can make these upgrades without consuming optical spectrum (a precious network resource).
The idea is to get the most out of every transmitted symbol. If you know “Moneyball,” the story of how the general manager of a Major League Baseball team used data science to achieve the longest winning streak in history with one-third of the budget of the richest team, you will probably understand why I call this “Moneybaud.”
Elasticity allows you to convert margins into more capacity. Observability and analytics help you decide whether or not this is a smart bet. This is part of the now famous observe-decide-act loop introduced in 2014 in the ORCHESTRA project, which has since been “borrowed” by many other vendors.
Everyone understands that transporting more capacity with the same spectral bandwidth essentially means generating more revenue from the same infrastructure. You can use the extra capacity, which is obtained almost for free, or sell it as a service to a third party. Converting margins into more capacity is thus financially appealing. But this conflicts with the idea that margins are intrinsically linked to availability. We actually proved this with real network data. So reducing margins means reduced availability and potentially less revenue because it also means lower quality of service and more complex network operation. That’s the losing side of the bet. So how do you beat the odds and get past this conundrum?
Statistical design
Worst-case design is about assuming the worst performance that you can think of for all network elements. This approach ensures you there will be sufficient margins once you deploy your network. However, it does not provide any visibility of the margins you are likely to have after deployment.
In statistical design, you treat the performance of different network elements such as amplifiers as random variables with specific distributions. Much like banks let you choose a risk profile to place your savings, statistical design allows you to tailor margins and thus network cost to your Day 1 availability requirements. You can do this even more efficiently for network upgrades thanks to the improved knowledge you got from observing your network from Day 1.
Check out this blog post and paper for more on statistical design.
Optimizing quality of transmission (QoT)
Now, what if you could go from passive to active and increase your QoT for free post Day 1, after deployment? With the same capacity, more QoT means more margins and thus more availability. More QoT also allows you to increase capacity while maintaining the same availability. It’s a win-win situation. Does it sound too good to be true? Well, it’s not. You can do it in many ways, for instance, by optimizing the launch power or fine-tuning channel frequencies to minimize filtering penalties.
Check out this paper and blog post to find out more about optimizing QoT.
Optimizing availability
Events such as fiber cuts, power failures and equipment malfunctions will always make it tougher to achieve high availability. These types of failures can’t be avoided in optical networks. However, we can better anticipate some of them by leveraging machine learning to support proactive real-time identification. We can also react to them more effectively with a solution such as the Nokia wavelength routing solution, which enables rapid protection and restoration. This can reduce outage time thus providing a drastic increase of the effective availability.
For more information, check out this blog post on using AI and automation to protect optical networks from fiber cuts.
Quantifying the capacity–availability trade-off
Converting margins into capacity might seem reckless, simply because everyone intuitively understands that this may impact quality of service by reducing availability. Now, what if you could accurately quantify how trading margins for capacity would impact availability? This is precisely what we propose in this paper. I’ll summarize the key points below.
Let’s consider the publicly available monitoring data of a large North American backbone network. The QoT of 4,000 demands that each deliver 100 Gb/s with PDM-QPSK format has been recorded over a 14-month period. We start by quantifying a posteriori the capacity-availability trade-off for a single representative demand. The results are illustrated in the figure below.
Monitored SNR from a 100Gb/s demand and error-free thresholds for selected bitrates.
The figure plots the monitored SNR samples as black circles and the error-free SNR thresholds as solid lines. For all considered bitrates, these SNR thresholds represent the minimum SNR values required to guarantee error-free transmission. Now, let’s assume the initial bitrate was 200 Gb/s instead of 100 Gb/s. What would be the cost in terms of average availability?
Answering this is easy with the monitored SNR. We just need to count the corresponding unavailable time – that is, the amount of time that the monitored SNR was below the 200 Gb/s threshold. If we do this for all bitrates we can think of, we get the figure below.
Availability versus bitrate for the selected demand calculated from monitored QoT and error-free thresholds
This figure quantifies the capacity-availability trade-off for our demand. For simplicity, we do not account for other sources of unavailability, such as beavers. The large dots correspond to selected bitrates with 25 Gb/s granularity, while the solid line represents a continuum of intermediary bitrates that could theoretically be achieved with techniques such as PCS.
In our previous example, doubling the bitrate and thus the capacity would have led to an availability close to 99 percent. However, upgrading to 175 Gb/s would have secured an availability above 99.99 percent. For comparison, going from 99 to 99.99 percent is precisely what you would get with 1+1 protection – roughly twice the infrastructure for the same demand!
Small capacity adjustments can have huge benefits in terms of availability, and thus quality of service. With observability and elasticity, it’s all about choosing the bitrate setting that will maximize your gains while controlling the risks. This is the essence of “Moneybaud.”
What happens if we consider the whole network rather than a single demand? The figure below shows the result of a network-wide study. The bottom right part of the figure indicates the network throughput achieved with the initial 100 Gb/s rate setting. Here, the throughput accounts for all downtimes. The optimal throughput is reached when bitrates are set network wide so that availability remains above 99.9 percent for all demands. When the availability target is too low, bitrates are too high, leading to frequent outages. In other words, there are not enough margins. This is clearly not where you want to be.
Projected network throughput as function of the targeted availability
On the right side of the optimum (indicated by the dotted line in the figure), throughput is just below optimal and availability remains high. In other words, data analysis allows us to identify a margin sweet spot where you get the most capacity out of your network with negligible impact on quality of service (i.e. the best network performance).
Naturally, we seek to go from a theoretical network capacity gain – as presented above – to an actual gain. This means moving from a posteriori analysis to automated, real-time rate adaptation, and it presents a real challenge. No matter how hard we try, we cannot fully predict the future. The best we can do is to prepare for it. How? The good thing with data is that we can run as many automated rate-adaptation scenarios as we want before deployment. If you tell us how safe you want to play, we’ll find the best automation to succeed. Of course, the more you take risks, the more you can gain. Fair enough?
You can further reduce risk levels and improve your odds by using multiple algorithm approaches to monitor and cross-check performance assumptions, such as the approaches described in the previous posts in this blog series. You can also reduce risk by better predicting the future, as described in this post on using AI and automation to protect optical networks from fiber cuts. This is the next best thing to a crystal ball, especially when you integrate these approaches with easy-to-use Nokia WaveSuite Network Insight application tools.
Learn more from a product perspective below.
Application notes:
Nokia Insight-driven optical networks
Nokia WaveSuite Network Insight